Early qualitative and quantitative amplitude-integrated electroencephalogram and raw electroencephalogram for predicting long-term neurodevelopmental outcomes in extremely preterm infants in the Netherlands: a 10-year cohort study.
The Lancet. Digital health(2023)
摘要
BACKGROUND:Extremely preterm infants (<28 weeks of gestation) are at great risk of long-term neurodevelopmental impairments. Early amplitude-integrated electroencephalogram (aEEG) accompanied by raw EEG traces (aEEG-EEG) has potential for predicting subsequent outcomes in preterm infants. We aimed to determine whether and which qualitative and quantitative aEEG-EEG features obtained within the first postnatal days predict neurodevelopmental outcomes in extremely preterm infants.
METHODS:This study retrospectively analysed a cohort of extremely preterm infants (born before 28 weeks and 0 days of gestation) who underwent continuous two-channel aEEG-EEG monitoring during their first 3 postnatal days at Wilhelmina Children's Hospital, Utrecht, the Netherlands, between June 1, 2008, and Sept 30, 2018. Only infants who did not have genetic or metabolic diseases or major congenital malformations were eligible for inclusion. Features were extracted from preprocessed aEEG-EEG signals, comprising qualitative parameters grouped in three types (background pattern, sleep-wake cycling, and seizure activity) and quantitative metrics grouped in four categories (spectral content, amplitude, connectivity, and discontinuity). Machine learning-based regression and classification models were used to evaluate the predictive value of the extracted aEEG-EEG features for 13 outcomes, including cognitive, motor, and behavioural problem outcomes, at 2-3 years and 5-7 years. Potential confounders (gestational age at birth, maternal education, illness severity, morphine cumulative dose, the presence of severe brain injury, and the administration of antiseizure, sedative, or anaesthetic medications) were controlled for in all prediction analyses.
FINDINGS:369 infants were included and an extensive set of 339 aEEG-EEG features was extracted, comprising nine qualitative parameters and 330 quantitative metrics. The machine learning-based regression models showed significant but relatively weak predictive performance (ranging from r=0·13 to r=0·23) for nine of 13 outcomes. However, the machine learning-based classifiers exhibited acceptable performance in identifying infants with intellectual impairments from those with optimal outcomes at age 5-7 years, achieving balanced accuracies of 0·77 (95% CI 0·62-0·90; p=0·0020) for full-scale intelligence quotient score and 0·81 (0·65-0·96; p=0·0010) for verbal intelligence quotient score. Both classifiers maintained identical performance when solely using quantitative features, achieving balanced accuracies of 0·77 (95% CI 0·63-0·91; p=0·0030) for full-scale intelligence quotient score and 0·81 (0·65-0·96; p=0·0010) for verbal intelligence quotient score.
INTERPRETATION:These findings highlight the potential benefits of using early postnatal aEEG-EEG features to automatically recognise extremely preterm infants with poor outcomes, facilitating the development of an interpretable prognostic tool that aids in decision making and therapy planning.
FUNDING:European Commission Horizon 2020.
更多查看译文
AI 理解论文
溯源树
样例
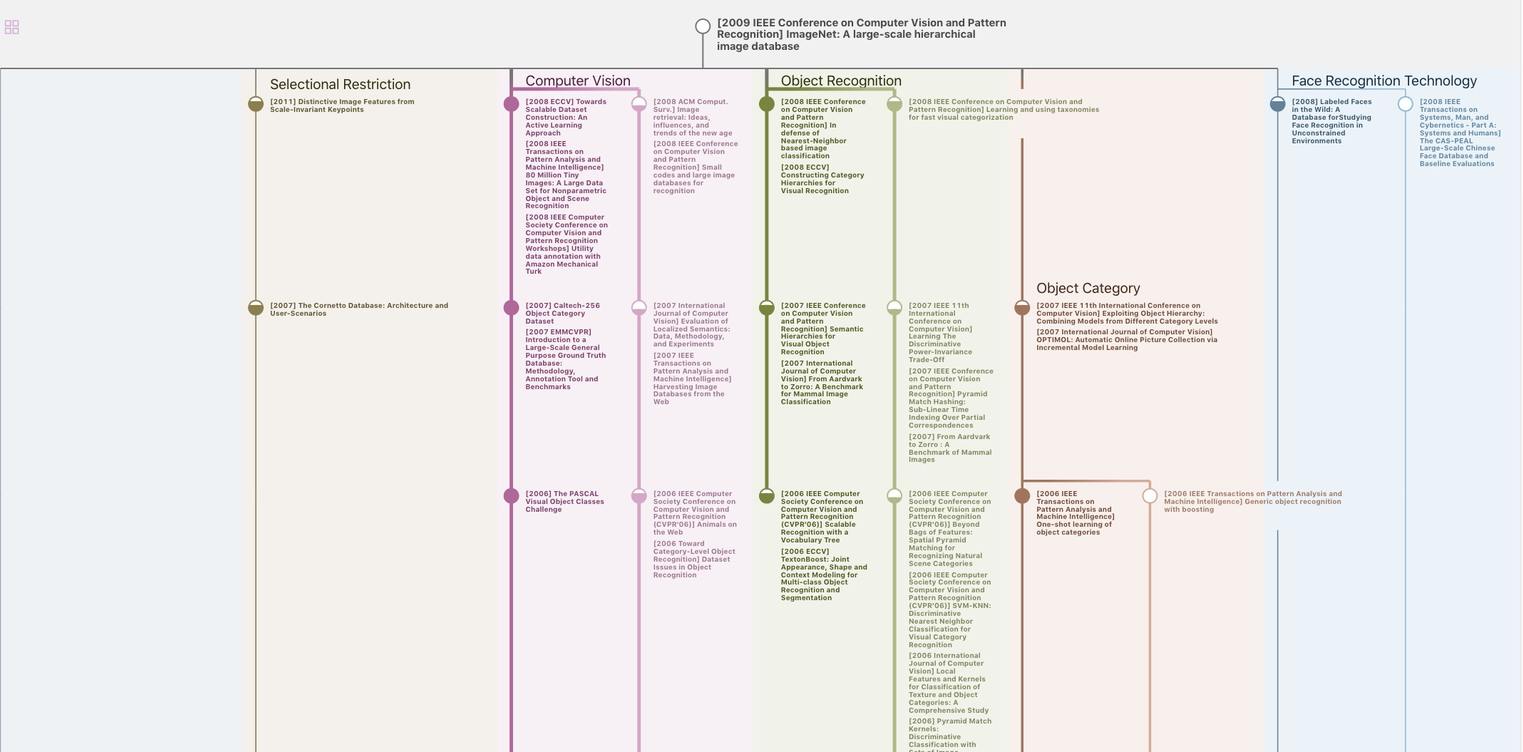
生成溯源树,研究论文发展脉络
Chat Paper
正在生成论文摘要