Common latent representation learning for low-resourced spoken language identification
MULTIMEDIA TOOLS AND APPLICATIONS(2023)
摘要
The i-vector method is one of the mainstream methods in spoken language identification (SLID). It estimates the total variability space (TVS) to obtain a low-rank representation which can characterize the language, called the i-vector. However, on small-scale datasets, low learning resources can significantly degrade the performance of SLID system. Therefore, it is necessary to improve the performance of SLID system in low-resourced condition. In this paper, we propose a common latent representation learning (CLRL) method to learn the TVS, which introduces prior information to address the lack of information in low-resourced condition. The prior information includes category label and parameter prior hypothesis. The CLRL method is evaluated on the OLR2020 dataset. Compared with other state-of-the-art methods, the CLRL method shows better performance on all datasets of different data scales. Moreover, the CLRL method can effectively improve the performance of the SLID system on low-resourced/small-scale datasets.
更多查看译文
关键词
Spoken language identification,Total variability space,I-vector,Common latent representation learning
AI 理解论文
溯源树
样例
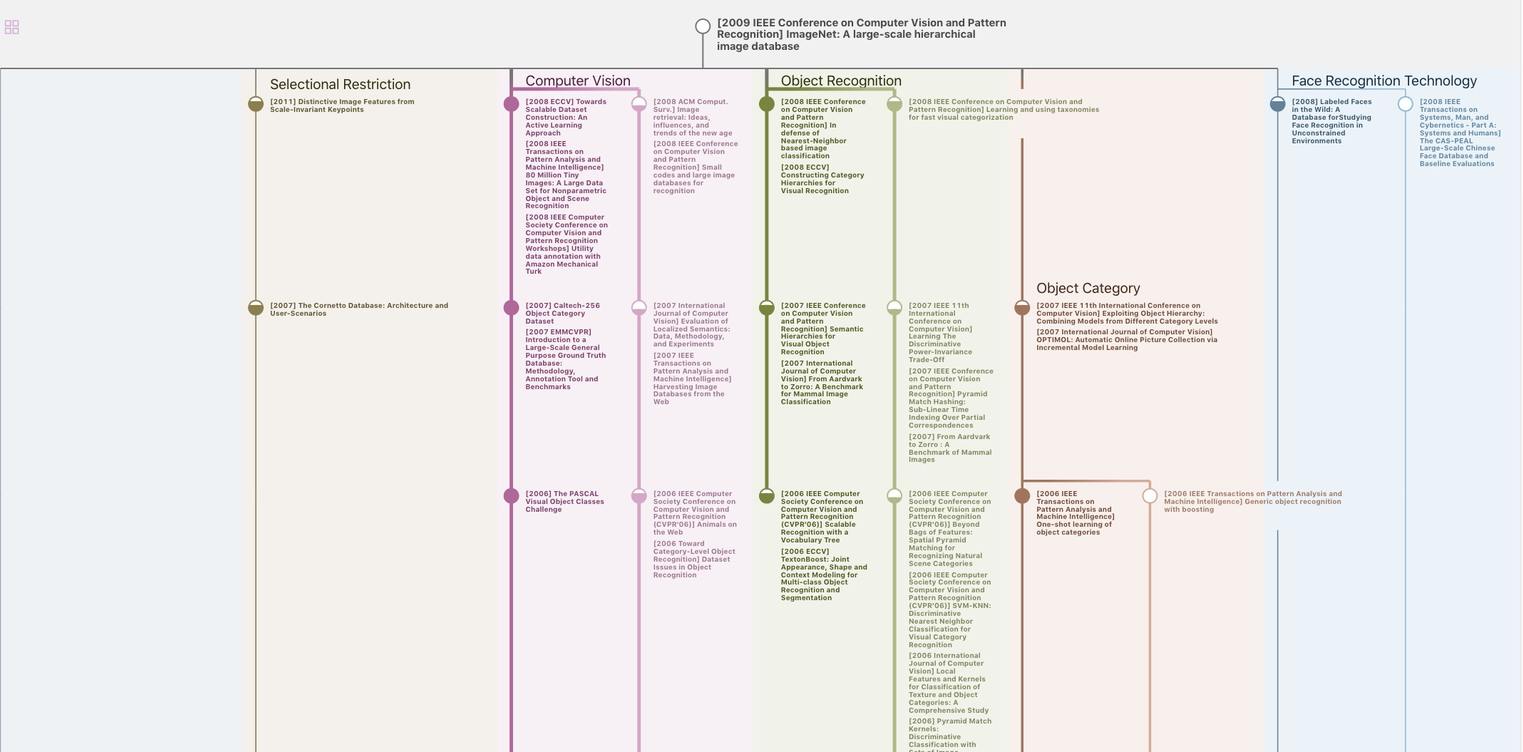
生成溯源树,研究论文发展脉络
Chat Paper
正在生成论文摘要