An adaptively weighted loss-enabled lightweight teacher–student model for real-time railroad inspection on edge devices
NEURAL COMPUTING & APPLICATIONS(2023)
摘要
Railroad inspections to identify missing track components are crucial to railroad operational safety. This paper presents a new lightweight computer vision model on edge devices for accurate, real-time rail track inspection. It modifies the teacher–student guidance mechanism in NanoDet ( https://github.com/RangiLyu/nanodet ) by introducing a new adaptively weighted loss (AWL) to the training process. The AWL evaluates the teacher and student model qualities, determines the weight of the student loss, and then balances their loss contributions on-the-fly, gearing the training process toward proper knowledge distillation and guidance. Compared to SOTA models, our AWL-NanoDet features a tiny model size of less than 10 MB and a computation cost of 1.52 G FLOPs and achieves an processing time of less than 14 ms per frame when tested on Nvidia’s AGX Orin. Relative to native NanoDet, it also notably improves the model’s performance by nearly 10%, enabling highly accurate, real-time detection of track components.
更多查看译文
关键词
Computer vision,Object detection,Lightweight model,Railroad inspection
AI 理解论文
溯源树
样例
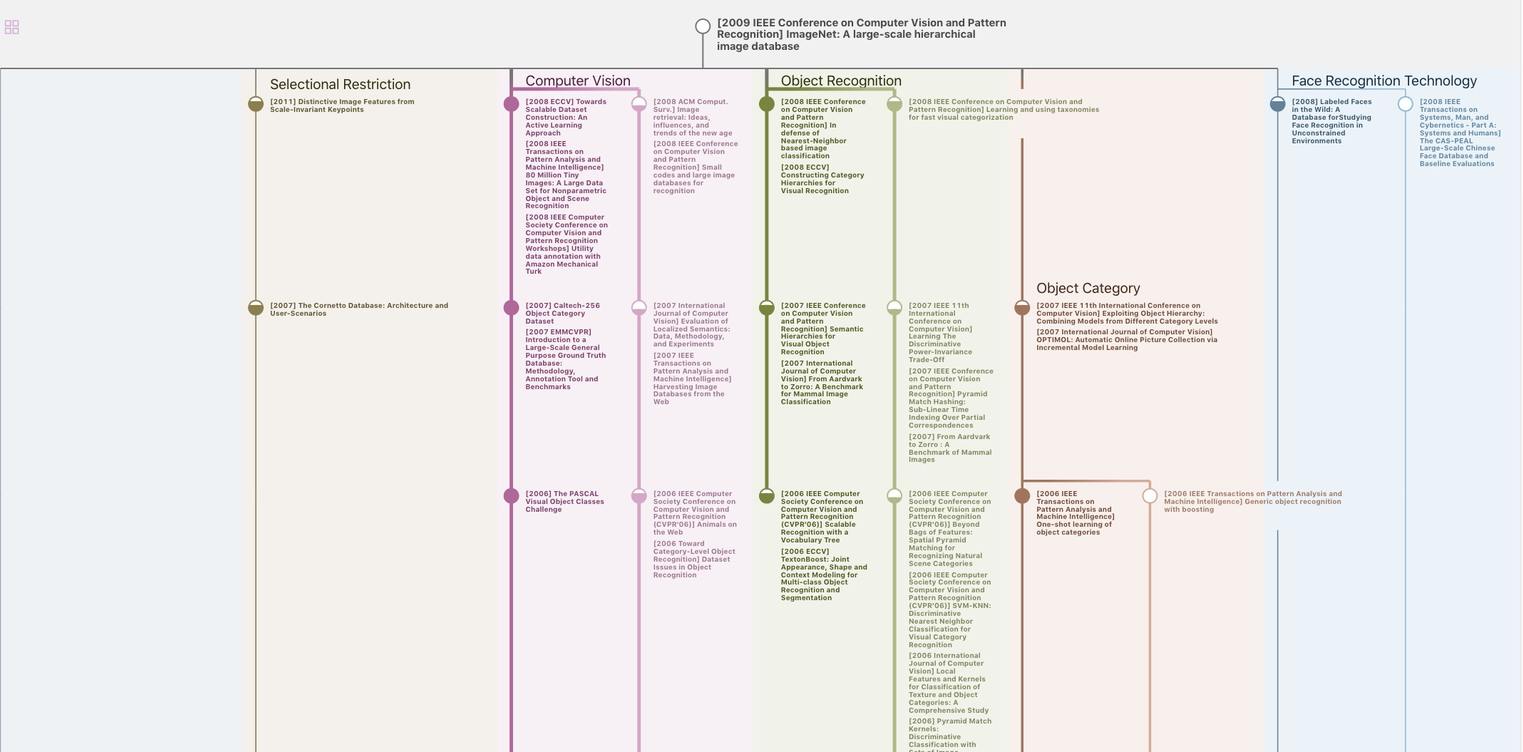
生成溯源树,研究论文发展脉络
Chat Paper
正在生成论文摘要