TransCropNet: a multichannel transformer with feature-level fusion for crop classification in agricultural smallholdings using Sentinel images
JOURNAL OF APPLIED REMOTE SENSING(2023)
摘要
Crop classification in small and fragmented agricultural lands is a challenging task. The presence of cloud cover poses additional challenges when classical techniques using multispectral images are applied. Multispectral data augmented with SAR images is capable of solving this issue. The use of robust deep neural network classifiers can further improve the classification performance. We propose a multichannel attention-based deep learning model to fuse time-series SAR and a single scene multispectral data at the feature level and classify tropical crops grown in smallholdings at pixel level with high accuracy. The attention-based transformer neural network for the fusion of Sentinel-1 and Sentinel-2 data is evaluated for crop classification in the Cauvery delta zone. The model achieves an overall classification accuracy of 92.6% and a kappa score of 0.906. The source code is available in a GitHub repository at: https:// github.com/ajigeo/temporal-SAR-transformer. (c) 2023 Society of Photo-Optical Instrumentation Engineers (SPIE) [DOI: 10.1117/1.JRS.17.024501]
更多查看译文
关键词
transcropnet classification,multichannel transformer,sentinel images,agricultural smallholdings,feature-level
AI 理解论文
溯源树
样例
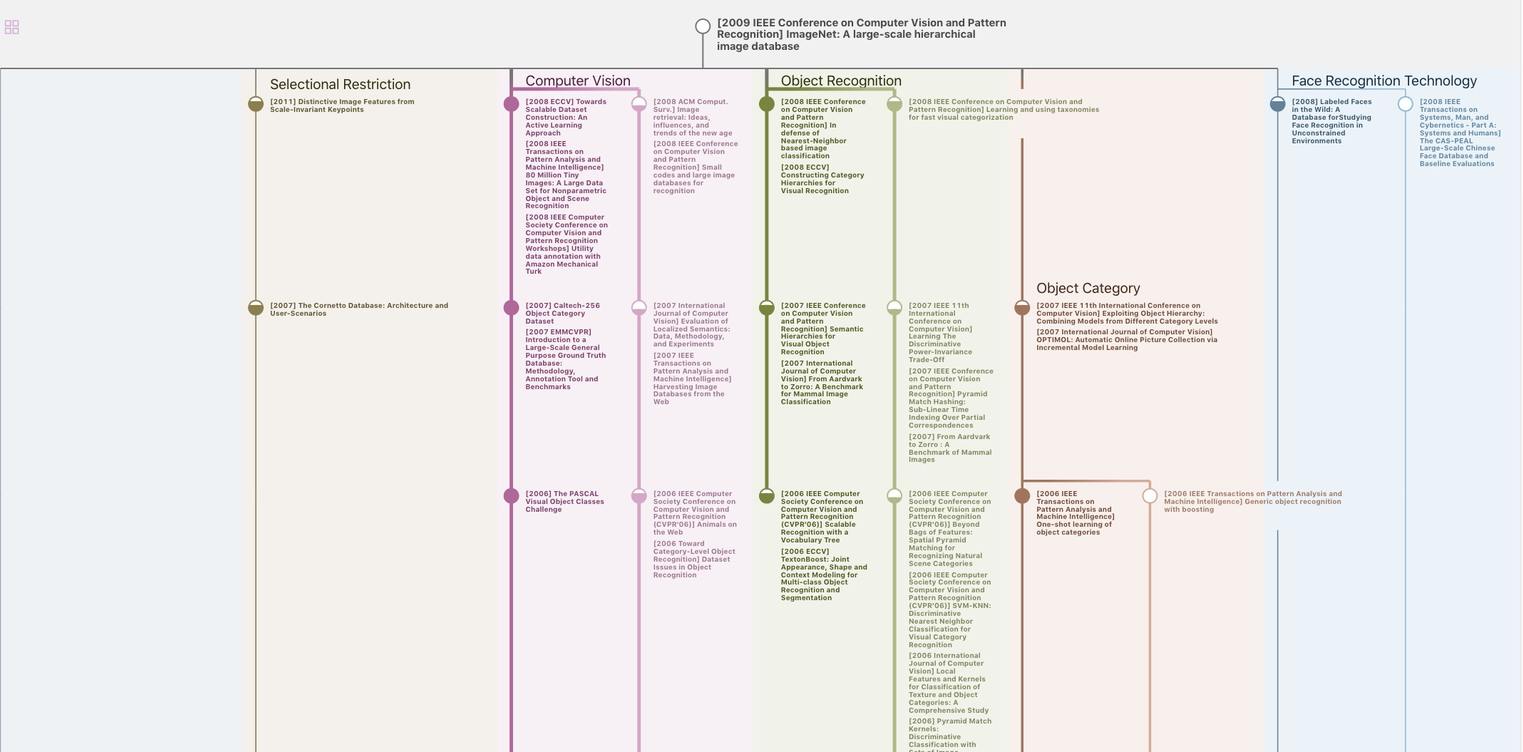
生成溯源树,研究论文发展脉络
Chat Paper
正在生成论文摘要