Measuring similarity based on user activeness in recommender systems to improve algorithm scalability
ENGINEERING APPLICATIONS OF ARTIFICIAL INTELLIGENCE(2023)
摘要
Recommender systems analyze user preferences and predict their preferred products based on available data, saving users time in the search for preferred products. However, accurate recommendations for both the system and the user may require complicated computations or the need to store more neighbors, leading to a less scalable algorithm. To address this issue, we define user activity coefficients and then analyze the relationship between different activity coefficients and similarity to design a novel approach for measuring user activity-based similarity in recommender systems. By examining the similarity of inactive user groups, our approach considers the accurate calculation of their similarity as one of the keys to influencing the number of required neighbors for optimal performance. As compared to several advanced collaborative filtering algorithms on two datasets, the algorithm developed in this paper can reduce the number of neighbors by 35.58%–75.38% while maintaining high prediction accuracy. The results indicate that user activity in recommender systems has a significant impact on similarity evaluation, which should be considered in relevant models.
更多查看译文
关键词
Recommender systems,Scalability,Collaborative filtering,User activeness,Prediction accuracy
AI 理解论文
溯源树
样例
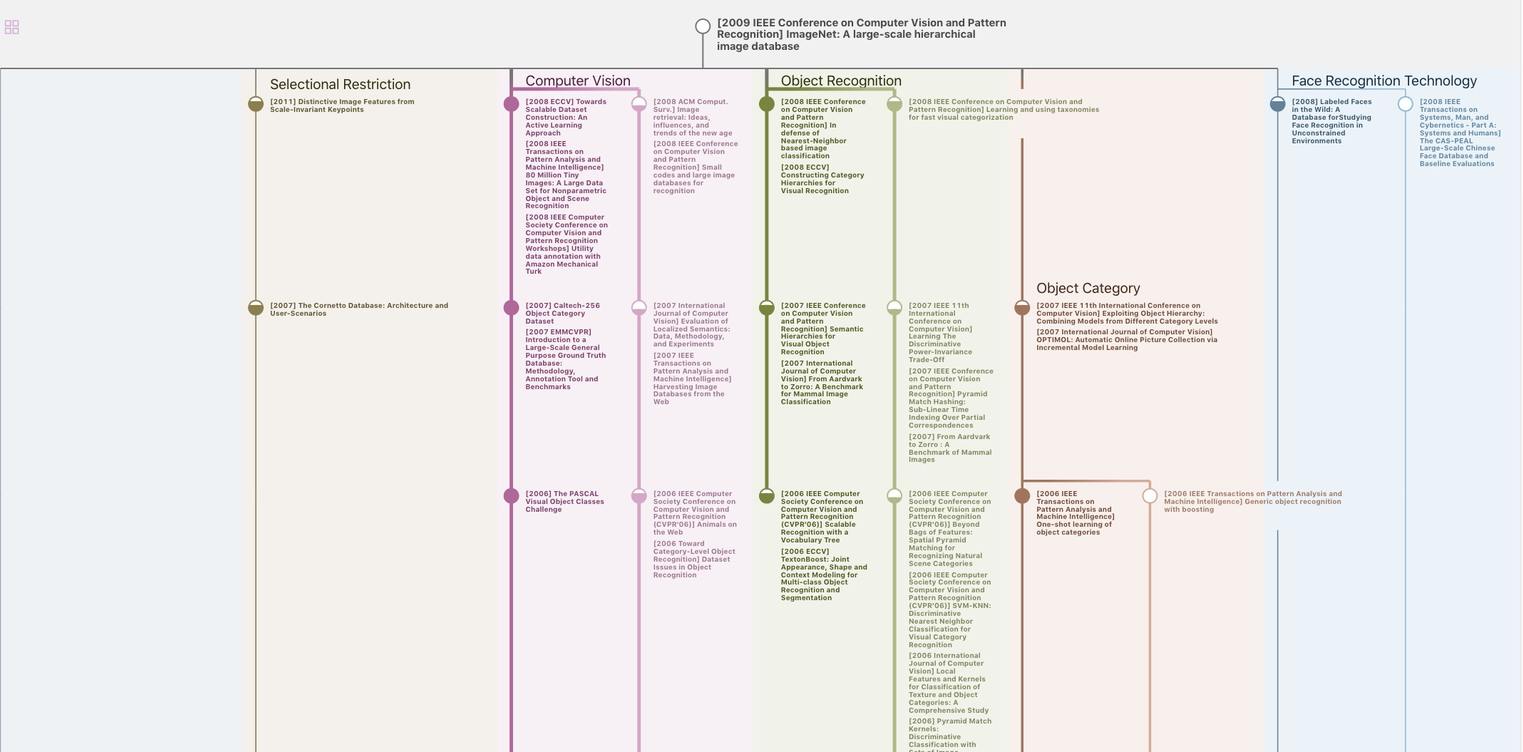
生成溯源树,研究论文发展脉络
Chat Paper
正在生成论文摘要