An efficient confusing choices decoupling framework for multi-choice tasks over texts
NEURAL COMPUTING & APPLICATIONS(2024)
摘要
This paper focuses on the multi-choice tasks, which aim to select the correct choice for a given query by reasoning over texts, such as sentences and passages. Benefiting from the provided knowledge in these tasks, the reasoning of multi-choice models becomes well-founded. However, besides the evidence of the correct choice, the text usually contains some content related to other choices as well, which causes multi-choice models vulnerable to being confused by candidate choices, and we denote it as choice confusion problem . To alleviate this challenge, we propose two auxiliary mechanisms to distinguish the confusing choices. Specifically, a q uery- g uided a ttention (QGA) mechanism is designed to automatically filter the text contents causing confusion by measuring the syntactic relevance between different contents and the query. Meanwhile, a c onfusion- a ware t raining (CAT) mechanism is designed to learn the correct and easily confused choices asynchronously and perform a pushing-away operation between their selection processes. We conduct experiments on multi-choice tasks based on sentences and passages. The results show that our framework improves the choice selection accuracy compared to strong baselines. Furthermore, the ablation test and case study verify the effectiveness of our proposed QGA and CAT, especially in addressing the choice confusion problem.
更多查看译文
关键词
efficient confusing choices,decoupling,texts,multi-choice
AI 理解论文
溯源树
样例
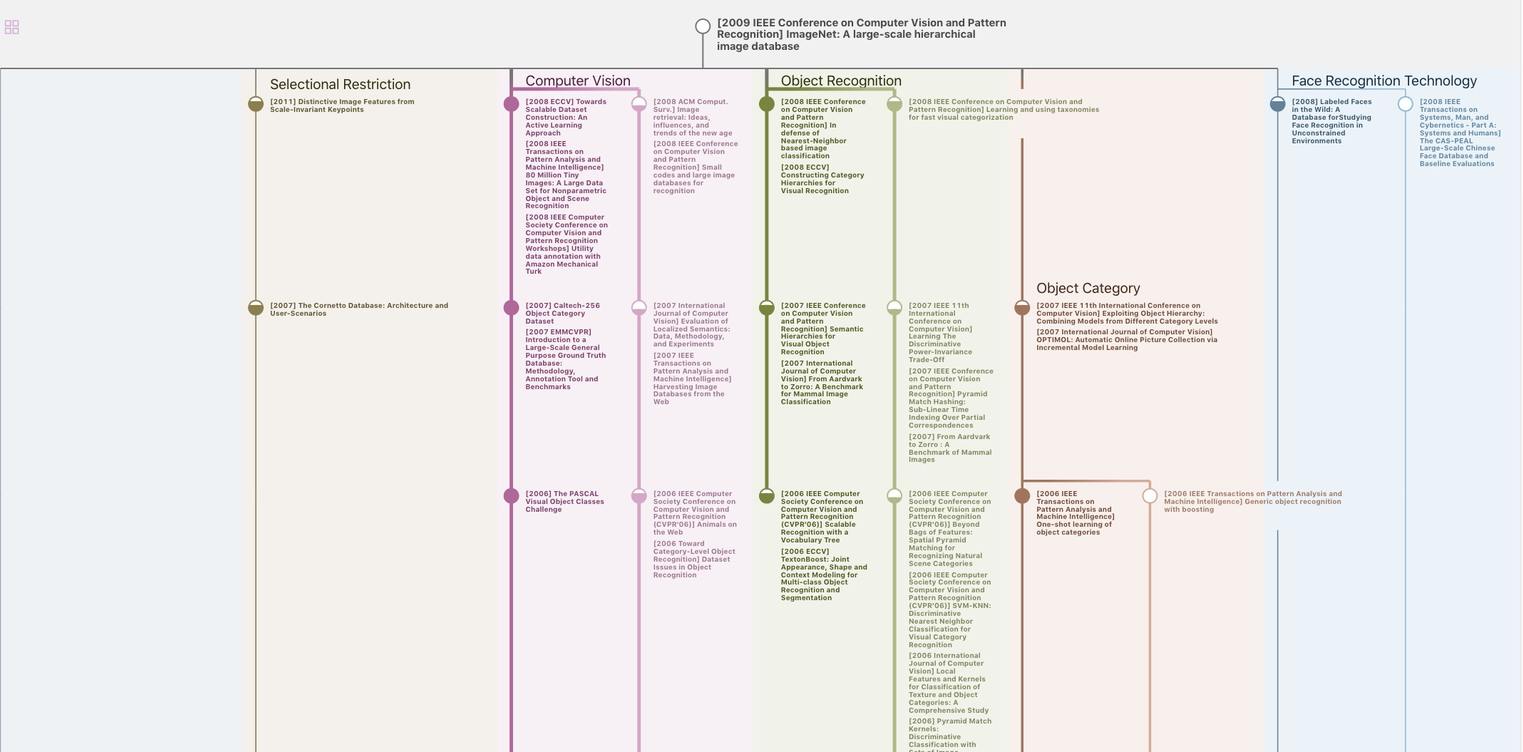
生成溯源树,研究论文发展脉络
Chat Paper
正在生成论文摘要