Assessing Critical Data Types for Deep Learning-based PV Generation Forecasting
2023 IEEE BELGRADE POWERTECH(2023)
摘要
Solar power generation is playing an increasingly important role for sustainable power systems. Reliable forecasting of solar power generation is one of the fundamental challenges faced by network operators nowadays, especially under drastic weather changes. In this paper, two artificial neural network models, Long Short-Term Memory (LSTM) network and Gated Recurrent Unit (GRU) network, are used to predict solar power generation day ahead, and the hyperparameters in the neural network are optimized by the Gaussian process. Considering various input variables affecting the forecast, such as active power, wind speed, temperature, humidity, global horizontal radiation, etc., the dominant factors for solar power generation forecast are analysed. To identify the critical data types for higher prediction accuracy, three models are designed to compare the influence of different levels of use of day ahead weather data on solar power forecasting, and the testing is carried out for the model input of 12 months, 6 months and 1 month.
更多查看译文
关键词
PV generation,short-term forecasting,artificial neural network,deep learning,Gaussian process
AI 理解论文
溯源树
样例
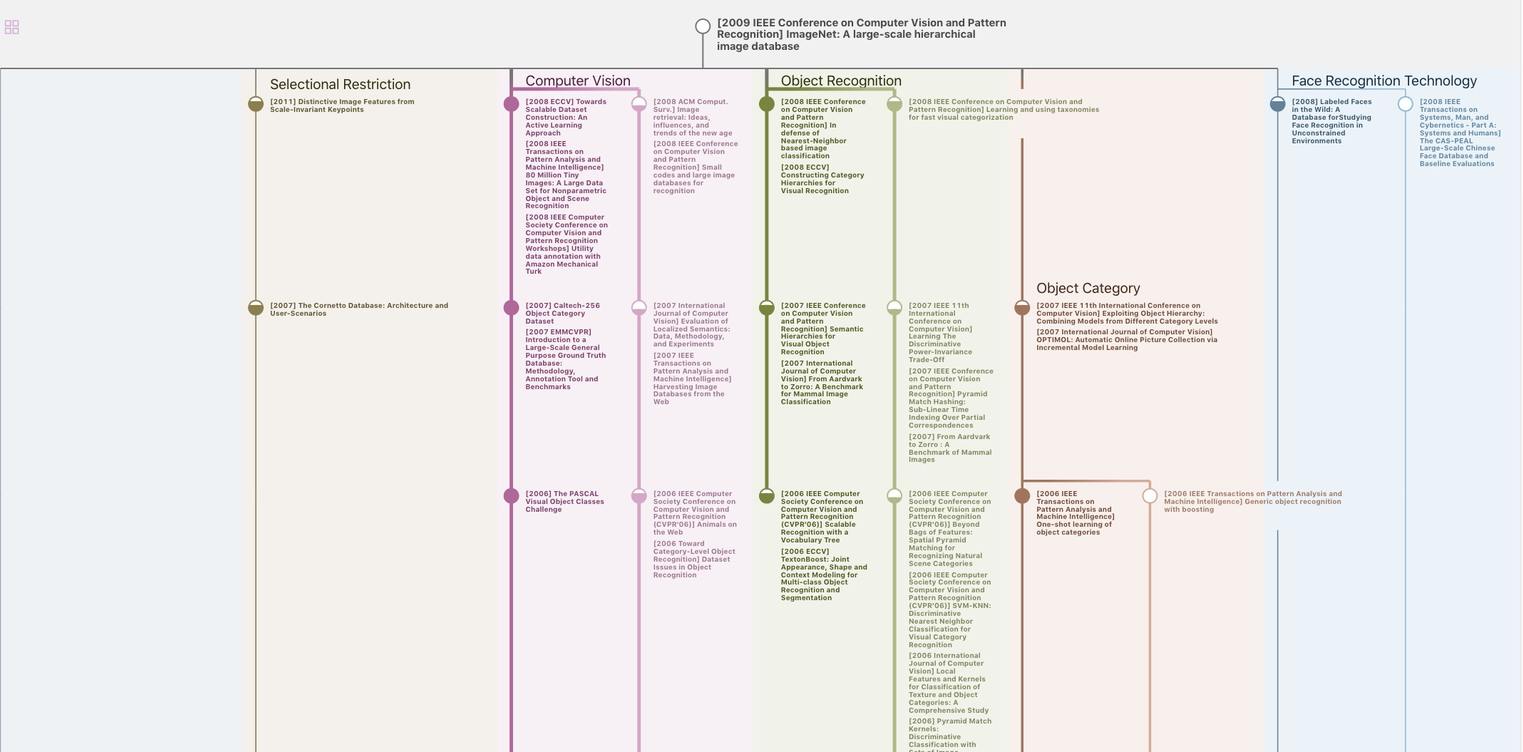
生成溯源树,研究论文发展脉络
Chat Paper
正在生成论文摘要