Comparative study of different machine learning models for automatic diabetic retinopathy detection using fundus image
MULTIMEDIA TOOLS AND APPLICATIONS(2023)
摘要
Diabetics suffer from an eye condition called diabetic retinopathy (DR), which can lead to vision loss. The main region affected is the blood vessels in the retina. A large proportion of the world's population is suffering from the harmful effects of diabetes, and most of them are not recognized early. Severe vision loss can be reduced through early detection, diagnosis, and treatment efficiency. The manual errors and tedious work of ophthalmologists can be reduced by using computer-assisted automatic diagnosis of DR. This paper provides a comparative study and analysis of different segmentation, feature extraction and classification methods used for the automatic detection of DR. The fundus images from the Kaggle data set will be used to evaluate these techniques. The best results were obtained when Watershed Transform (WT) and Triplet Half Band Filter Bank (THFB) based segmentation and Haralick, and ADTCWT (Anisotropic Dual Tree Complex Wavelet Transform) based feature extraction together with machine learning based SVM (Support Vector Machine) classifier. The performance of the classifiers was evaluated in terms of accuracy, precision, F-Score, TPR (True Positive Rate), TNR (True Negative Rate), Kappa, FPR (False Positive Rate), FNR (False Negative rate), pixel accuracy, Jaccard similarity, cube coefficient, VOE (volumetric overlap error) and SVD (symmetric volume difference). The SVM model obtained a training accuracy of (98.42%).
更多查看译文
关键词
Diabetic retinopathy detection,Machine learning (ML),Haralick features,Wavelet transforms
AI 理解论文
溯源树
样例
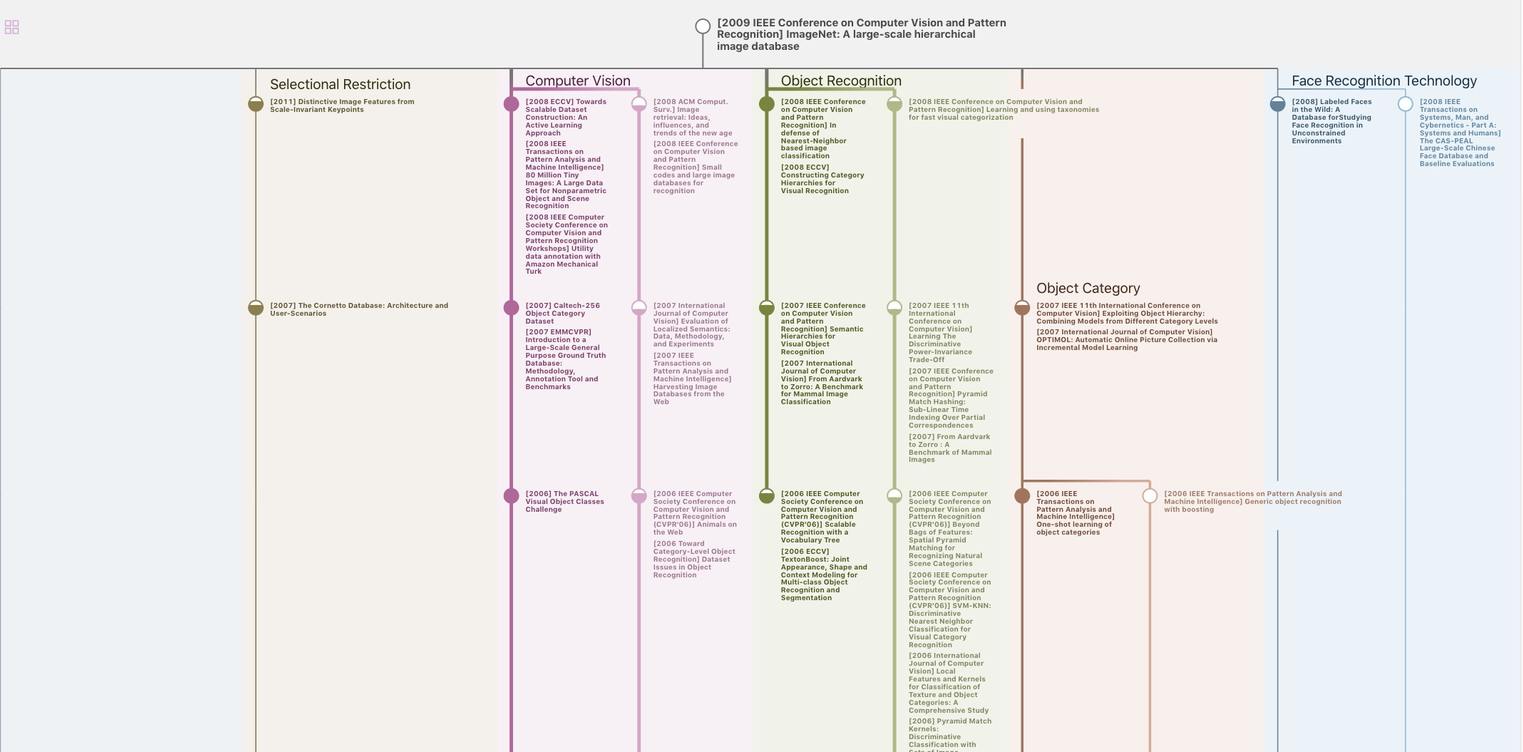
生成溯源树,研究论文发展脉络
Chat Paper
正在生成论文摘要