Developed Fall Detection of Elderly Patients in Internet of Healthcare Things
CMC-COMPUTERS MATERIALS & CONTINUA(2023)
摘要
Falling is among the most harmful events older adults may encounter. With the continuous growth of the aging population in many societies, developing effective fall detection mechanisms empowered by machine learning technologies and easily integrable with existing healthcare systems becomes essential. This paper presents a new healthcare Internet of Health Things (IoHT) architecture built around an ensemble machine learning-based fall detection system (FDS) for older people. Compared to deep neural networks, the ensemble multi-stage random forest model allows the extraction of an optimal subset of fall detection features with minimal hyperparameters. The number of cascaded random forest stages is automatically optimized. This study uses a public dataset of fall detection samples called SmartFall to validate the developed fall detection system. The SmartFall dataset is collected based on the acquired measurements of the three-axis accelerometer in a smartwatch. Each scenario in this dataset is classified and labeled as a fall or a non-fall. In comparison to the three machine learning models-K-nearest neighbors (KNN), decision tree (DT), and standard random forest (SRF), the proposed ensemble classifier outperformed the other models and achieved 98.4% accuracy. The developed healthcare IoHT framework can be realized for detecting fall accidents of older people by taking security and privacy concerns into account in future work.
更多查看译文
关键词
fall detection,elderly patients
AI 理解论文
溯源树
样例
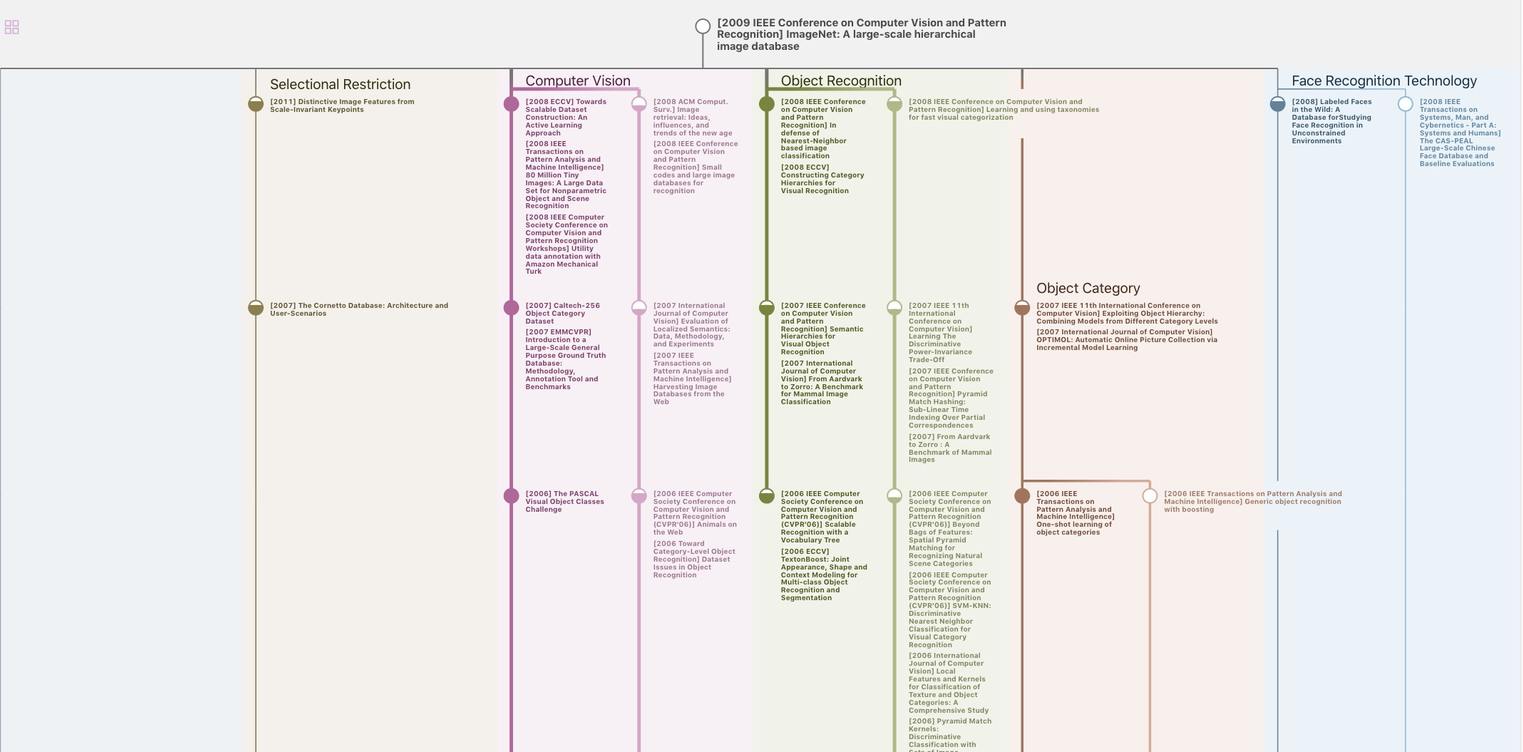
生成溯源树,研究论文发展脉络
Chat Paper
正在生成论文摘要