MLSE-Net: Multi-level Semantic Enriched Network for Medical Image Segmentation
KSII TRANSACTIONS ON INTERNET AND INFORMATION SYSTEMS(2023)
摘要
Medical image segmentation techniques based on convolution neural networks indulge in feature extraction triggering redundancy of parameters and unsatisfactory target localization, which outcomes in less accurate segmentation results to assist doctors in diagnosis. In this paper, we propose a multi-level semantic-rich encoding-decoding network, which consists of a Pooling-Conv-Former (PCFormer) module and a Cbam-Dilated-Transformer (CDT) module. In the PCFormer module, it is used to tackle the issue of parameter explosion in the conservative transformer and to compensate for the feature loss in the down-sampling process. In the CDT module, the Cbam attention module is adopted to highlight the feature regions by blending the intersection of attention mechanisms implicitly, and the Dilated convolution-Concat (DCC) module is designed as a parallel concatenation of multiple atrous convolution blocks to display the expanded perceptual field explicitly. In addition, MultiHead Attention-DwConv-Transformer (MDTransformer) module is utilized to evidently distinguish the target region from the background region. Extensive experiments on medical image segmentation from Glas, SIIM-ACR, ISIC and LGG demonstrated that our proposed network outperforms existing advanced methods in terms of both objective evaluation and subjective visual performance.
更多查看译文
关键词
Transformer, Attention mechanism, Semantic enriched, Medical image segmentation
AI 理解论文
溯源树
样例
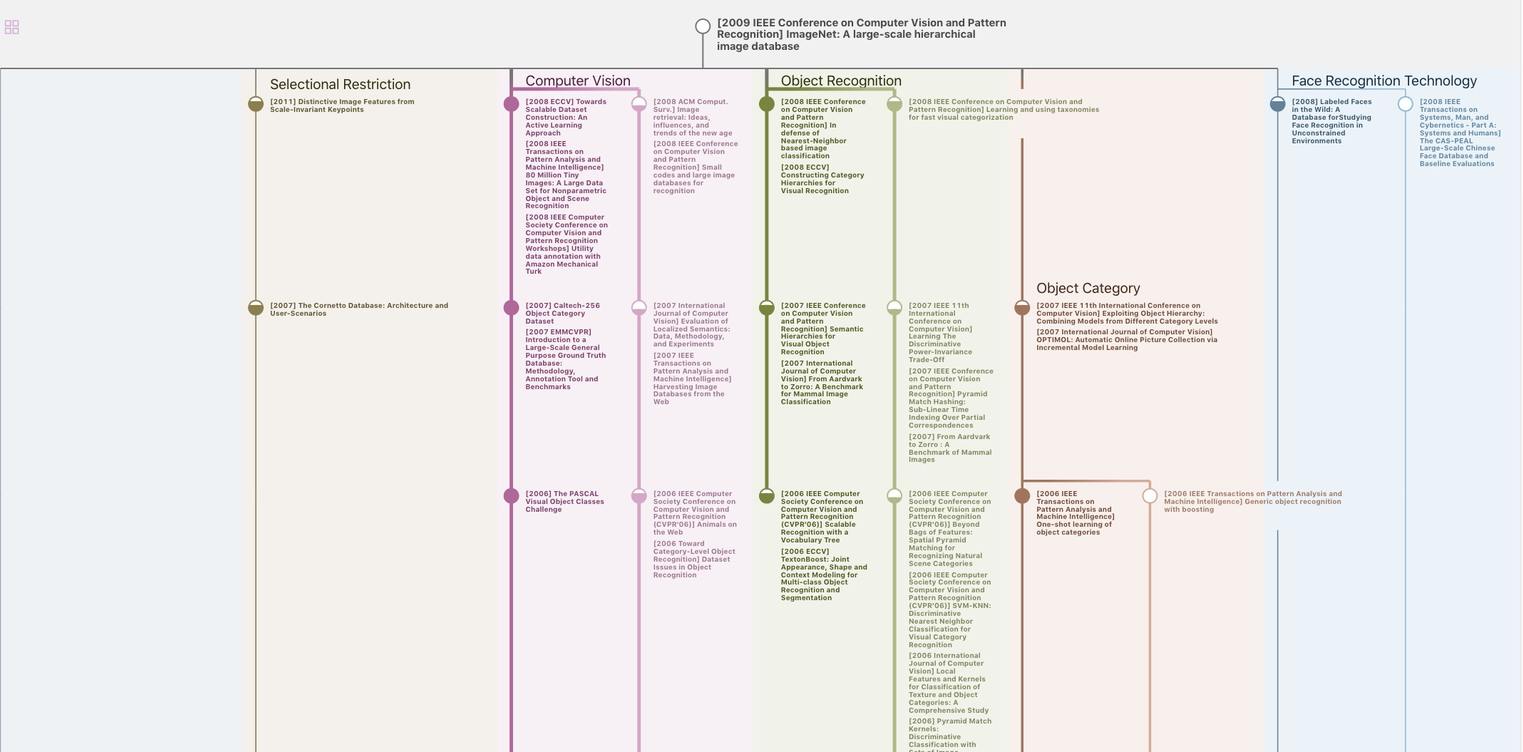
生成溯源树,研究论文发展脉络
Chat Paper
正在生成论文摘要