Efficient Diffraction Modeling Using Neural Networks and Infinite Impulse Response Filters
JOURNAL OF THE AUDIO ENGINEERING SOCIETY(2023)
摘要
Creating plausible geometric acoustic simulations in complex scenes requires the inclusion of diffraction modeling. Current real-time diffraction implementations use the Uniform Theory of Diffraction, which assumes all edges are infinitely long. The authors utilize recent advances in machine learning to create an efficient infinite impulse response model trained on data generated using the physically accurate Biot-Tolstoy-Medwin model. The authors propose an approach to data generation that allows their model to be applied to higher-order diffraction. They show that their model is able to approximate the Biot-Tolstoy-Medwin model with a mean absolute level difference of 1.0 dB for first-order diffraction while maintaining a higher computational efficiency than the current state of the art using the Uniform Theory of Diffraction.
更多查看译文
关键词
efficient diffraction modeling,infinite impulse response filters,neural networks
AI 理解论文
溯源树
样例
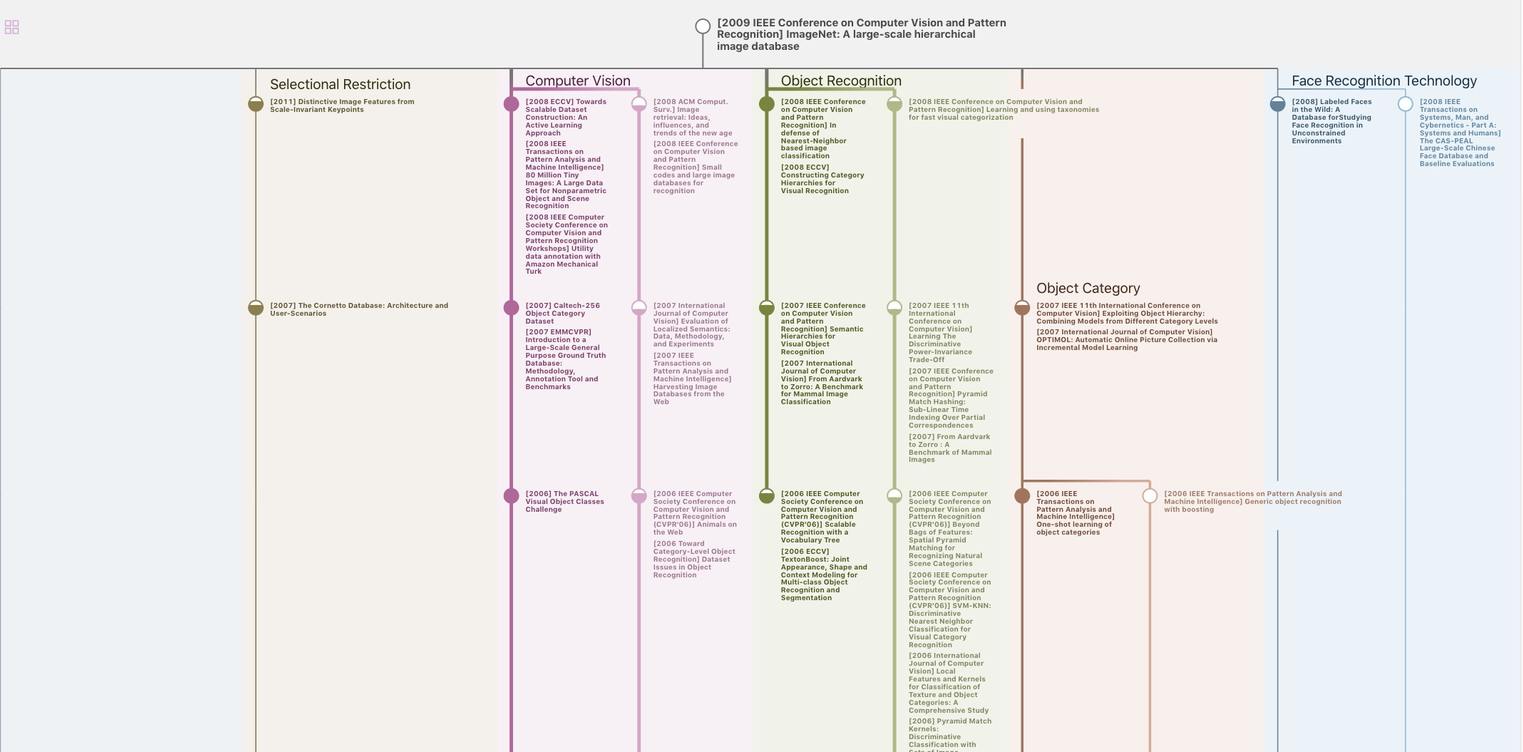
生成溯源树,研究论文发展脉络
Chat Paper
正在生成论文摘要