ConRad: Image Constrained Radiance Fields for 3D Generation from a Single Image
NeurIPS 2023(2023)
摘要
We present a novel method for reconstructing 3D objects from a single RGB image. Our method leverages the latest image generation models to infer the hidden 3D structure while remaining faithful to the input image. While existing methods obtain impressive results in generating 3D models from text prompts, they do not provide an easy approach for conditioning on input RGB data. Naive extensions of these methods often lead to improper alignment in appearance between the input image and the 3D reconstructions. We address these challenges by introducing Image Constrained Radiance Fields (ConRad), a novel variant of neural radiance fields. ConRad is an efficient 3D representation that explicitly captures the appearance of an input image in one viewpoint. We propose a training algorithm that leverages the single RGB image in conjunction with pretrained Diffusion Models to optimize the parameters of a ConRad representation. Extensive experiments show that ConRad representations can simplify preservation of image details while producing a realistic 3D reconstruction. Compared to existing state-of-the-art baselines, we show that our 3D reconstructions remain more faithful to the input and produce more consistent 3D models while demonstrating significantly improved quantitative performance on a ShapeNet object benchmark.
更多查看译文
关键词
image constrained radiance fields,3d generation
AI 理解论文
溯源树
样例
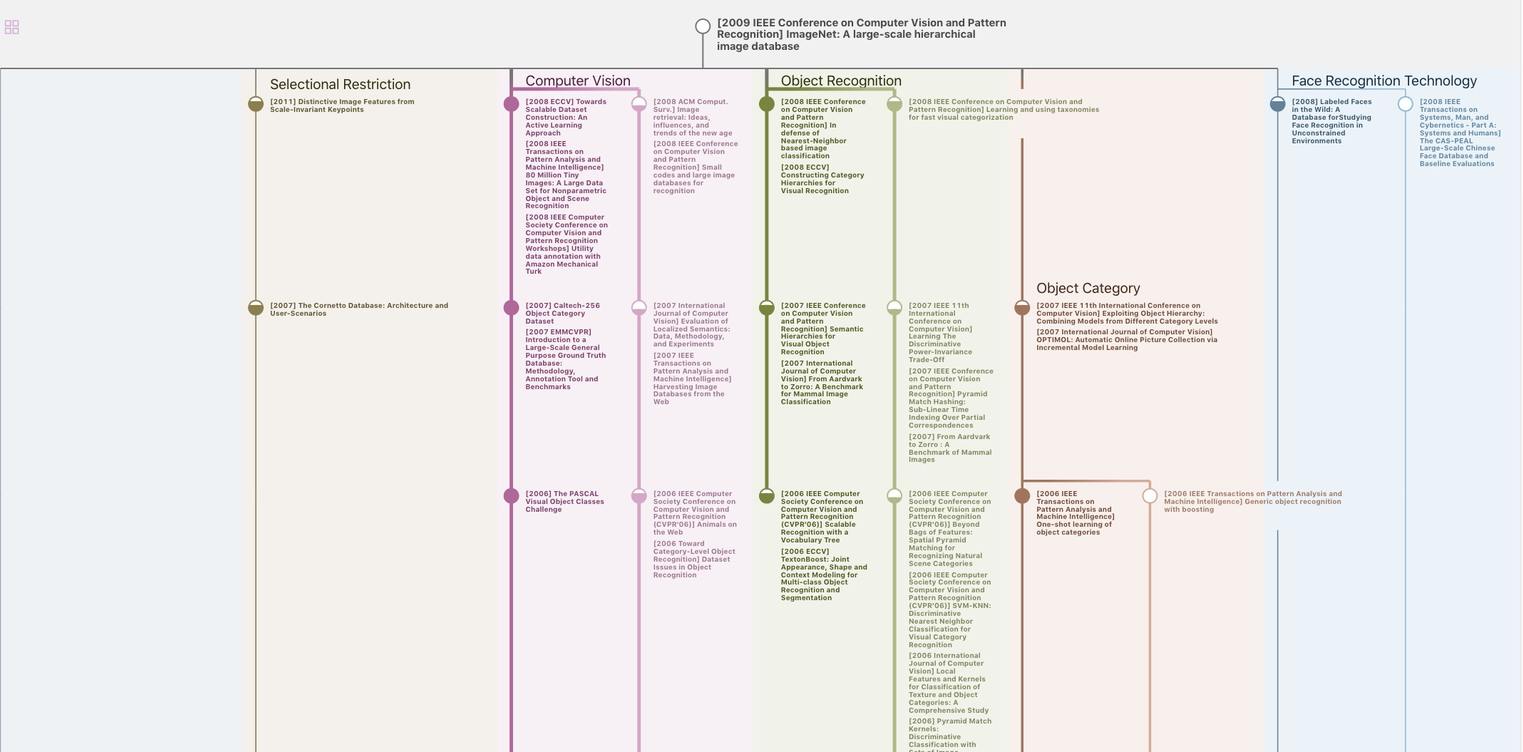
生成溯源树,研究论文发展脉络
Chat Paper
正在生成论文摘要