A domain knowledge enhanced machine learning method to predict the properties of halide double perovskite A2B+B3+X6
JOURNAL OF MATERIALS CHEMISTRY A(2023)
摘要
The heat of formation and band gap are important properties of halide double perovskites, which can dictate the range of application. However, traditional laborious experiments and density functional theory (DFT) calculations are time-consuming, and machine learning methods perform poorly in acquiring hidden knowledge from material samples, which make it difficult to explore promising perovskites with high stability efficiently. Here, we present a new machine learning framework, which could embed material domain knowledge into a machine learning method, and then it is used to predict the heat of formation and band gap. Compared with traditional machine learning methods, we found that the prediction accuracy of the heat of formation and band gap in 540 perovskite materials A(2)B(+)B(3+)X(6) is improved by 5.65% and 15.52% (namely 93.58% and 62.65%) respectively. It is shown that material domain knowledge contains a wealth of logical rules and expert experience, which can remedy most of the problems in data-driven materials research, such as data sparse and complex relationships between features.
更多查看译文
关键词
domain knowledge,machine learning,halide
AI 理解论文
溯源树
样例
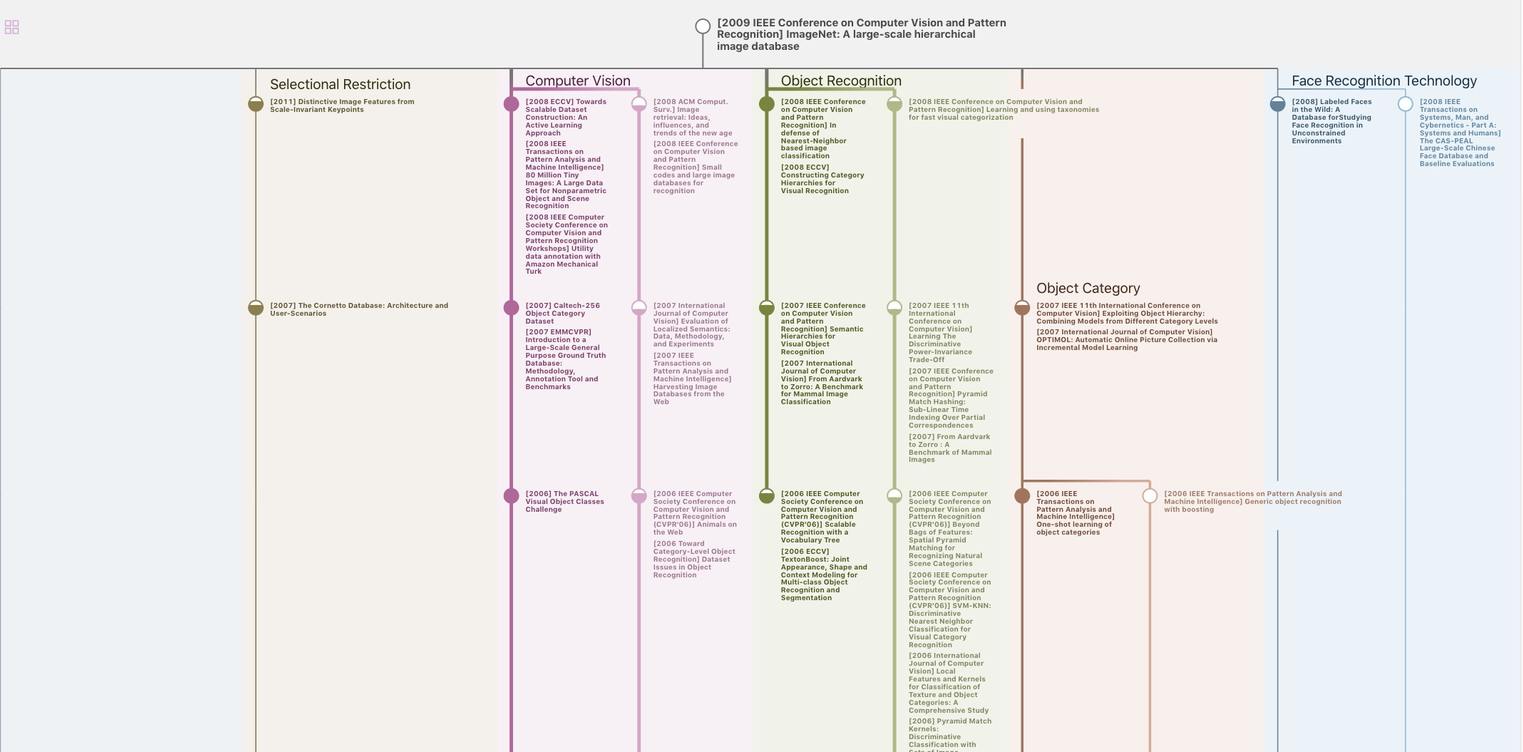
生成溯源树,研究论文发展脉络
Chat Paper
正在生成论文摘要