Evo-MAML: Meta-Learning with Evolving Gradient
ELECTRONICS(2023)
摘要
How to rapidly adapt to new tasks and improve model generalization through few-shot learning remains a significant challenge in meta-learning. Model-Agnostic Meta-Learning (MAML) has become a powerful approach, with offers a simple framework with excellent generality. However, the requirement to compute second-order derivatives and retain a lengthy calculation graph poses considerable computational and memory burdens, limiting the practicality of MAML. To address this issue, we propose Evolving MAML (Evo-MAML), an optimization-based meta-learning method that incorporates evolving gradient within the inner loop. Evo-MAML avoids the second-order information, resulting in reduced computational complexity. Experimental results show that Evo-MAML exhibits higher generality and competitive performance when compared to existing first-order approximation approaches, making it suitable for both few-shot learning and meta-reinforcement learning settings.
更多查看译文
关键词
gradient,evo-maml,meta-learning
AI 理解论文
溯源树
样例
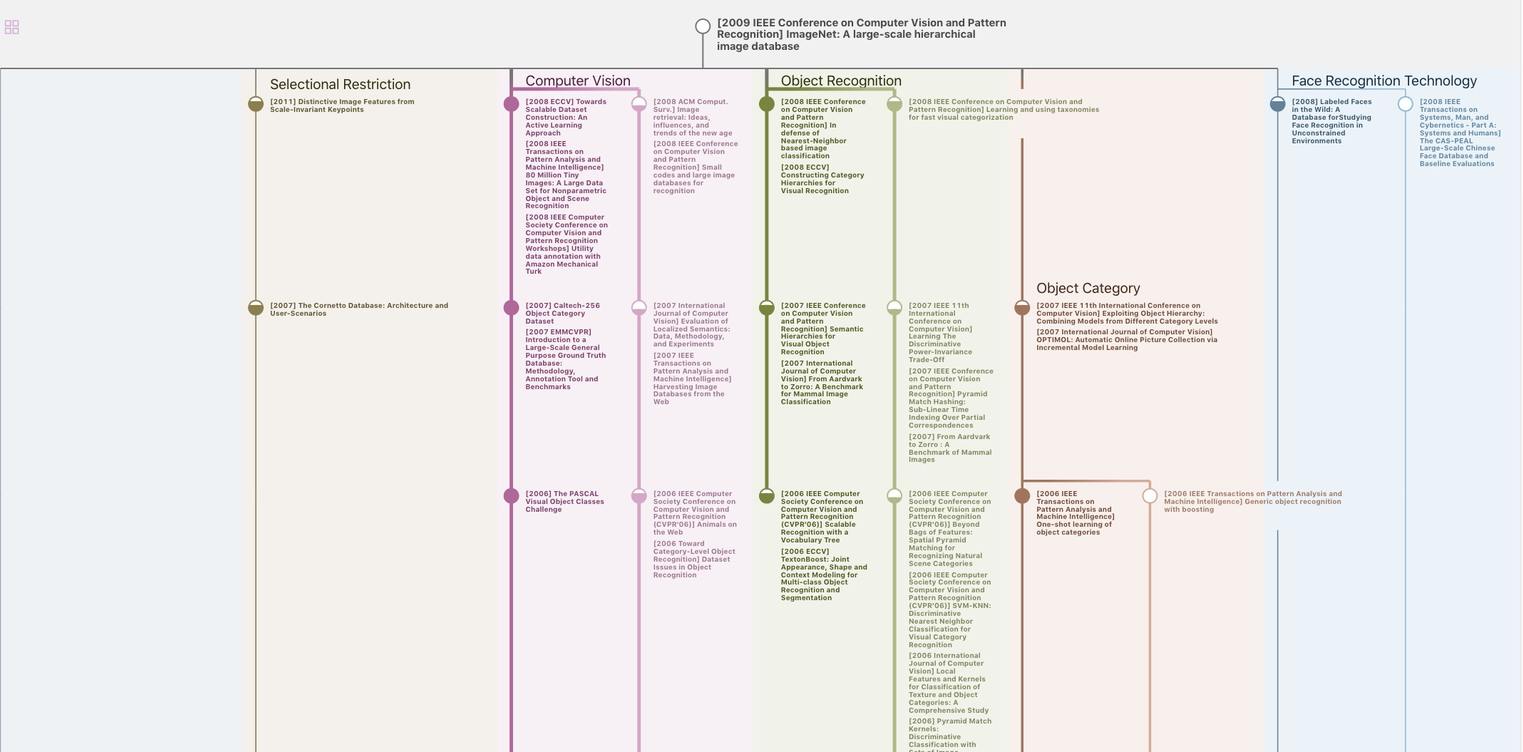
生成溯源树,研究论文发展脉络
Chat Paper
正在生成论文摘要