In Reply: Deep Neural Networks Can Accurately Detect Blood Loss and Hemorrhage Control Task Success from Video
NEUROSURGERY(2023)
摘要
To the Editor: We appreciate the opportunity to respond to the Letter to the Editor from Dr Lim1 on our recent article titled “Deep Neural Networks Can Accurately Detect Blood Loss and Hemorrhage Control Task Success From Video.”2 In this study, we developed deep learning models to predict outcomes from cadaveric training exercises of surgeons controlling simulated internal carotid hemorrhage, finding that models using a combination of video and tool presence in frame outperformed models using video alone. We thank Dr Lim for engaging with our work. Our dialogue highlights the urgent need for deep engagement between scientists and surgeons to create, disseminate, and analyze data collected during the surgical episode and then to subsequently bring these analyses to bear on the performance of surgery. In this letter to the editor, Dr Lim highlights the use of machine learning–based time series data analysis and its potential applications to Neurosurgery. We agree with Dr Lim's observations and believe that there are currently many opportunities for these types of analytical tools to be used in Neurosurgery. For the analysis of surgical video, other surgical disciplines have demonstrated that neural networks leveraging time series models can be used to predict surgical phases in cholecystectomy,3 sleeve gastrectomy,4 and peroral endoscopic myotomy5 and to identify surgical actions and provide feedback in robotic procedures in urology.6-8 Within Neurosurgery, our group demonstrated that neural networks could reliably detect surgical instruments in view in a cadaveric training exercise and that these results translated to real intraoperative video.9,10 The use of time series deep learning approaches extends to efforts outside of the analysis of surgical video, such as measuring intracranial pressure.11,12 We wholeheartedly concur with Dr Lim's view that, despite the widespread availability of modeling approaches, exploiting intraoperative time series data remains an undiscovered scientific frontier with deep clinical applications. Overcoming the dearth of public, high-quality, and clinically relevant data sets and the clinically relevant common task frameworks to encourage scientific progress is a major emphasis of our current work. In our field of surgical video analysis, managing high volumes of video data and altering the surgical workflow required to collect video are major obstacles. In addition, there are often concerns around patient privacy and data sharing that can limit access to large data sets. For the analysis of surgical video, several groups have created publicly available data sets and the EndoVis challenge has a consistent call for the submission of additional data sets.13 Within Neurosurgery, our group has released 2 video datasets, the Simulated Outcomes after Carotid Artery Laceration9 and Simulated Outcomes for Durotomy Repair in Minimally Invasive Spine Surgery (SOSpine, submitted for review) and made them easily publicly available online to encourage the development of improved models for surgical video analysis. In sharing our own data sets, we hope to continue to cultivate an interest in applying machine learning to Neurosurgery and that it encourages other groups engaged in these projects to produce and publish their own data sets in the future. Dr Lim highlighted the importance of explainable artificial intelligence (AI) and the recent interest in the development of models that allow users to understand the most salient input data affecting a model's prediction. The recent development and publication of ChatGPT14 have increased public excitement about explainable AI since the core ChatGPT model has very limited explainability. In the work at the center of this letter, we found that including information about tool presence along with the surgical frames led to an improvement in model performance on task success and blood loss prediction. This finding suggested that adding features based on surgeons' clinical experience can lead to better performing models, while helping surgeons have greater confidence that the developed models are picking up on relevant clinical features rather than noise within the video stream. Within image-based deep learning analysis, several tools such as Grad-CAM15 and Smooth Grad-CAM++16 aim to create visual representations of where a deep learning model is “looking” in an image when making a prediction through the use of heatmaps of features contributing to predictions. Within time series machine learning analysis, the attention layer of transformers has frequently been used as an “interpretable” layer to gain an understanding of which timesteps a model may be using to make its predictions.17,18 Nevertheless, we agree with Dr Lim that explainable AI is crucial for the widespread use of these methods within clinical practice and that it should remain a priority in current AI research efforts. In conclusion, we thank Dr Lim for continuing the dialogue of our work and its role in the larger effort to apply machine learning methods to Neurosurgery. We hope that by emphasizing the encouraging results of our study, outlining the need for publicly available data sets in Neurosurgery, and describing a proposed emphasis on explainable AI methods, other groups will be motivated to join this exciting field of research. We are issuing a call for large, cross-disciplinary collaboration to our major neurosurgical societies such as the Congress, and we are actively building the research infrastructure to support this work through a new research collective.
