Robust key parameter identification of dedicated hybrid engine performance indicators via K-fold filter collaborated feature selection
ENGINEERING APPLICATIONS OF ARTIFICIAL INTELLIGENCE(2023)
摘要
Dedicated hybrid engine technology using auxiliary electronic components has been proven as an energy-saving solution to public concerns about energy consumption and carbon emissions. This paper proposes a generic approach of K-fold filter-collaborated feature selection (KFFC-FS) to robustly identify the key parameters of three engine performance indicators, i.e., volumetric efficiency, thermal efficiency, and fuel consumption. By using this approach, five filters are collaborated to provide a robust rank of feature importance and avoid the feature overestimation caused by the single filter. Meanwhile, the K-fold cross validation method is introduced to avoid random precision issues and overfitting, further enhancing the robustness of key parameter identification for the independent engine performance indicators. In this research, the modelling data is collected from an experi-mental test bench with a BYD 1.5L gasoline engine. Under the basics of the studied three engine performance indicators by using a multiple-layer perceptron network, the proposed approach further reduces by at least 10.3% root-mean-square error (RMSE) and at least 30% reduction of the model inputs.
更多查看译文
关键词
Data-driven modelling,Feature selection,Parameter identification,K-fold cross validation,Internal combustion engine
AI 理解论文
溯源树
样例
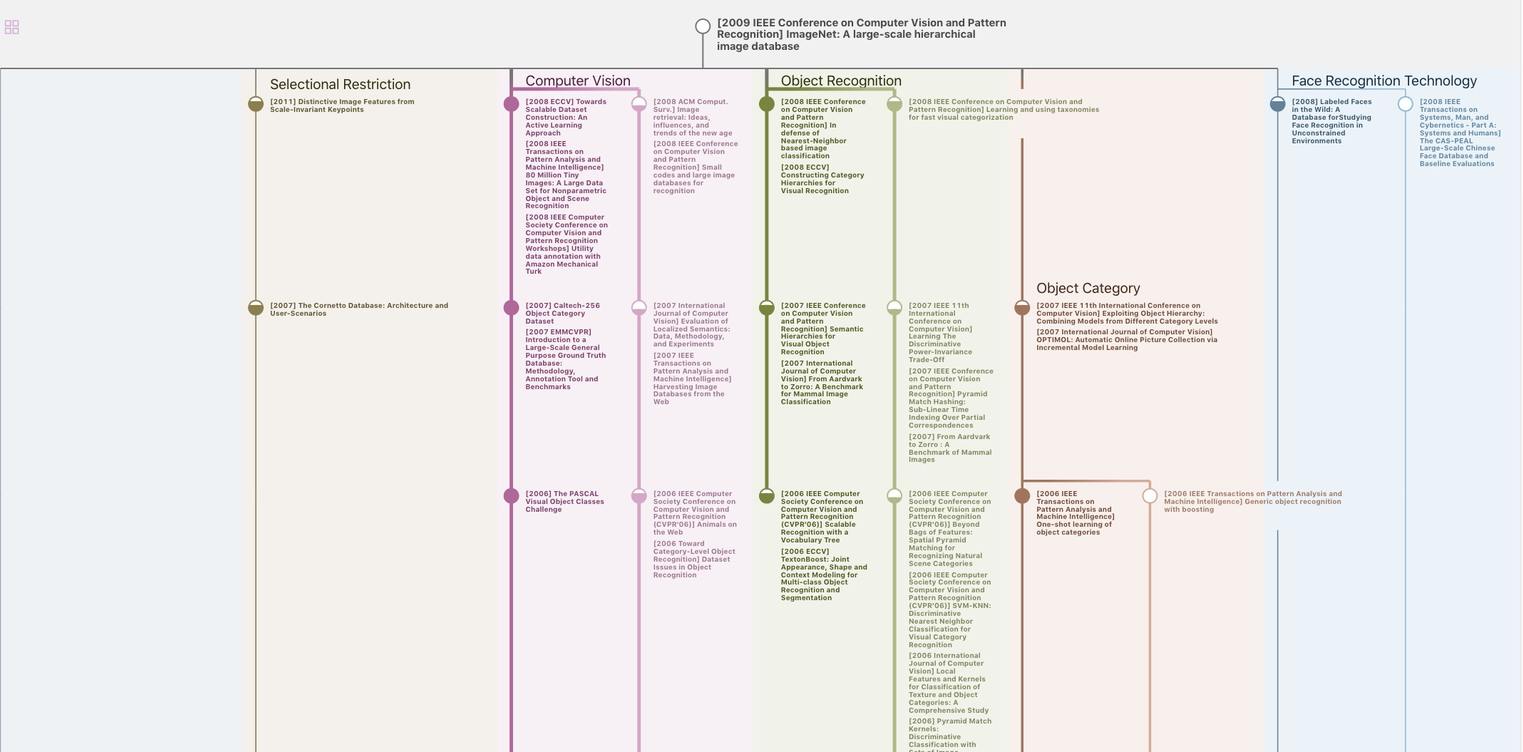
生成溯源树,研究论文发展脉络
Chat Paper
正在生成论文摘要