FireMatch: A semi-supervised video fire detection network based on consistency and distribution alignment
EXPERT SYSTEMS WITH APPLICATIONS(2024)
摘要
Deep learning techniques have greatly enhanced the performance of fire detection in videos. However, videobased fire detection models heavily rely on labeled data, and the process of data labeling is particularly costly and time-consuming, especially when dealing with videos. Considering the limited quantity of labeled video data, we propose a semi -supervised fire detection model called FireMatch, which is based on consistency regularization and adversarial distribution alignment. Specifically, we first combine consistency regularization with pseudo -label. For unlabeled data, we design video data augmentation to obtain corresponding weakly augmented and strongly augmented samples. The proposed model predicts weakly augmented samples and retains pseudo -label above a threshold, while training on strongly augmented samples to predict these pseudolabels for learning more robust feature representations. Secondly, we generate video cross -set augmented samples by adversarial distribution alignment to expand the training data and alleviate the decline in classification performance caused by insufficient labeled data. Finally, we introduce a fairness loss to help the model produce diverse predictions for input samples, thereby addressing the issue of high confidence with the non -fire class in fire classification scenarios. The FireMatch achieved an accuracy of 76.92% and 91.80% on two real -world fire datasets, respectively. The experimental results demonstrate that the proposed method outperforms the current state-of-the-art semi -supervised classification methods.
更多查看译文
关键词
Fire detection,Semi-supervised learning,Consistency regularization,Adversarial distribution alignment
AI 理解论文
溯源树
样例
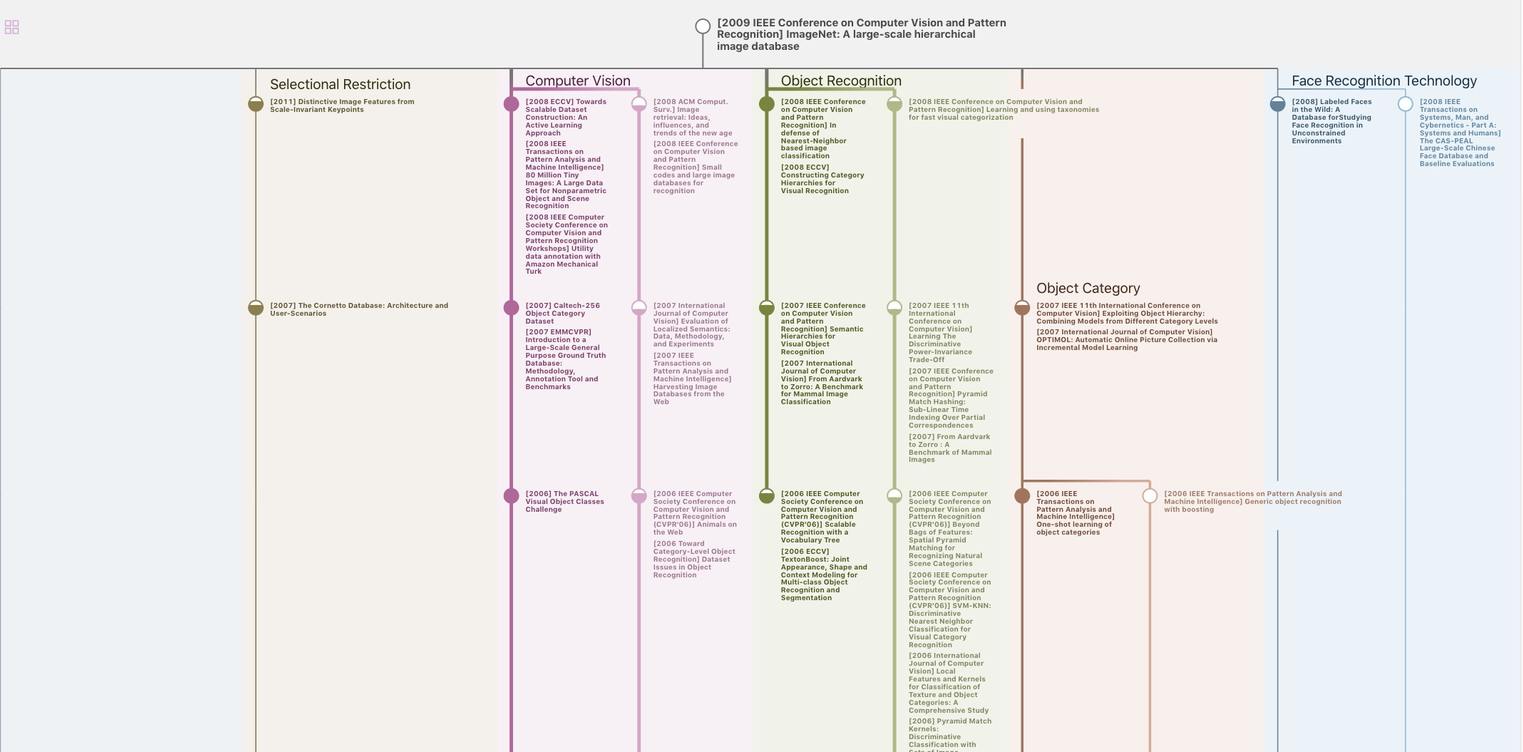
生成溯源树,研究论文发展脉络
Chat Paper
正在生成论文摘要