Unsupervised Translation Quality Estimation Exploiting Synthetic Data and Pre-trained Multilingual Encoder.
CoRR(2023)
摘要
Translation quality estimation (TQE) is the task of predicting translation quality without reference translations. Due to the enormous cost of creating training data for TQE, only a few translation directions can benefit from supervised training. To address this issue, unsupervised TQE methods have been studied. In this paper, we extensively investigate the usefulness of synthetic TQE data and pre-trained multilingual encoders in unsupervised sentence-level TQE, both of which have been proven effective in the supervised training scenarios. Our experiment on WMT20 and WMT21 datasets revealed that this approach can outperform other unsupervised TQE methods on high- and low-resource translation directions in predicting post-editing effort and human evaluation score, and some zero-resource translation directions in predicting post-editing effort.
更多查看译文
关键词
translation,quality,pre-trained
AI 理解论文
溯源树
样例
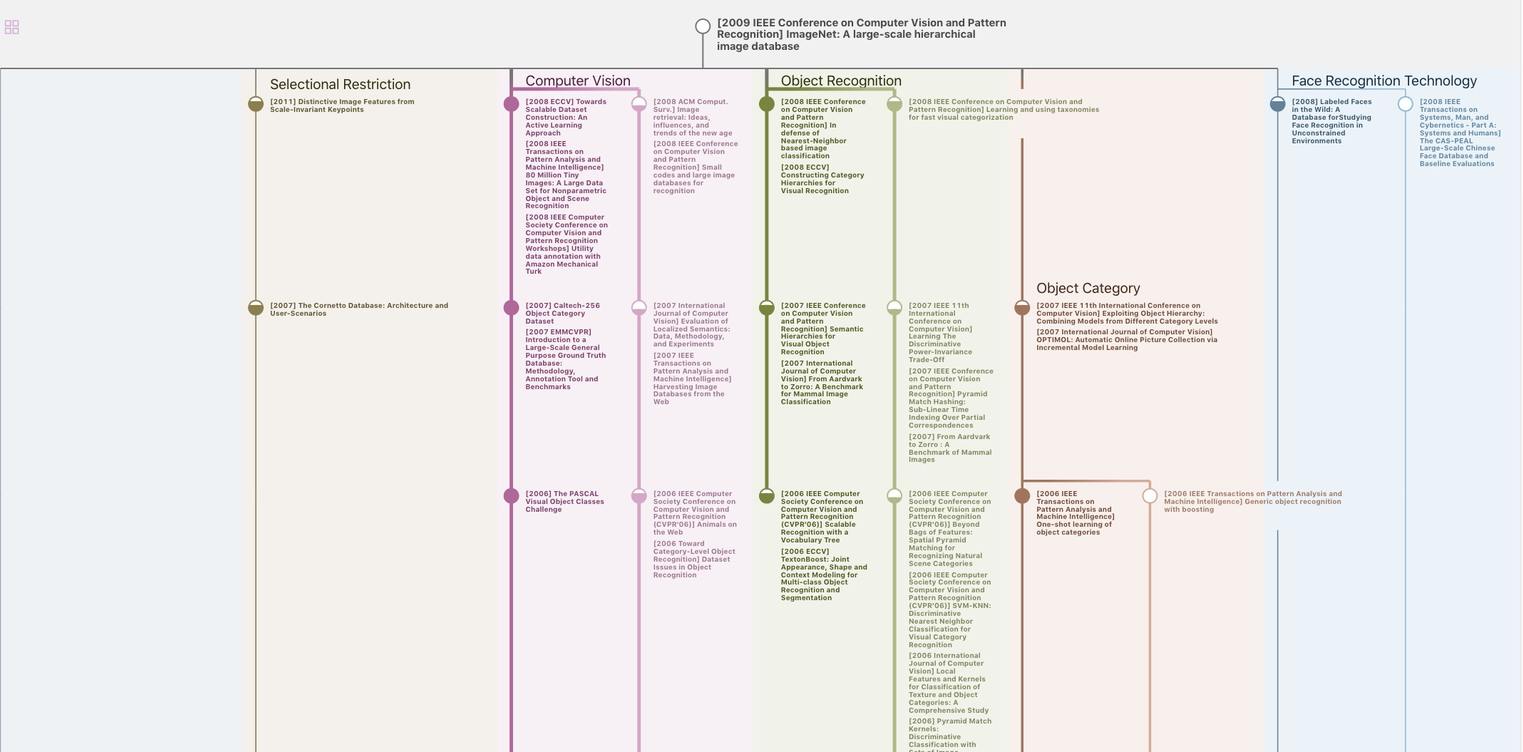
生成溯源树,研究论文发展脉络
Chat Paper
正在生成论文摘要