ViT-PGC: vision transformer for pedestrian gender classification on small-size dataset
PATTERN ANALYSIS AND APPLICATIONS(2023)
摘要
Pedestrian gender classification (PGC) is a key task in full-body-based pedestrian image analysis and has become an important area in applications like content-based image retrieval, visual surveillance, smart city, and demographic collection. In the last decade, convolutional neural networks (CNN) have appeared with great potential and with reliable choices for vision tasks, such as object classification, recognition, detection, etc. But CNN has a limited local receptive field that prevents them from learning information about the global context. In contrast, a vision transformer (ViT) is a better alternative to CNN because it utilizes a self-attention mechanism to attend to a different patch of an input image. In this work, generic and effective modules such as locality self-attention (LSA), and shifted patch tokenization (SPT)-based vision transformer model are explored for the PGC task. With the use of these modules in ViT, it is successfully able to learn from stretch even on small-size (SS) datasets and overcome the lack of locality inductive bias. Through extensive experimentation, we found that the proposed ViT model produced better results in terms of overall and mean accuracies. The better results confirm that ViT outperformed state-of-the-art (SOTA) PGC methods.
更多查看译文
关键词
Vision transformer, LSA and SPT, Deep CNN models, SS datasets, Pedestrian gender classification
AI 理解论文
溯源树
样例
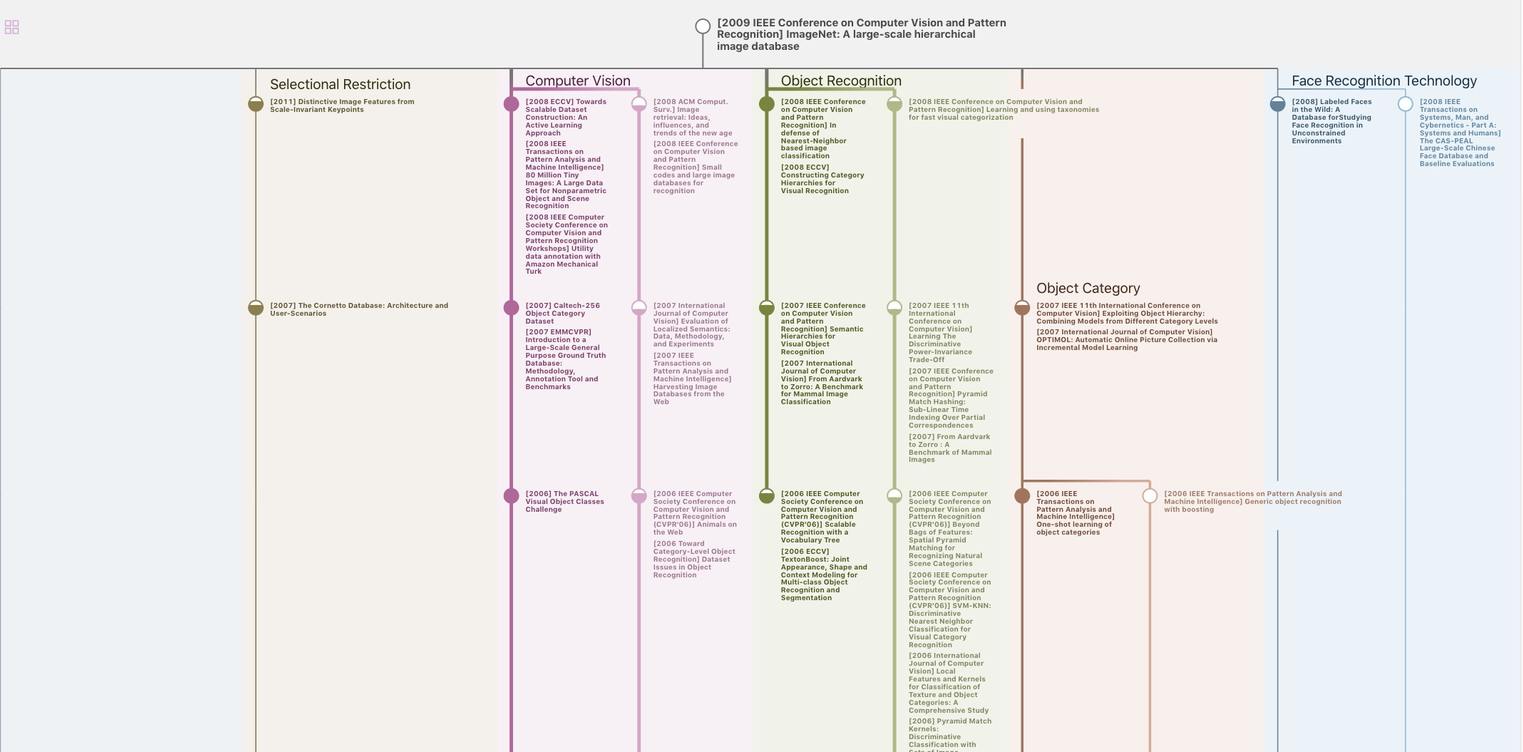
生成溯源树,研究论文发展脉络
Chat Paper
正在生成论文摘要