Simultaneous Design of Double-D Pad Coil and Core Geometry by Neural Network Optimisation
2023 IEEE WIRELESS POWER TECHNOLOGY CONFERENCE AND EXPO, WPTCE(2023)
摘要
This paper proposes a new design framework for an IPT pad for EV charging by optimisation with an artificial neural network. Many design parameters exist within an IPT pad, which affect magnetic performance, cost and mechanical robustness. The multivariate nature of the problem makes optimisation a suitable approach for semi-automated design of IPT pads. FEM simulation is used when ferrite cores are present, which slows the design process and makes it computationally expensive. Therefore, the proposed design approach replaces FEM with a neural network model, trading off accuracy for a faster search of the solution space. This paper uses genetic algorithm optimisation with neural network predictions, trained by FEM data, to design the coil and core layer geometry of a double-D primary pad. The neural network is capable of identifying multiple relationships between the design outputs and pad parameters, showing its ability to emulate the simulation physics. The selected optimal design replaces 48% ferrite with SMC over the typical matched full-ferrite primary, with a further 36% ferrite directly removed. The maximum leakage field has dropped by 30 %, while the coupling has only lowered by 3 %.
更多查看译文
关键词
inductive power transfer,wireless power transfer,optimisation,machine learning,neural network
AI 理解论文
溯源树
样例
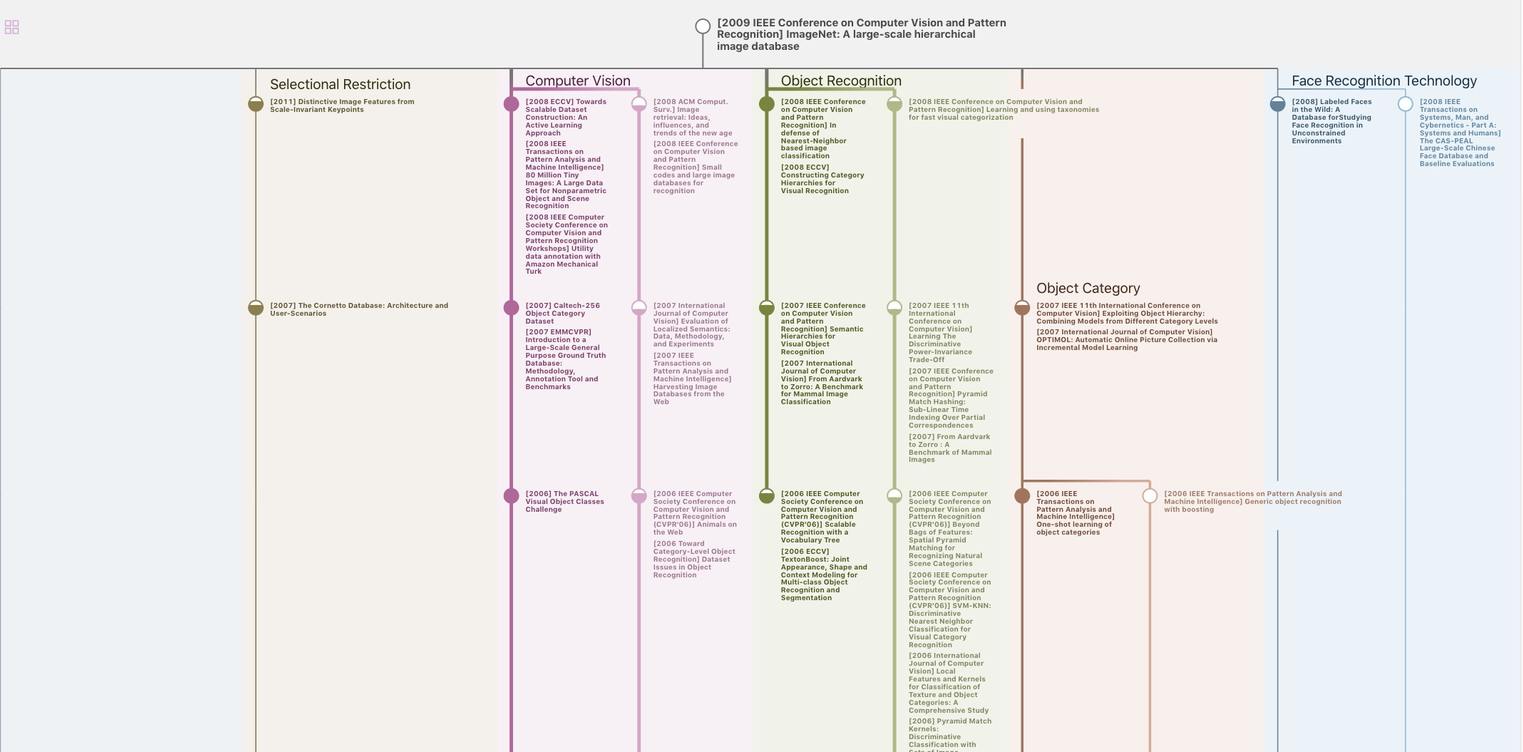
生成溯源树,研究论文发展脉络
Chat Paper
正在生成论文摘要