Relative permeability curve prediction from digital rocks with variable sizes using deep learning
PHYSICS OF FLUIDS(2023)
摘要
Recent advancements in artificial intelligence (AI) technology have offered new ways to obtain the relative permeability curve that is crucial for subsurface engineering problems. The existing data-driven AI approaches for the relative permeability prediction are only suitable for a specific geometry rock size and lack the cross-size prediction ability. In this paper, we propose a new physics-informed prediction model called the three-dimensional (3D) spatial pyramid pooling convolutional network (3DSPPConvNet) model embedding three important parameters: computed tomography (CT) scanning resolution, interfacial tension, and contact angle distribution. Our model can deal with 3D digital rocks with variable sizes as it is based on a multi-size digital rock dataset containing 1512 couples of relative permeability curves. Their relative permeability curves for training and testing are obtained from pore network modeling (PNM) results with a linear postprocessing process. The results show that the physics-informed 3DSPPConvNet has a high prediction accuracy of 95.3%, with scale information being the most important physics feature accounting for 51%. In addition, compared with PNM, the computational time and memory of the intelligent model are saved by 63% and 80%, respectively.
更多查看译文
关键词
relative permeability curve prediction,digital rocks,deep learning
AI 理解论文
溯源树
样例
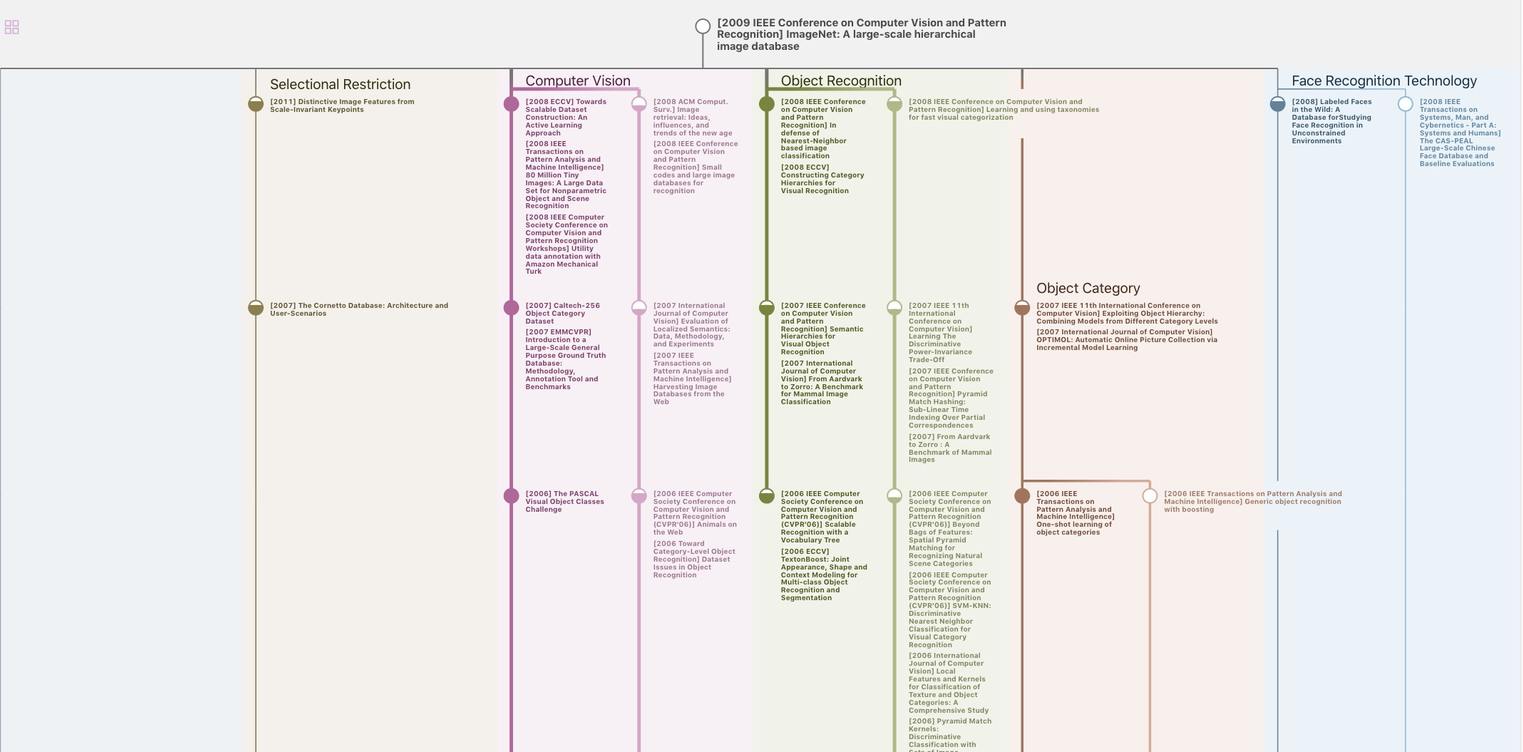
生成溯源树,研究论文发展脉络
Chat Paper
正在生成论文摘要