Multilevel Self-Training Approach for Cross-Domain Semantic Segmentation in Intelligent Vehicles
IEEE INTELLIGENT TRANSPORTATION SYSTEMS MAGAZINE(2024)
摘要
The use of intelligent vehicle technology is increasing; however, this technology requires further improvement. Semantic segmentation enables intelligent vehicles to understand the environment. Although advances have been achieved in a deep learning model for semantic segmentation, large-scale real-world datasets with manual pixel-level annotations, which are expensive, are required for adequately training these models. Unsupervised domain adaptation (UDA) for semantic segmentation enables training on a synthetic source domain and allows a model to be adapted to the real-world target domain without target domain annotations. Self-training is a highly effective UDA method; however, current UDA methods struggle to shift domains effectively and rely heavily on aggressive image-based perturbations for consistency training, resulting in poor-quality pseudolabels during the early training phase and decreased training efficiency. Therefore, we propose a novel multilevel self-training UDA method for semantic segmentation. First, a feature guidance and resampling strategy is adopted to improve the learning of rare classes in the source domain, and a spatial-aware mixing method is then used for gradually and smoothly eliminating the domain gap through training in various cross-domain intermediate spaces. In addition, we developed an instance and prototype feature consistency technique that explores optimization in the feature space to improve the training process by increasing model consistency. We conducted large-scale experiments on two challenging UDA benchmarks, "GTA5-to-Cityscapes" and "SYNTHIA-to-Cityscapes." Our method achieved similar or superior performance to that of previously developed UDA methods for semantic segmentation and has advantages in terms of training stability and convergence efficiency.
更多查看译文
关键词
Training,Semantic segmentation,Adaptation models,Semantics,Predictive models,Prototypes,Stability analysis
AI 理解论文
溯源树
样例
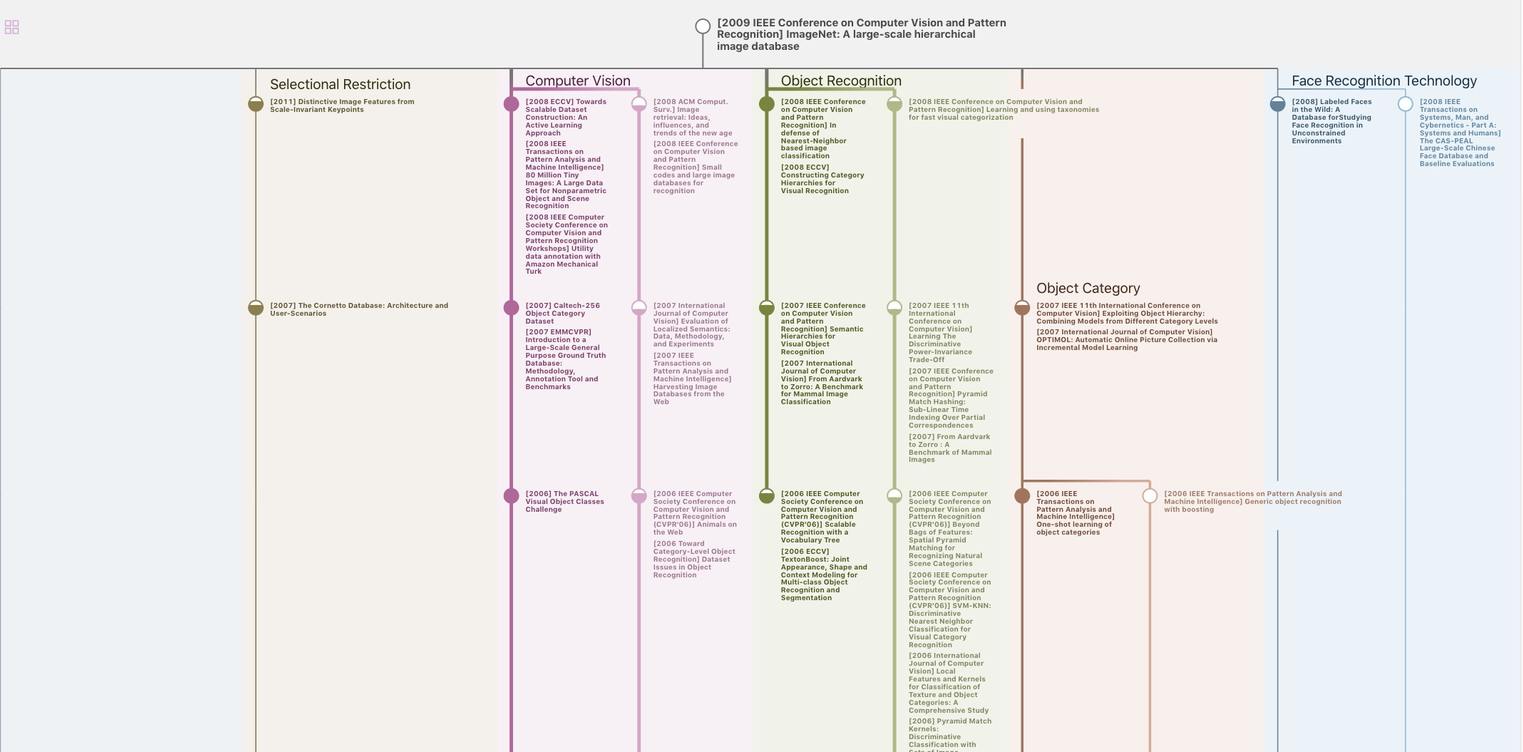
生成溯源树,研究论文发展脉络
Chat Paper
正在生成论文摘要