A Flexible and Robust Tensor Completion Approach for Traffic Data Recovery With Low-Rankness
IEEE TRANSACTIONS ON INTELLIGENT TRANSPORTATION SYSTEMS(2023)
摘要
Data missing phenomena and random anomalies are ubiquitous in intelligent transportation systems (ITS), resulting in poor data quality and usability, which is a major impediment to real-world ITS applications. Most studies regarding traffic data recovery either assume that the original data are clean or complete, while such two issues often coexist in reality due to inevitable data measurement errors like detector malfunctions. In this paper, we fully exploit the algebraically low-rank property of traffic spatiotemporal data and develop an innovative tensor completion approach (termed SCPN) based on the tensor Schatten capped p norm, a unified representation of tensor norms with a high flexibility. Furthermore, we extend the proposed method to a robust form (termed RSCPN) by leveraging the sparsity of unstructured outliers, with the aim to reconstruct ground-truth values from corrupted and incomplete observations. Finally, associated optimization solutions based on the alternating direction multiplier method are derived. Extensive experiments on four datasets substantiate the significant superiority of our proposed models over other state-of-the-art methods on both missing data imputation and corrupted data recovery tasks with miscellaneous simulated scenarios.
更多查看译文
关键词
Spatiotemporal traffic data,missing data imputation,corrupted data recovery,low-rank tensor completion
AI 理解论文
溯源树
样例
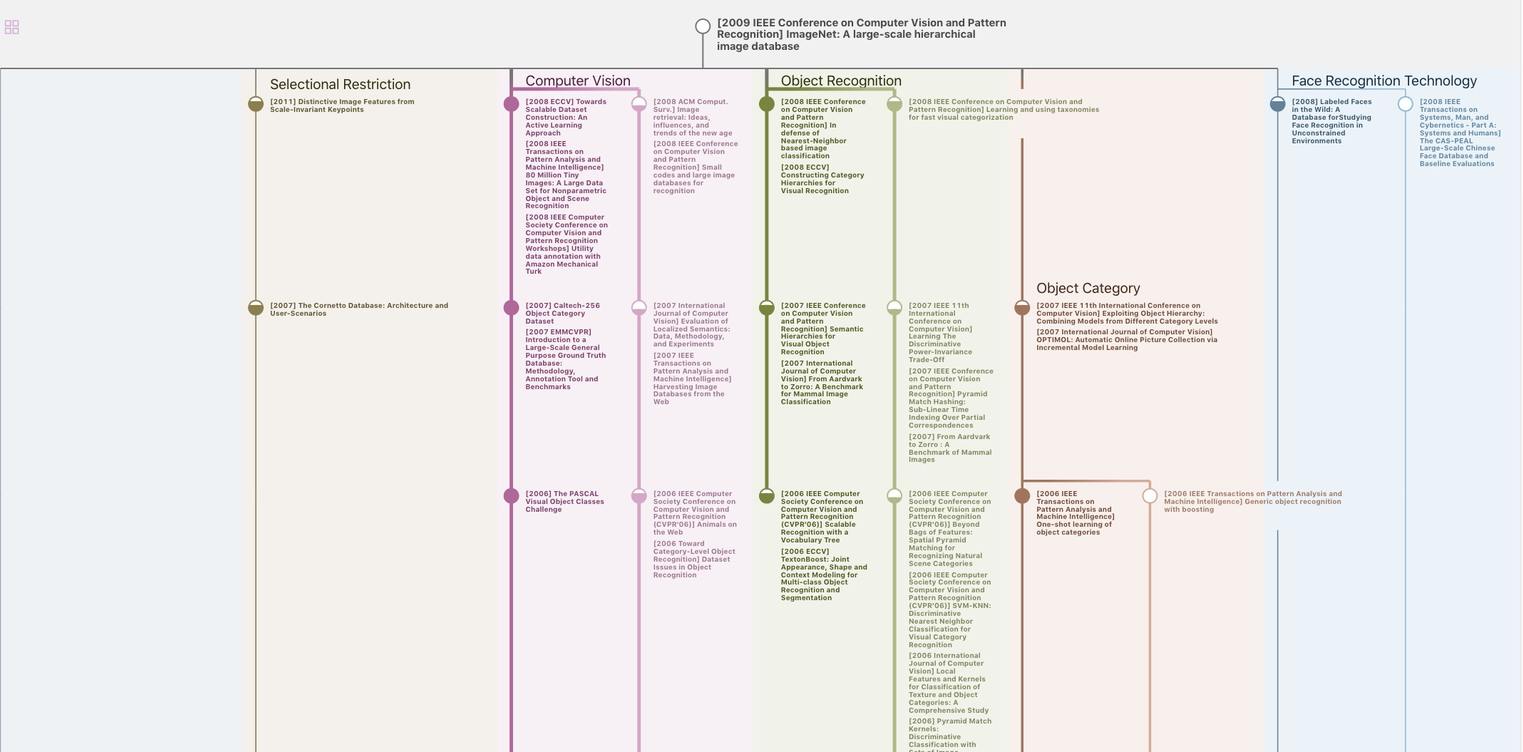
生成溯源树,研究论文发展脉络
Chat Paper
正在生成论文摘要