Towards Probabilistic Robust and Sparsity-Free Compressive Sampling in Civil Engineering: A Review
INTERNATIONAL JOURNAL OF STRUCTURAL STABILITY AND DYNAMICS(2023)
摘要
Compressive sampling (CS) is a novel signal processing paradigm whereby the data compression is performed simultaneously with the sampling, by measuring some linear functionals of original signals in the analog domain. Once the signal is sparse sufficiently under some bases, it is strictly guaranteed to stably decompress/reconstruct the original one from significantly fewer measurements than that required by the sampling theorem, bringing considerable practical convenience. In the field of civil engineering, there are massive application scenarios for CS, as many civil engineering problems can be formulated as sparse inverse problems with linear measurements. In recent years, CS has gained extensive theoretical developments and many practical applications in civil engineering. Inevitable modelling and measurement uncertainties have motivated the Bayesian probabilistic perspective into the inverse problem of CS reconstruction. Furthermore, the advancement of deep learning techniques for efficient representation has also contributed to the elimination of the strict assumption of sparsity in CS. This paper reviews the advancements and applications of CS in civil engineering, focusing on challenges arising from data acquisition and analysis. The reviewed theories also have applicability to inverse problems in broader scientific fields.
更多查看译文
关键词
Compressive sampling,sparsity,Bayesian inference,generative model,deep learning,structural health monitoring
AI 理解论文
溯源树
样例
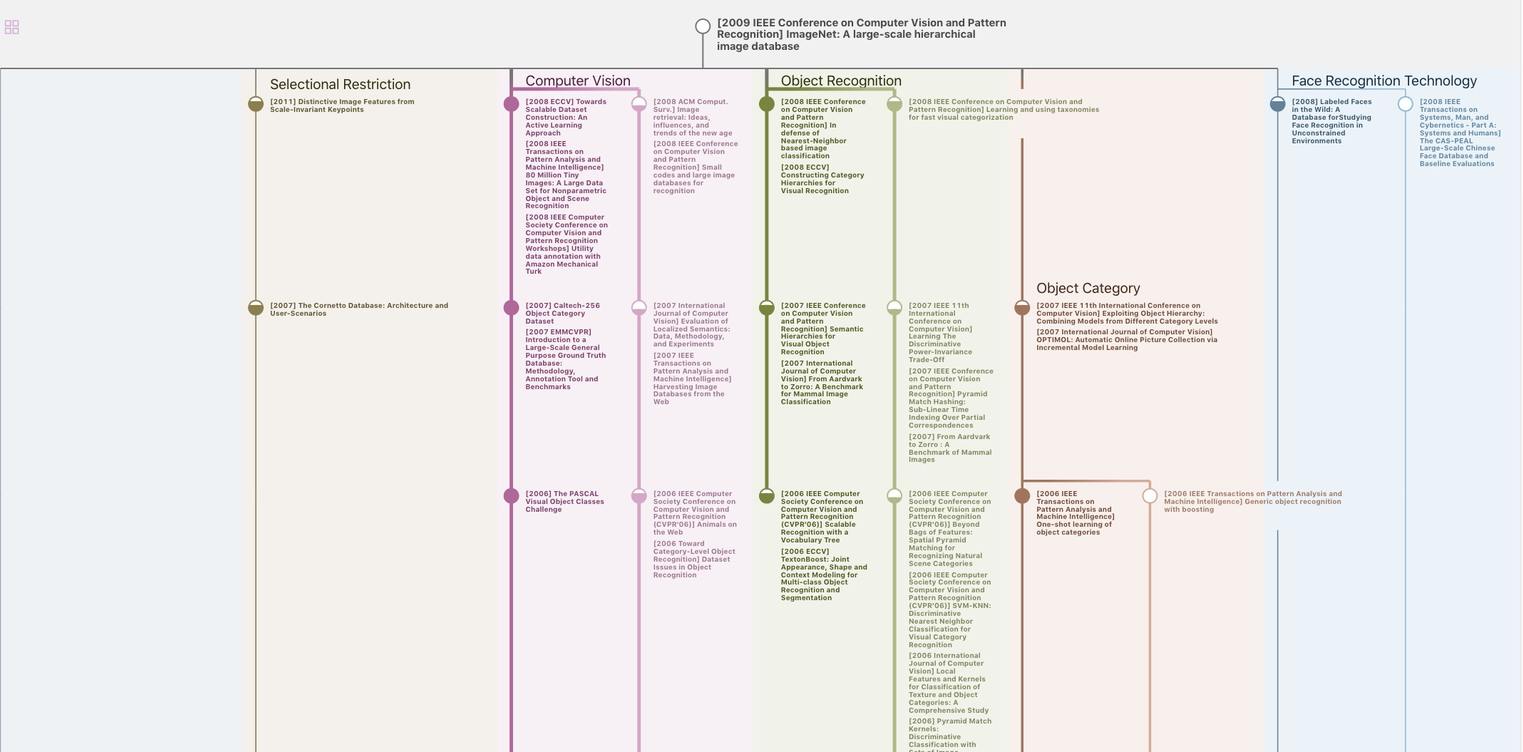
生成溯源树,研究论文发展脉络
Chat Paper
正在生成论文摘要