SegTransConv: Transformer and CNN Hybrid Method for Real-Time Semantic Segmentation of Autonomous Vehicles
IEEE TRANSACTIONS ON INTELLIGENT TRANSPORTATION SYSTEMS(2023)
摘要
Real-time and high-performance semantic segmentation is a crucial task in the scene understanding of autonomous vehicles. This paper focuses on this issue and proposes a transformer and convolutional neural networks (CNN) hybrid encoder-decoder structure SegTransConv. Firstly, we present a four-stage hierarchical encoder, and the feature extractor in each stage is composed of two transformer layers and CNN modules in series. In this way, the encoder better exploits the global contexts of the input and expands the receptive fields. In the U-shape decoder, the feature maps are upsampled through the proposed feature enhancement upsampling module (FE_Up). Then the knowledge distillation strategy is leveraged to improve the model performance under the guidance of the teacher network STDCNet. Finally, a novel evaluation metric is designed to comprehensively assess the accuracy, speed, floating-point operations (FLOPs), and parameters of real-time segmentation methods. Extensive experiments on two public datasets and self-collected images have evaluated the effectiveness of our method. SegTransConv-A and SegTransConv-B obtain 72.8% and 73.0% mIoU, respectively, at the inference speed of 68.0 FPS with an input resolution of 1024 x 512.
更多查看译文
关键词
Autonomous driving,deep learning,real-time semantic segmentation,transformer
AI 理解论文
溯源树
样例
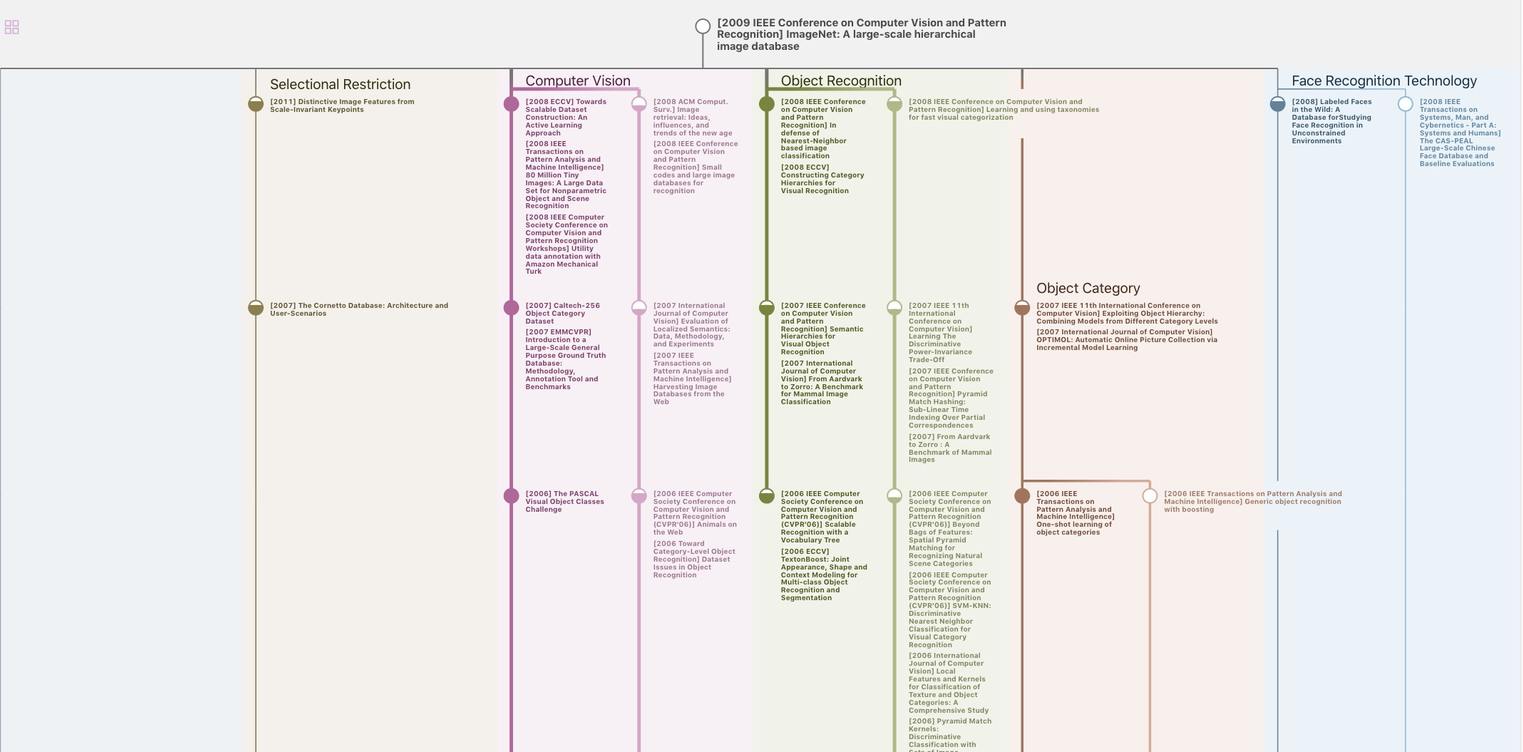
生成溯源树,研究论文发展脉络
Chat Paper
正在生成论文摘要