更多查看译文
关键词
hemorrhage control task success,blood loss,deep neural networks,neural networks
AI 理解论文
溯源树
样例
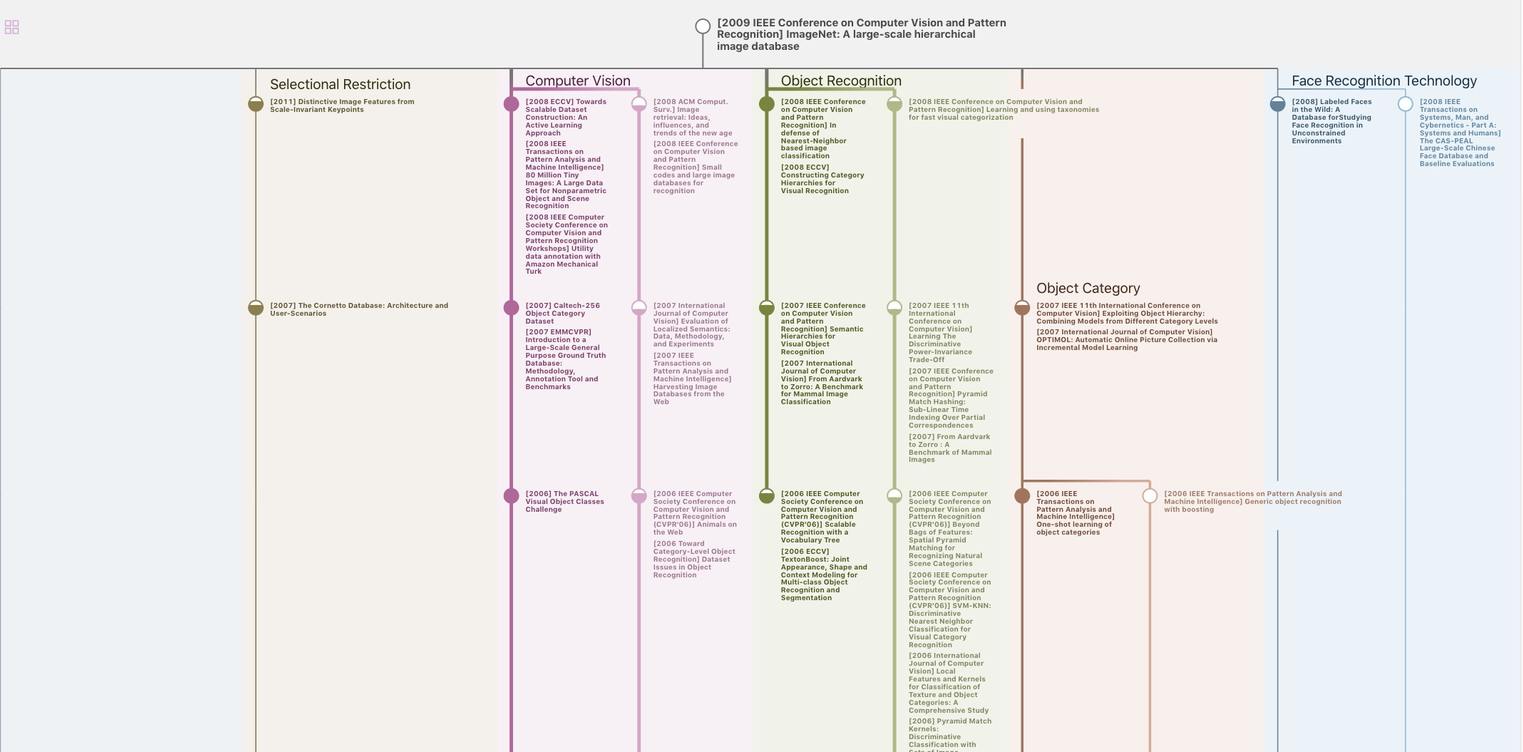
生成溯源树,研究论文发展脉络
Chat Paper
正在生成论文摘要