Sorting Out Quantum Monte Carlo.
CoRR(2023)
摘要
Molecular modeling at the quantum level requires choosing a parameterization of the wavefunction that both respects the required particle symmetries, and is scalable to systems of many particles. For the simulation of fermions, valid parameterizations must be antisymmetric with respect to the exchange of particles. Typically, antisymmetry is enforced by leveraging the anti-symmetry of determinants with respect to the exchange of matrix rows, but this involves computing a full determinant each time the wavefunction is evaluated. Instead, we introduce a new antisymmetrization layer derived from sorting, the $\textit{sortlet}$, which scales as $O(N \log N)$ with regards to the number of particles -- in contrast to $O(N^3)$ for the determinant. We show numerically that applying this anti-symmeterization layer on top of an attention based neural-network backbone yields a flexible wavefunction parameterization capable of reaching chemical accuracy when approximating the ground state of first-row atoms and small molecules.
更多查看译文
关键词
monte carlo,quantum
AI 理解论文
溯源树
样例
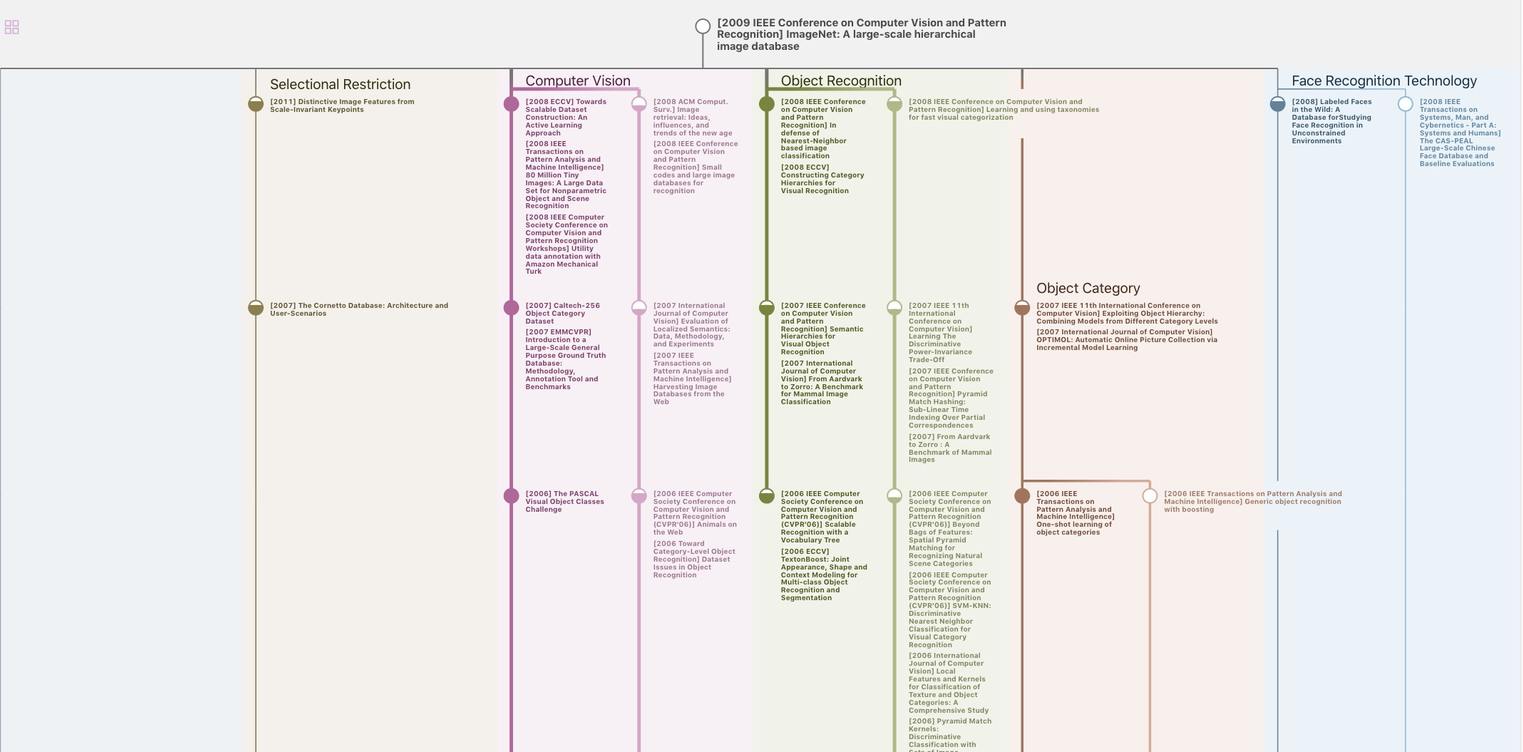
生成溯源树,研究论文发展脉络
Chat Paper
正在生成论文摘要