Artificial Intelligence Aided Low Complexity RRM Algorithms for 5G-MBS
IEEE TRANSACTIONS ON BROADCASTING(2023)
摘要
For the upcoming 5G-Advanced, the multicast/broadcast services (5G-MBS) capability is one of the most appealing use cases. The effective integration of point-to-multipoint communication will address the ever-growing traffic demands, disruptive multimedia services, massive connectivity, and low-latency applications. This paper proposes novel approaches for the dynamic access technique selection and resource allocation for multicast groups (MGs) subject to the 5G-MBS paradigm. Our proposal is oriented to address and contextualize the complexity associated with multicast radio resource management (RRM) and the implications of fast variations in the reception conditions of the MG members. We propose a solution structured by a multicast-oriented trigger to avoid overrunning the algorithm, a K-means clustering for group-oriented detection and splitting, a classifier for selecting the most suitable multicast access technique, and a final resource allocation algorithm. To choose the multicast access technique that better fits the specific reception conditions of the users, we evaluate heuristic strategies and machine learning (ML) multiclass classification solutions. We consider the conventional multicast scheme (MCS) and subgrouping based on orthogonal/non-orthogonal multiplex access (OMA/NOMA) as access techniques. We assess the effectiveness of our solution in terms of the quality of service (QoS) parameters and complexity. The proposed technical solution is validated through extensive simulation for a single-cell 5G-MBS use case in the microwave (mu Wave) and millimeter wave (mmWave) band with different mobility behaviors.
更多查看译文
关键词
5G-MBS,computational complexity,machine learning,mmWave,multicast access techniques,NOMA
AI 理解论文
溯源树
样例
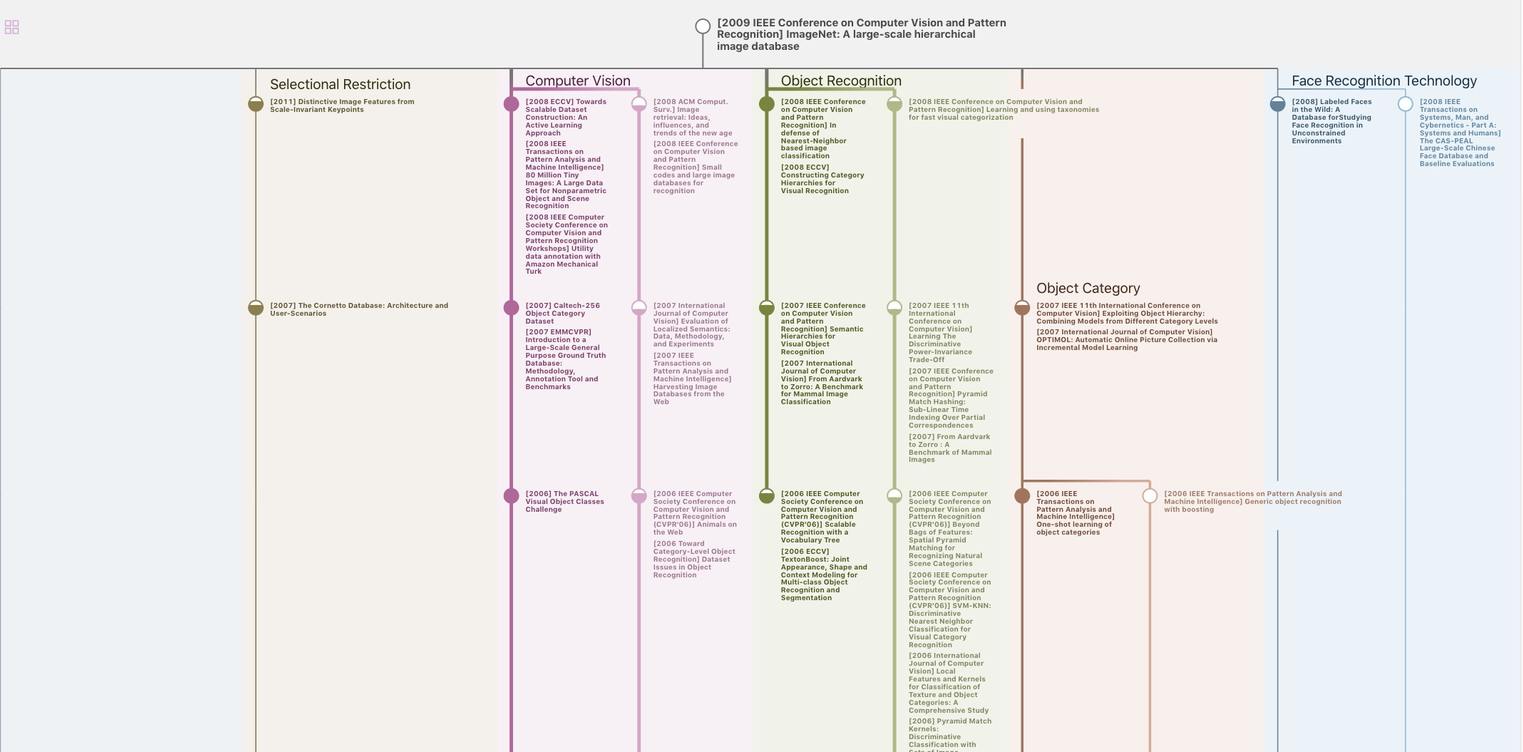
生成溯源树,研究论文发展脉络
Chat Paper
正在生成论文摘要