Parameter-Efficient Transfer Learning for Medical Visual Question Answering
IEEE TRANSACTIONS ON EMERGING TOPICS IN COMPUTATIONAL INTELLIGENCE(2023)
摘要
The Contrastive Language-Image Pre-Training (CLIP) model, pretrained on large visual text corpora, has demonstrated significant improvements in visual and linguistic tasks and has been applied to various downstream tasks. At least two issues, however, hinder the transfer learning with such powerful pretrained models in the field of medical visual question answering (Med-VQA). That current methods tend to full fine-tune these large-scale models are suffering from increasingly expensive computational cost as the model size grows in development. Additionally, published Med-VQA datasets are small that may lead to overfitting when directly fine-tuning on them. In this article, we integrate two designs and propose an efficient transfer learning method for Med-VQA named VQA-Adapter. To alleviate training costs, we introduce a novel and parameter-efficient adapter component into Med-VQA. During training, only the proposed light-weight adapter needs to be tuned, while all parameters in the large-scale visual model of CLIP could be kept frozen. We further design a multi-stage label smoothing paradigm for Med-VQA to deal with the overfitting issue in small Med-VQA datasets. Experimental results on two popular Med-VQA datasets, i.e., VQA-RAD and SLAKE, demonstrate that our method can significantly outperform existing state-of-the-art methods on both open-ended and closed-ended question answering tasks. Furthermore, compared to directly fine-tuning the entire CLIP model, our approach only requires to update 2.38% of the parameters. Extensive ablation studies, analysis and visualizations convincingly demonstrate the great potential of designing light-weight frameworks to transfer large-scale pretrained models from natural vision-language tasks to domain-specific medical applications.
更多查看译文
关键词
visual,learning,transfer,parameter-efficient
AI 理解论文
溯源树
样例
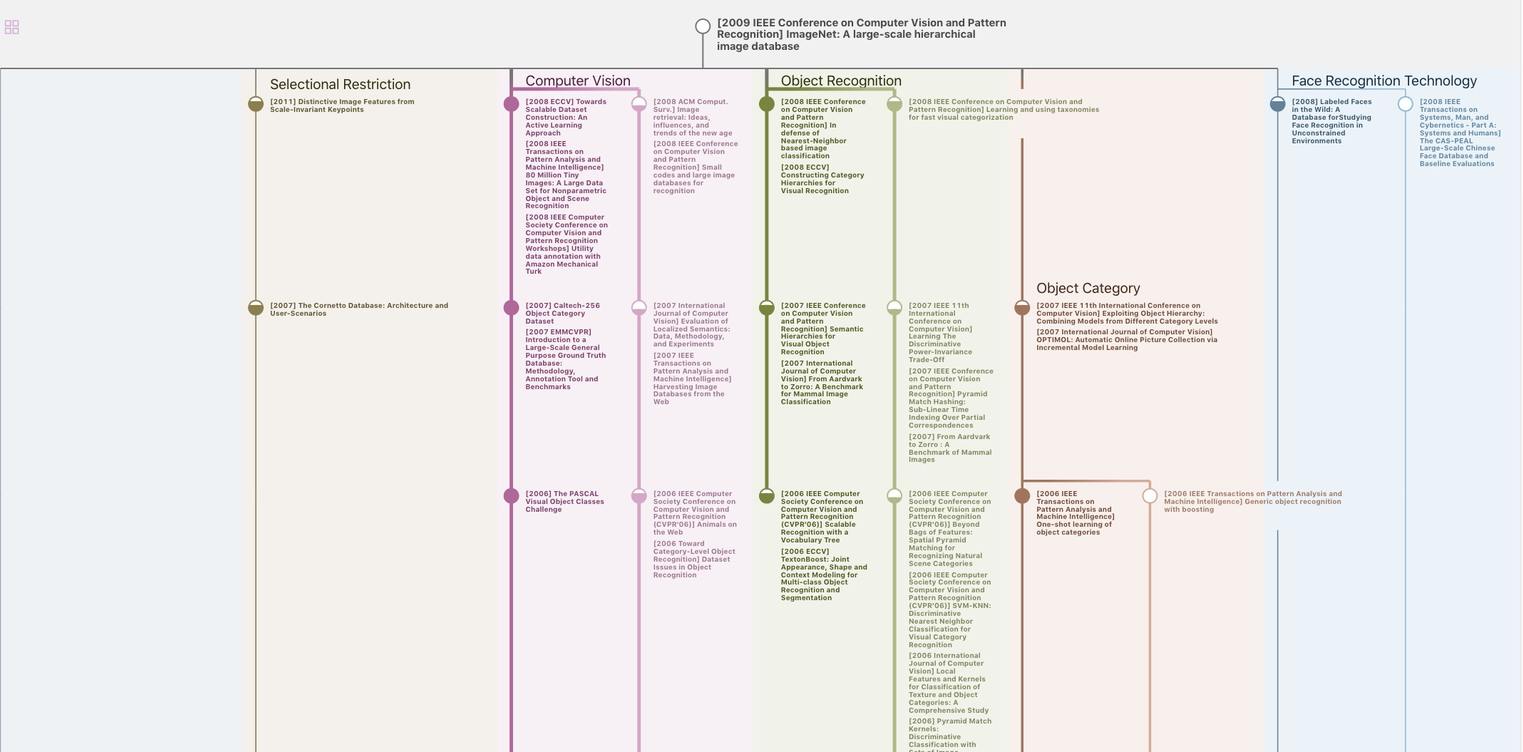
生成溯源树,研究论文发展脉络
Chat Paper
正在生成论文摘要