Improving exploration in deep reinforcement learning for stock trading
INTERNATIONAL JOURNAL OF COMPUTER APPLICATIONS IN TECHNOLOGY(2023)
摘要
Deep reinforcement learning techniques have become quite widespread over the last decades. One challenge is the Exploration-Exploitation Dilemma. Although many exploration techniques for single-agent and multi-agent deep reinforcement learning are proposed and have shown promising results in various domains, their value has not yet been demonstrated in the financial markets. In this paper, we will apply the NoisyNet-DQN method, which was previously tested and brought promising results in Atari games, to the stock trading problem. The trained reinforcement learning agent is employed to trade the S&P500 ETF (SPY) data set. Findings show that this approach can encounter the best trading action to choose at a specific moment and outperforms the classical DQN (Deep QNetwork) method.
更多查看译文
关键词
deep reinforcement learning,exploration,stock trading
AI 理解论文
溯源树
样例
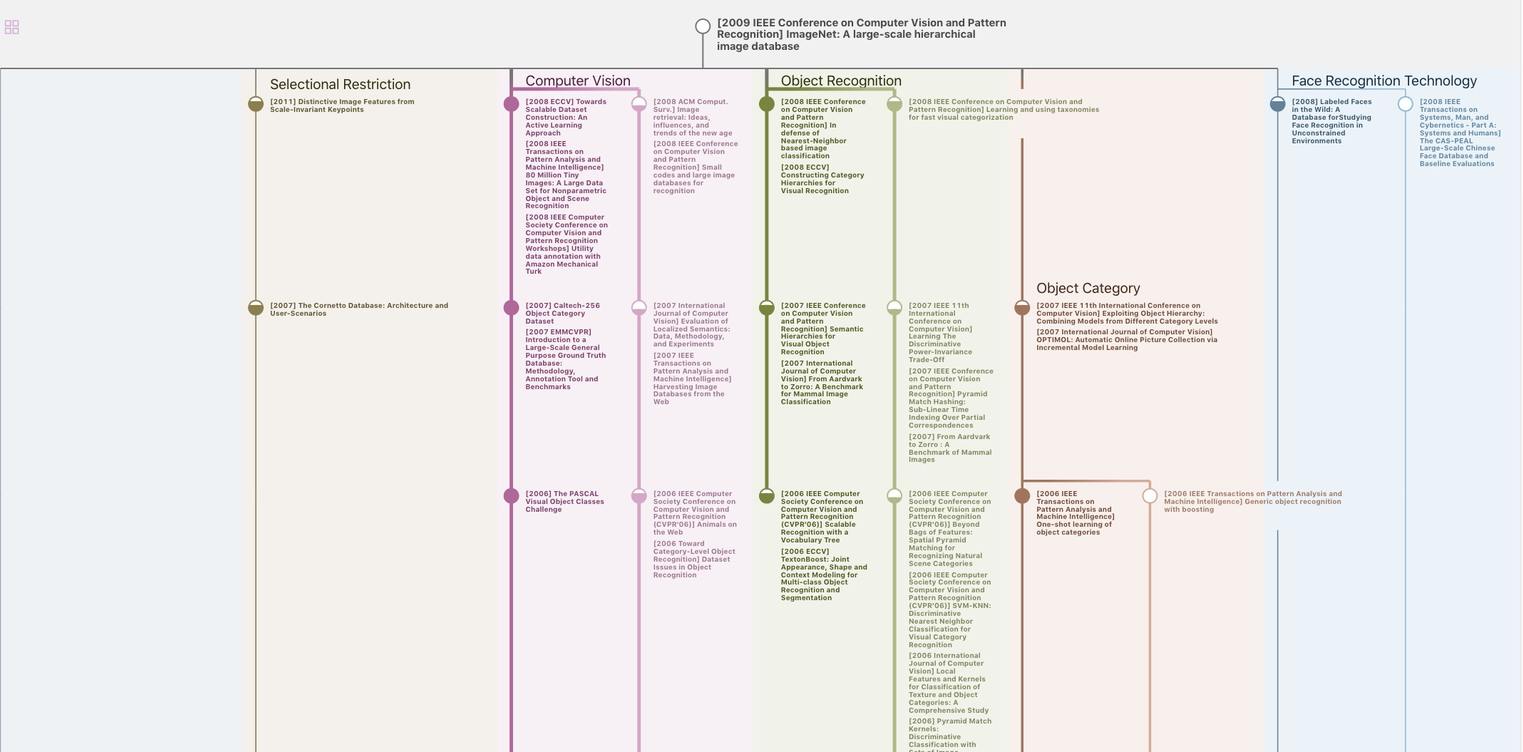
生成溯源树,研究论文发展脉络
Chat Paper
正在生成论文摘要