Reliable learning in challenging environments.
NeurIPS(2023)
摘要
The problem of designing learners that provide guarantees that their predictions are provably correct is of increasing importance in machine learning. However, learning theoretic guarantees have only been considered in very specific settings. In this work, we consider the design and analysis of reliable learners in challenging test-time environments as encountered in modern machine learning problems: namely adversarial test-time attacks (in several variations) and natural distribution shifts. In this work, we provide a reliable learner with provably optimal guarantees in such settings. We discuss computationally feasible implementations of the learner and further show that our algorithm achieves strong positive performance guarantees on several natural examples: for example, linear separators under log-concave distributions or smooth boundary classifiers under smooth probability distributions.
更多查看译文
关键词
distribution shift,learning,test-time
AI 理解论文
溯源树
样例
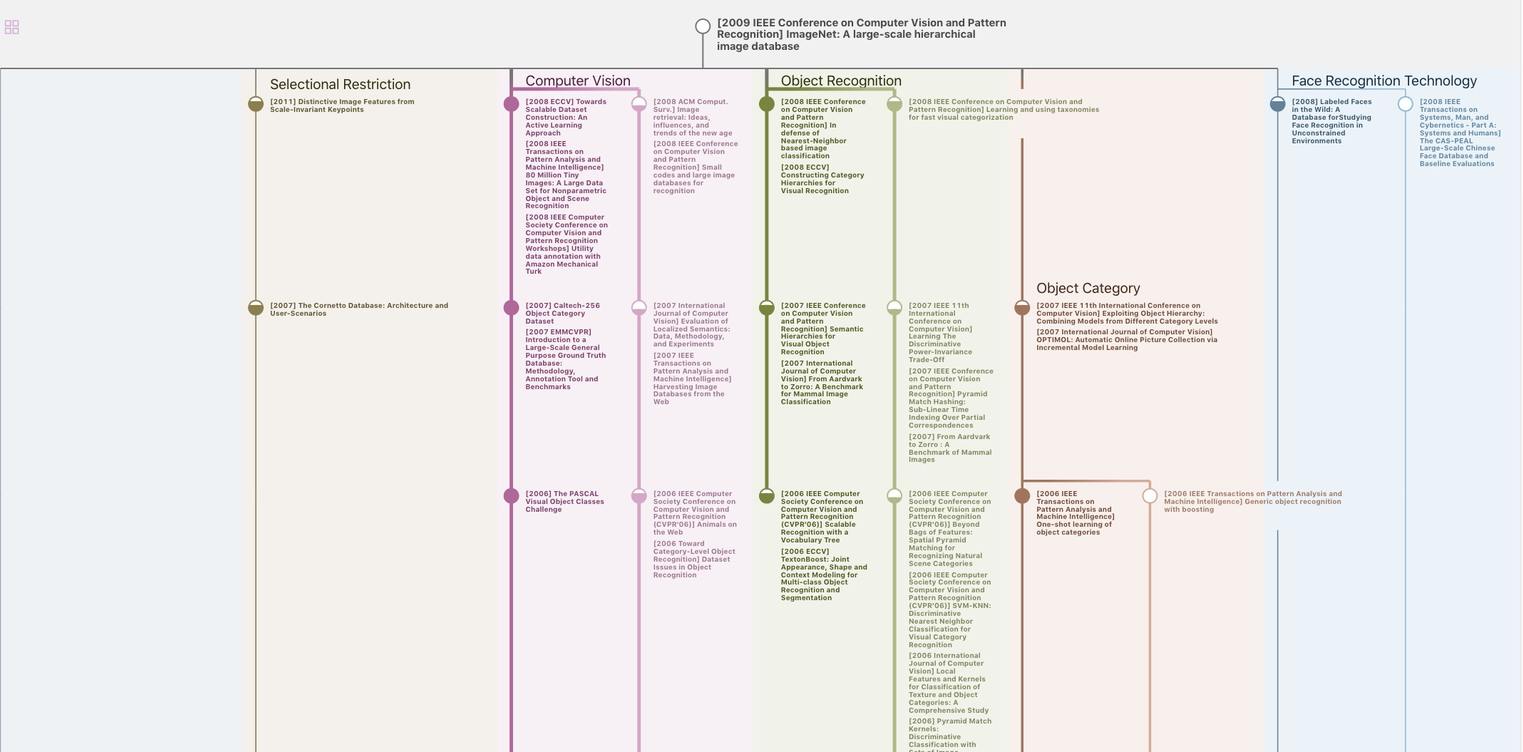
生成溯源树,研究论文发展脉络
Chat Paper
正在生成论文摘要