Blueprint Separable Subsampling and Aggregate Feature Conformer-Based End-to-End Neural Diarization
ELECTRONICS(2023)
摘要
At present, a prevalent approach to speaker diarization is clustering based on speaker embeddings. However, this method encounters two primary issues. Firstly, it cannot directly minimize the diarization error during the training process; secondly, the majority of clustering-based methods struggle to handle speaker overlap in audio. A viable approach for addressing these issues involves adopting end-to-end speaker diarization (EEND). Nevertheless, training this EEND system generally requires lengthy audio inputs, which must be downsampled to allow efficient model processing. In this study, we develop a novel downsampling layer using blueprint separable convolution (BSConv) instead of depthwise separable convolution (DSC) as the foundational convolutional unit, which effectively preserves information from the original audio. Furthermore, we incorporate multi-scale feature aggregation (MFA) into the encoder structure to combine the features extracted by each conformer block to the output layer, consequently enhancing the expressiveness of the model's feature extraction. Lastly, we employ the conformer as the backbone network to incorporate the proposed enhancements, resulting in an EEND system named BSAC-EEND. We assess our suggested methodology on both simulated and real datasets. The experiment indicates that our proposed EEND system reduces diarization error rate (DER) by an average of 17.3% for two-speaker datasets and 12.8% for three-speaker datasets compared to the baseline.
更多查看译文
关键词
feature,conformer-based,end-to-end
AI 理解论文
溯源树
样例
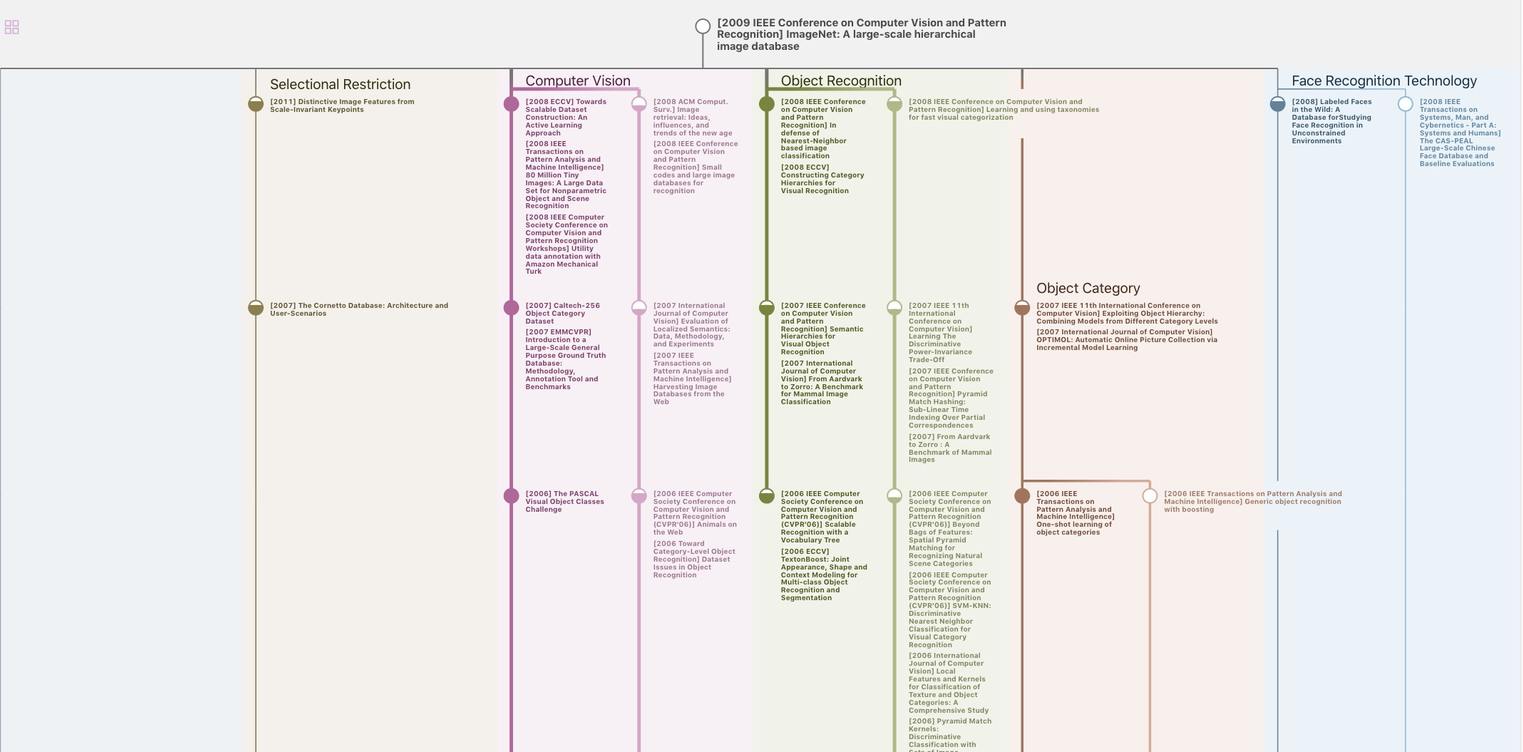
生成溯源树,研究论文发展脉络
Chat Paper
正在生成论文摘要