A Reinforcement Learning and Prediction-Based Lookahead Policy for Vehicle Repositioning in Online Ride-Hailing Systems
IEEE TRANSACTIONS ON INTELLIGENT TRANSPORTATION SYSTEMS(2023)
摘要
Existing approaches for vehicle repositioning on large-scale ride-hailing platforms either ignore the spatial -temporal mismatch between supply and demand in real-time or overlook the long-term balance of the system. To account for both, we propose a lookahead repositioning policy in this paper, which is a novel approach to repositioning idle vehicles from both a dynamic system and a long-term performance perspective. Our method consists of two parts; the first part utilizes linear programming (LP) to formulate the nonstationary system as a time-varying, T-step lookahead optimization problem and explicitly models the fraction of drivers who follow repositioning recommendations (called the repositioning rate). The second step is to incorporate a reinforcement learning (RL) method to maximize long-term return based on learned value functions after the T time slots. Extensive studies utilizing a real-world dataset on both small-scale and large-scale simulators show that our method outperforms previous baseline methods and is robust to prediction errors.
更多查看译文
关键词
Ride-hailing,large-scale,reposition,idle car routing,reinforcement learning
AI 理解论文
溯源树
样例
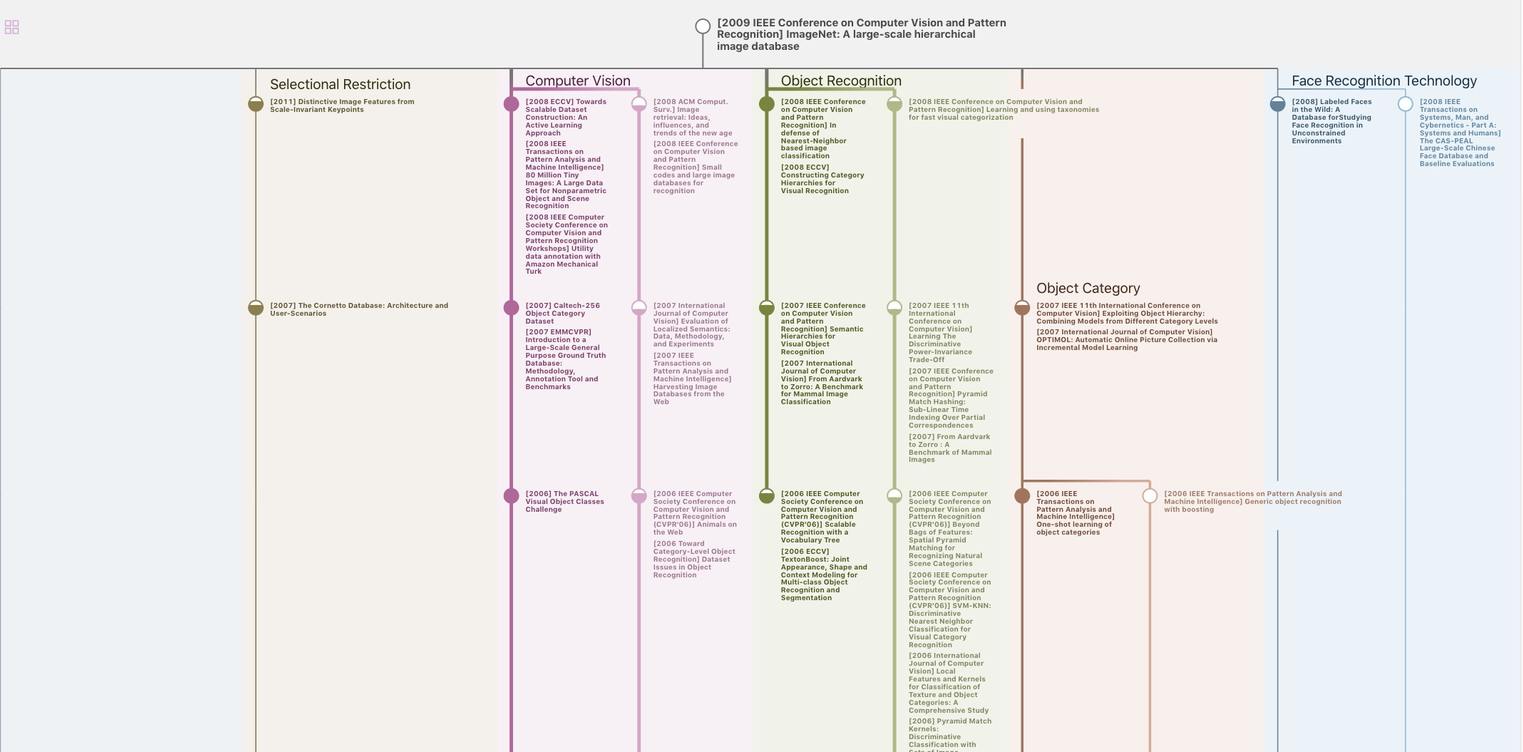
生成溯源树,研究论文发展脉络
Chat Paper
正在生成论文摘要