Amalga: Designable Protein Backbone Generation with Folding and Inverse Folding Guidance
bioRxiv (Cold Spring Harbor Laboratory)(2023)
摘要
Recent advances in deep learning enable new approaches to protein design through inverse folding and backbone generation. However, backbone generators may produce structures that inverse folding struggles to identify sequences for, indicating designability issues. We propose Amalga, an inference-time technique that enhances designability of backbone generators. Amalga leverages folding and inverse folding models to guide backbone generation towards more designable conformations by incorporating "folded-from-inverse-folded" (FIF) structures. To generate FIF structures, possible sequences are predicted from step-wise predictions in the reverse diffusion and further folded into new backbones. Being intrinsically designable, the FIF structures guide the generated backbones to a more designable distribution. Experiments on both de novo design and motif-scaffolding demonstrate improved designability and diversity with Amalga on RFdiffusion.
### Competing Interest Statement
The authors have declared no competing interest.
更多查看译文
关键词
designable protein backbone generation,folding
AI 理解论文
溯源树
样例
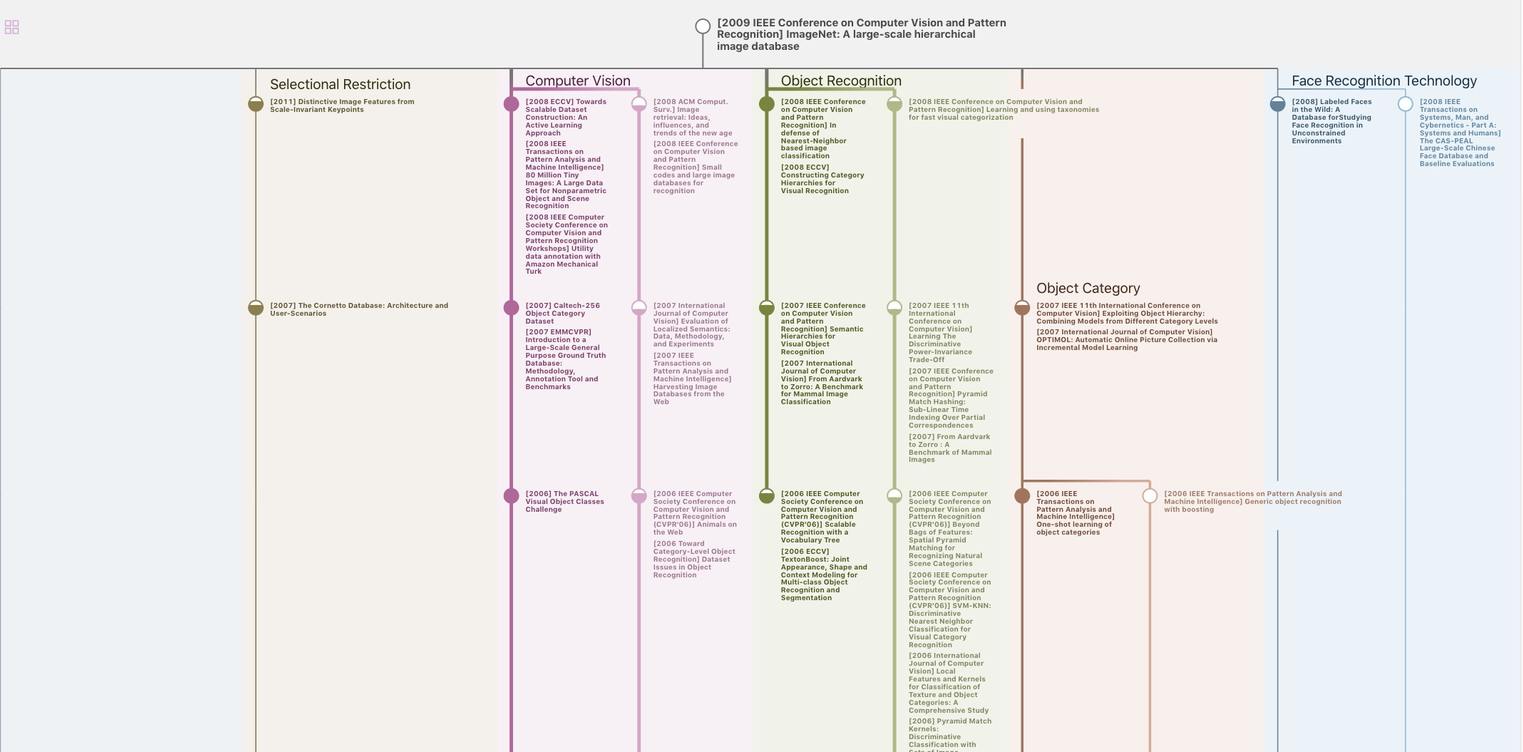
生成溯源树,研究论文发展脉络
Chat Paper
正在生成论文摘要