Spatio-Temporal Graph Neural Network for Traffic Prediction Based on Adaptive Neighborhood Selection
TRANSPORTATION RESEARCH RECORD(2023)
摘要
Traffic prediction is critical to intelligent transportation and smart cities. The prediction performance of many existing traffic prediction models is limited by the fixed original graph structure and inappropriate spatio-temporal dependency extraction. For this situation, this paper proposes a spatio-temporal graph neural network based on adaptive neighborhood selection (STGNN-ANS). To obtain more flexible graph structures, STGNN-ANS designs a neighbor selection mechanism to generate a new graph structure by filtering inappropriate neighbors. To further capture the spatio-temporal dependence of traffic data, a spatio-temporal serial module of STGNN-ANS adopts the bidirectional learning manner of bidirectional long short-term memory (BiLSTM) and the graph convolution network (GCN) enhanced by self-attention mechanism to reach excellent prediction accuracy in both short-range and long-range scenarios. In this paper, a new baseline comprehensive comparison metric (BCCM) is invented to cope with the complexity in the comparative analysis of large numbers of experimental results. Many experiments have been performed on four real-world traffic datasets, and the results show that the comprehensive prediction performance of STGNN-ANS is better than previous models.
更多查看译文
关键词
data and data science,artificial intelligence and advanced computing applications,artificial intelligence,deep learning,traffic predication
AI 理解论文
溯源树
样例
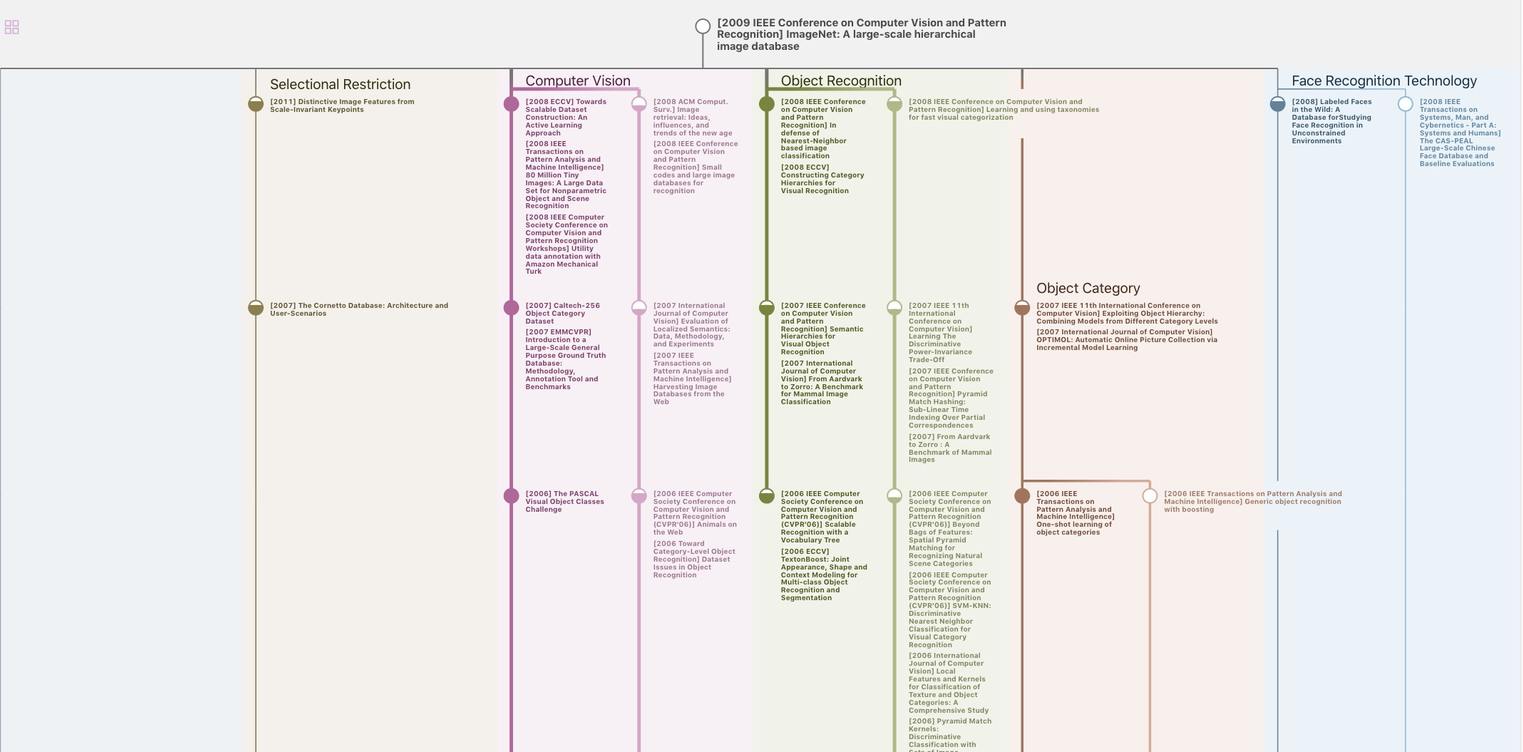
生成溯源树,研究论文发展脉络
Chat Paper
正在生成论文摘要