A Dynamic Ensemble Selection of Deepfake Detectors Specialized for Individual Face Parts
ELECTRONICS(2023)
摘要
The development of deepfake technology, based on deep learning, has made it easier to create images of fake human faces that are indistinguishable from the real thing. Many deepfake methods and programs are publicly available and can be used maliciously, for example, by creating fake social media accounts with images of non-existent human faces. To prevent the misuse of such fake images, several deepfake detection methods have been proposed as a countermeasure and have proven capable of detecting deepfakes with high accuracy when the target deepfake model has been identified. However, the existing approaches are not robust to partial editing and/or occlusion caused by masks, glasses, or manual editing, all of which can lead to an unacceptable drop in accuracy. In this paper, we propose a novel deepfake detection approach based on a dynamic configuration of an ensemble model that consists of deepfake detectors. These deepfake detectors are based on convolutional neural networks (CNNs) and are specialized to detect deepfakes by focusing on individual parts of the face. We demonstrate that a dynamic selection of face parts and an ensemble of selected CNN models is effective at realizing highly accurate deepfake detection even from partly edited and occluded images.
更多查看译文
关键词
deepfake, deep learning, ensemble learning, object detection
AI 理解论文
溯源树
样例
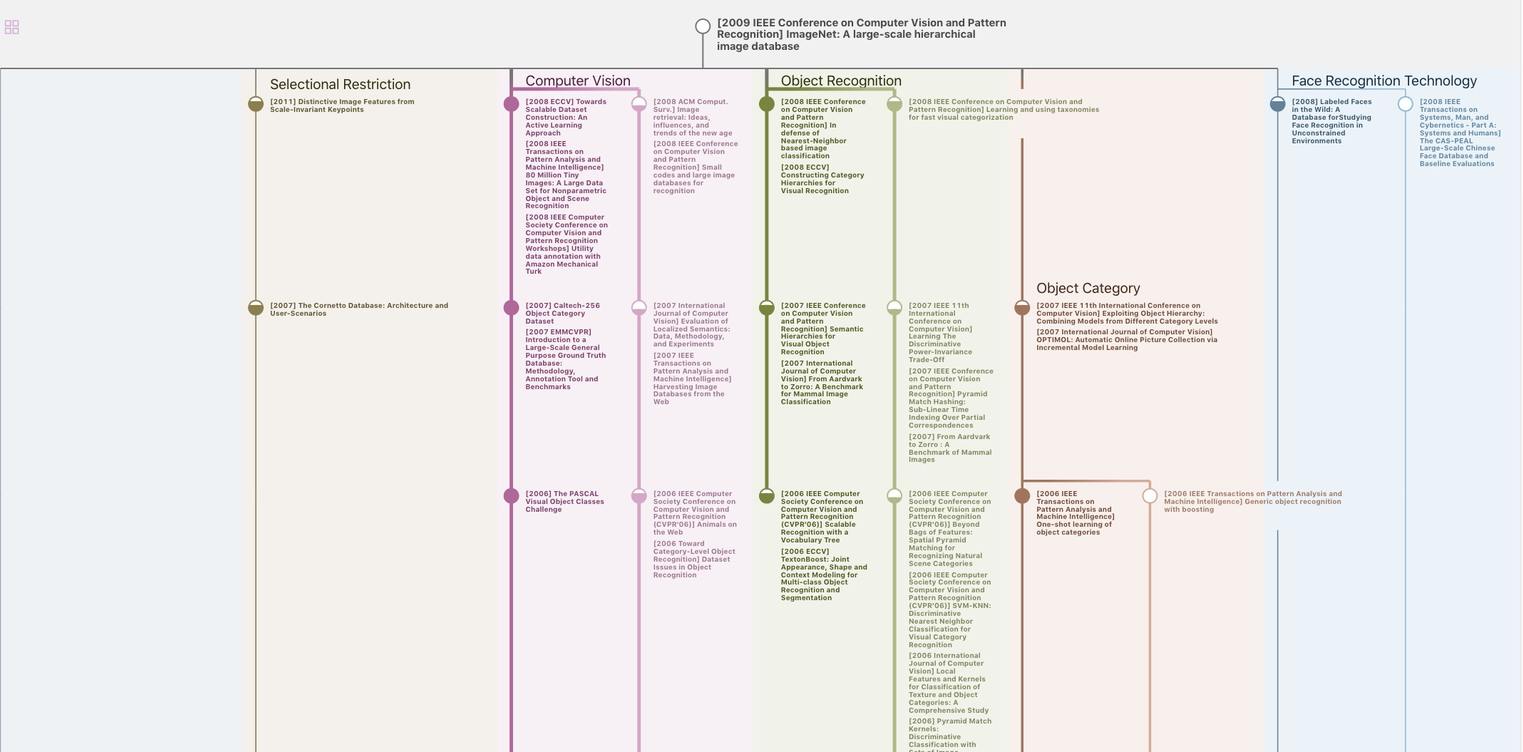
生成溯源树,研究论文发展脉络
Chat Paper
正在生成论文摘要