A graph neural network-based data cleaning method to prevent intelligent fault diagnosis from data contamination
ENGINEERING APPLICATIONS OF ARTIFICIAL INTELLIGENCE(2023)
摘要
The success of deep learning (DL) based-mechanical fault diagnosis hinges on the high quality of training data. However, it is difficult to acquire high-quality mechanical monitoring data due to data contamination: 1) Monitoring device irregularities, such as sensor malfunction and signal transmission disruption, bring anomalies into the training data; 2) human labour-based data annotation inevitably produces incorrectly labeled data. These two types of data contamination degrade the performance of DL models. To address the aforementioned issue, this paper proposes a graph neural network-based data-cleaning method. In the first stage, a group anomaly detector is designed to identify the presence of anomalous data. This detector incorporates affinity graphs for depicting data groups and subsequently calculates the group anomaly score to determine the abnormal group. In the second stage, a graph clustering model is developed to relabel the mislabeled data. This model takes advantage of the graph neural network's proficiency in handling affinity graphs to prepare clean labels for subsequent network training. Experimental results, conducted on a pump and an industrial robot joint reducer, show the proposed method's ability to effectively detect anomalous data and rectify incorrect labeling, surpassing the performance of baseline methods in mechanical fault diagnosis.
更多查看译文
关键词
Mechanical fault diagnosis,Data cleaning,Graph neural network,Graph clustering
AI 理解论文
溯源树
样例
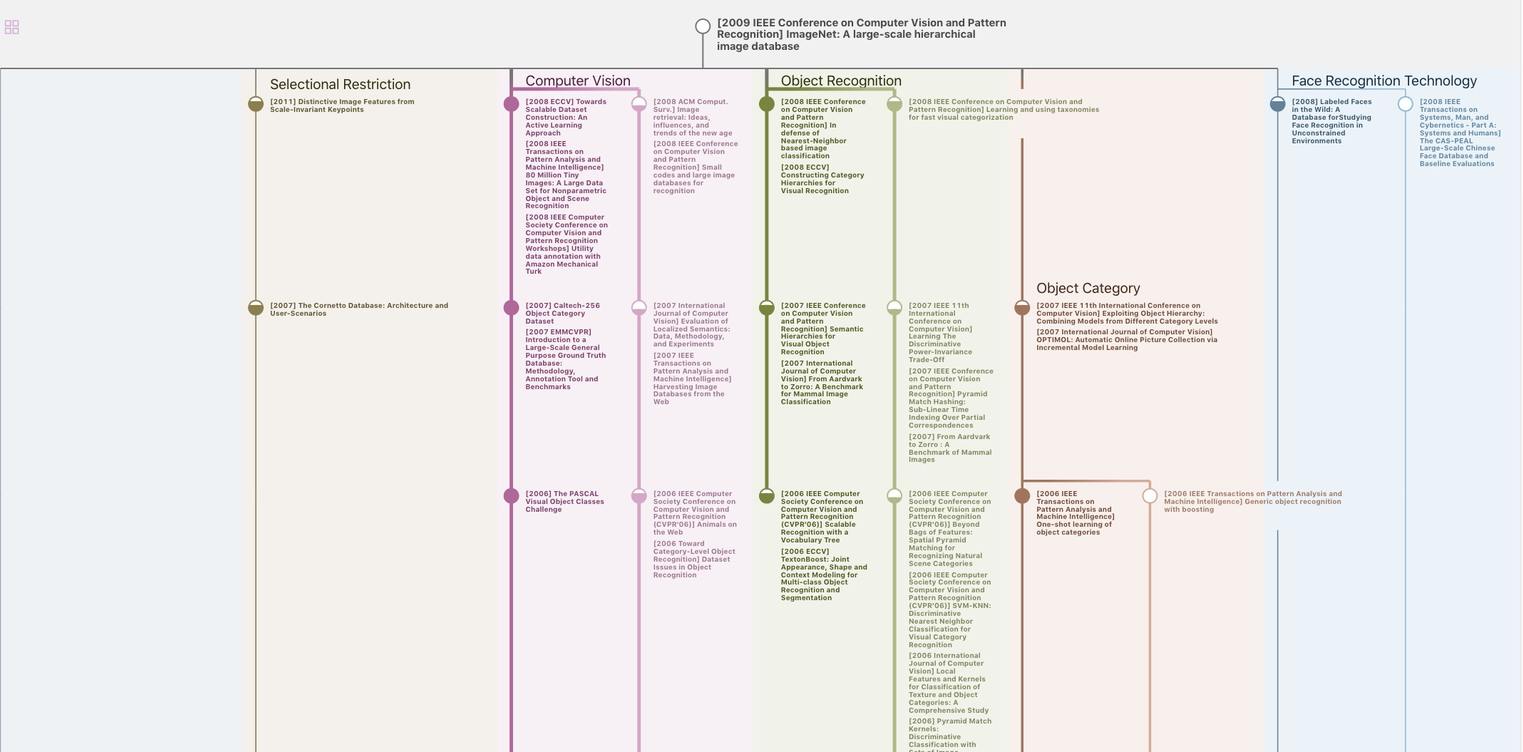
生成溯源树,研究论文发展脉络
Chat Paper
正在生成论文摘要