An efficient Bayesian approach to joint functional principal component analysis for complex sampling designs
arXiv (Cornell University)(2023)
摘要
The analysis of multivariate functional curves has the potential to yield important scientific discoveries in domains such as healthcare, medicine, economics and social sciences. However it is common for real-world settings to present data that are both sparse and irregularly sampled, and this introduces important challenges for the current functional data methodology. Here we propose a Bayesian hierarchical framework for multivariate functional principal component analysis which accommodates the intricacies of such sampling designs by flexibly pooling information across subjects and correlated curves. Our model represents common latent dynamics via shared functional principal component scores, thereby effectively borrowing strength across curves while circumventing the computationally challenging task of estimating covariance matrices. These scores also provide a parsimonious representation of the major modes of joint variation of the curves, and constitute interpretable scalar summaries that can be employed in follow-up analyses. We perform inference using a variational message passing algorithm which combines efficiency, modularity and approximate posterior density estimation, enabling the joint analysis of large datasets with parameter uncertainty quantification. We conduct detailed simulations to assess the effectiveness of our approach in sharing information under complex sampling designs. We also exploit it to estimate the molecular disease courses of individual patients with SARS-CoV-2 infection and characterise patient heterogeneity in recovery outcomes; this study reveals key coordinated dynamics across the immune, inflammatory and metabolic systems, which are associated with survival and long-COVID symptoms up to one year post disease onset. Our approach is implemented in the R package bayesFPCA.
更多查看译文
关键词
complex sampling designs,joint functional principal component
AI 理解论文
溯源树
样例
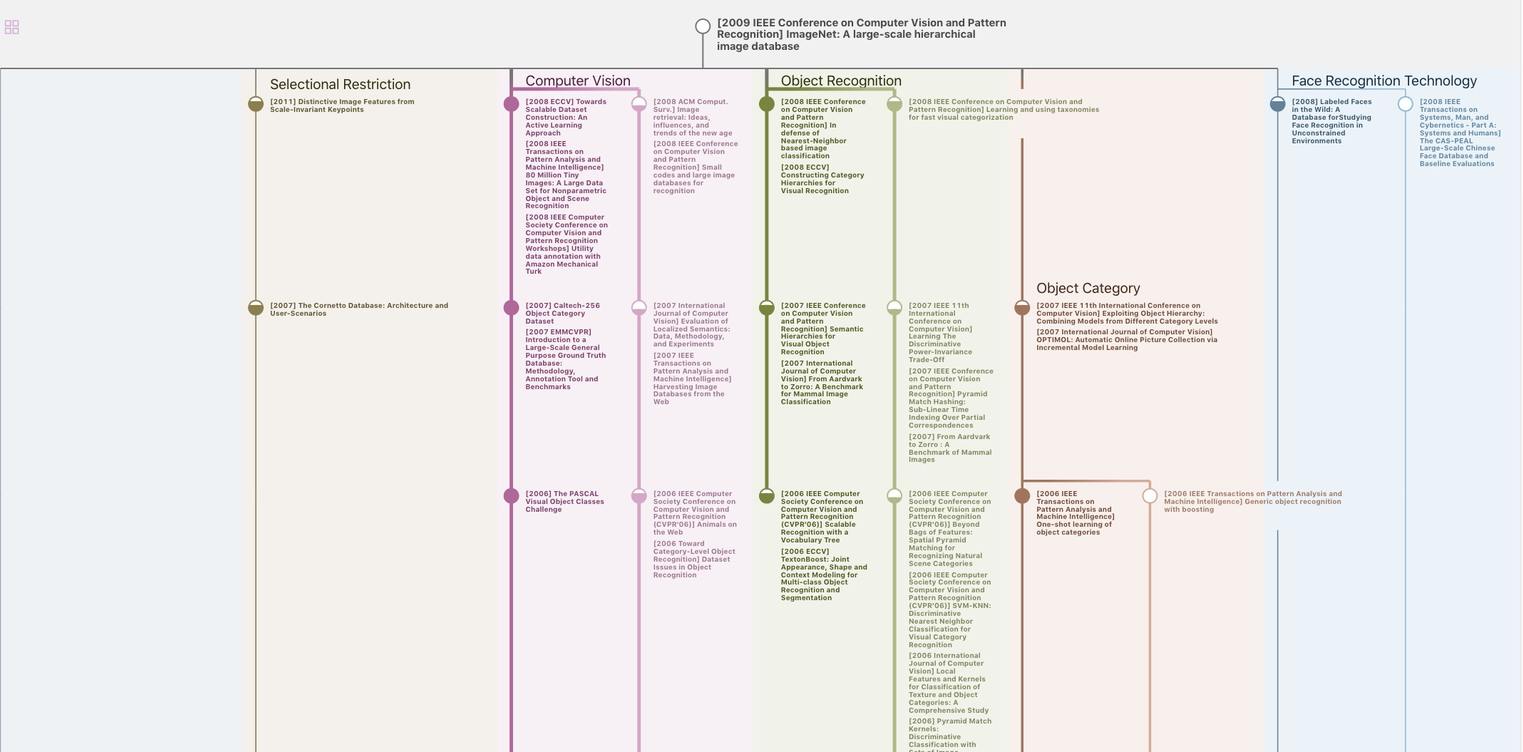
生成溯源树,研究论文发展脉络
Chat Paper
正在生成论文摘要