From Cloze to Comprehension: Retrofitting Pre-trained Masked Language Models to Pre-trained Machine Reader.
NeurIPS(2023)
摘要
We present Pre-trained Machine Reader (PMR), a novel method for retrofitting pre-trained masked language models (MLMs) to pre-trained machine reading comprehension (MRC) models without acquiring labeled data.
PMR can resolve the discrepancy between model pre-training and downstream fine-tuning of existing MLMs.
To build the proposed PMR, we constructed a large volume of general-purpose and high-quality MRC-style training data by using Wikipedia hyperlinks and designed a Wiki Anchor Extraction task to guide the MRC-style pre-training.
Apart from its simplicity, PMR effectively solves extraction tasks, such as Extractive Question Answering and Named Entity Recognition. PMR shows tremendous improvements over existing approaches, especially in low-resource scenarios.
When applied to the sequence classification task in the MRC formulation, PMR enables the extraction of high-quality rationales to explain the classification process, thereby providing greater prediction explainability. PMR also has the potential to serve as a unified model for tackling various extraction and classification tasks in the MRC formulation.
更多查看译文
关键词
masked language
AI 理解论文
溯源树
样例
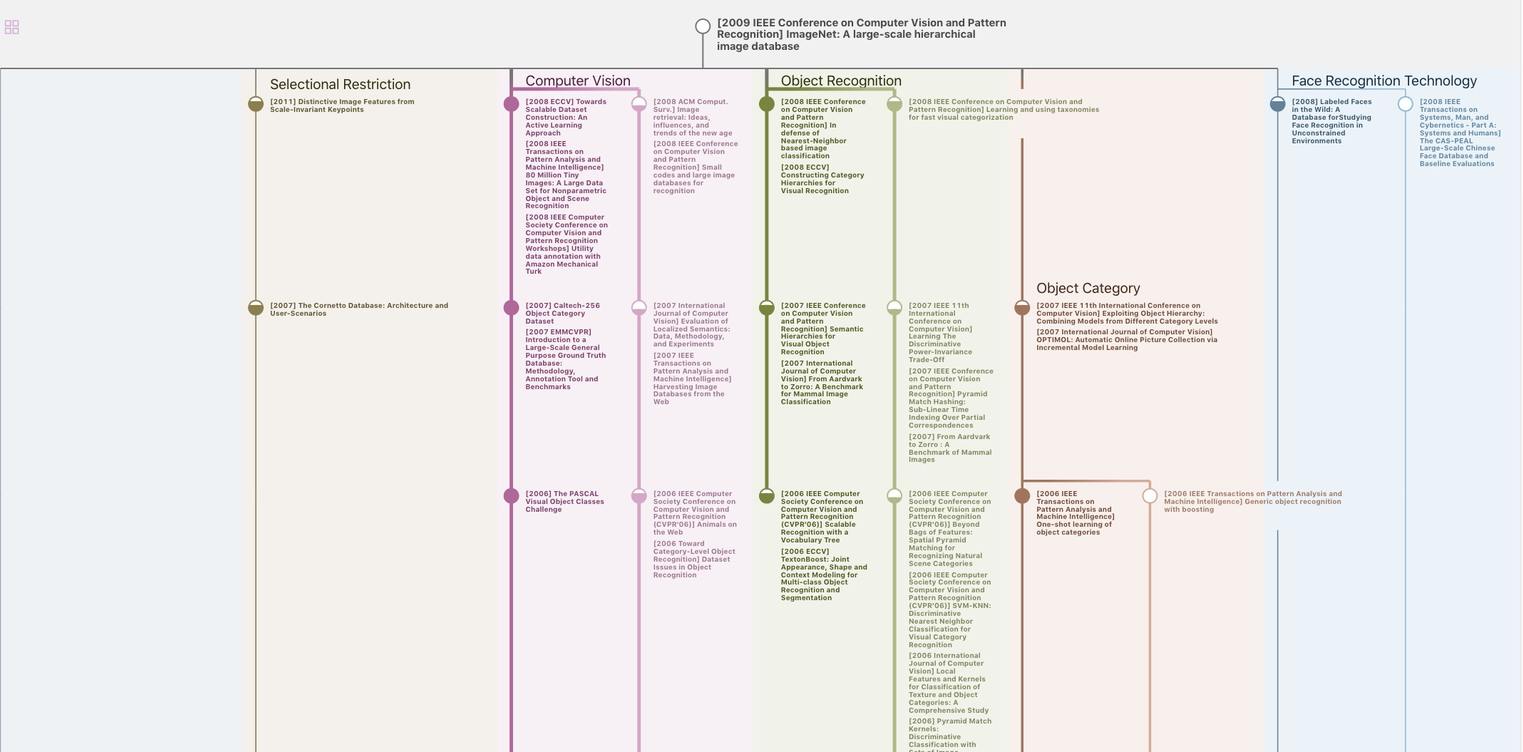
生成溯源树,研究论文发展脉络
Chat Paper
正在生成论文摘要