Finite Control-Set Learning Predictive Control for Power Converters
IEEE TRANSACTIONS ON INDUSTRIAL ELECTRONICS(2023)
摘要
This letter concentrates on introducing a learning methodology that extends and improves classical finite control-set model predictive control approach, which is able to significantly mitigate the inherent limitations of system uncertainties and unknown perturbations subject to robustness characteristics. To this end, in our work, a finite control-set learning predictive control architecture, which is addressed as an unsupervised learning technique, is presented. In this control task, we define a single neural network to learn the tracking control part online, and a robustifying control term is embedded into the suggested control solution so as to handle the approximator error and/or external disturbances, thereby leading to considerable enhancement of robustness. Dissimilar to classical finite control-set model predictive control, we establish that this method does not require a priori knowledge of model information and weighting factors, making our approach applicable to a variety of power converter systems. Finally, we highlight its advantages with a case study.
更多查看译文
关键词
Finite control-set model predictive control (FCS-MPC),neural network (NN),power converters,unsupervised learning
AI 理解论文
溯源树
样例
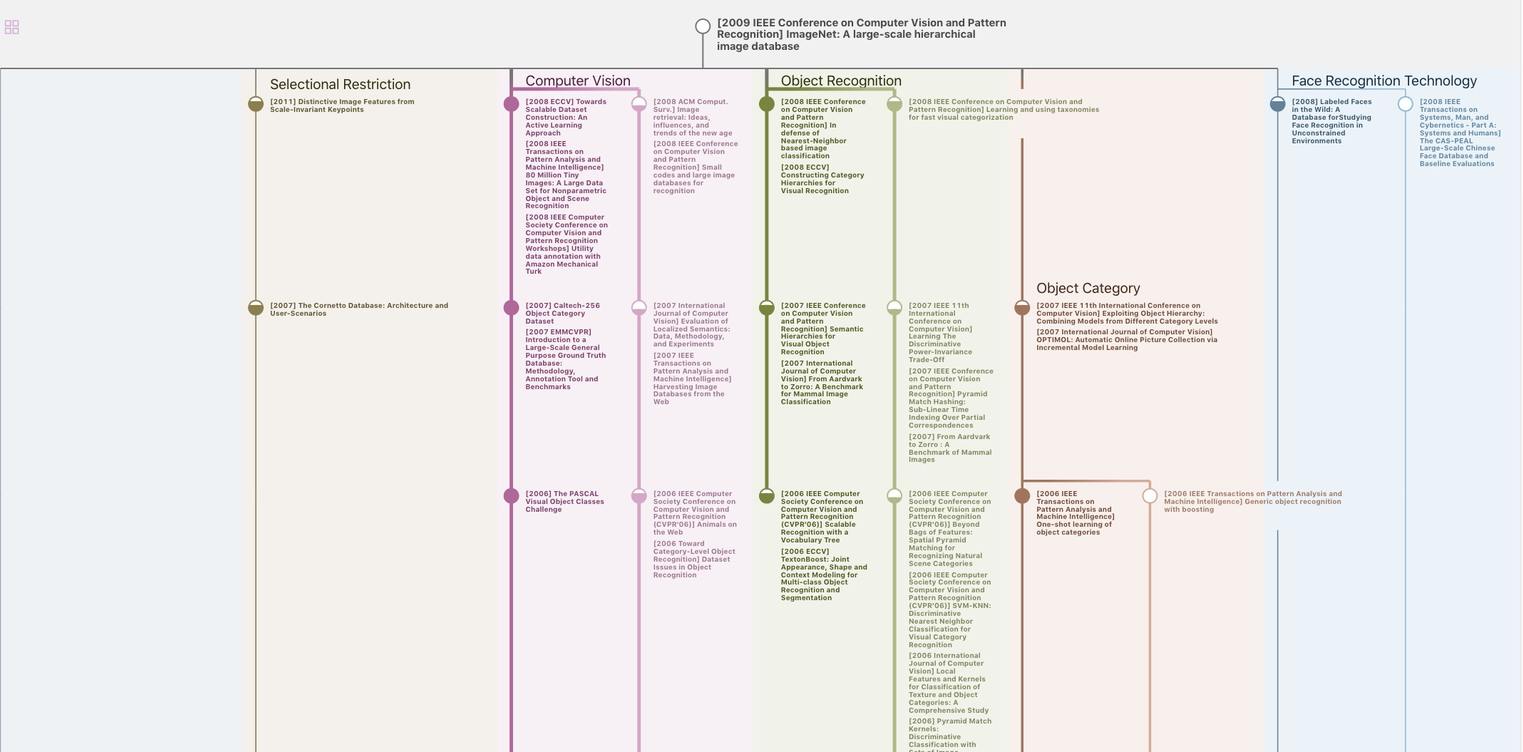
生成溯源树,研究论文发展脉络
Chat Paper
正在生成论文摘要