Designing Robust Transformers using Robust Kernel Density Estimation.
NeurIPS(2023)
摘要
Transformer-based architectures have recently exhibited remarkable successes across different domains beyond just powering large language models. However, existing approaches typically focus on predictive accuracy and computational cost, largely ignoring certain other practical issues such as robustness to contaminated samples. In this paper, by re-interpreting the self-attention mechanism as a non-parametric kernel density estimator, we adapt classical robust kernel density estimation methods to develop novel classes of transformers that are resistant to adversarial attacks and data contamination. We first propose methods that down-weight outliers in RKHS when computing the self-attention operations. We empirically show that these methods produce improved performance over existing state-of-the-art methods, particularly on image data under adversarial attacks. Then we leverage the median-of-means principle to obtain another efficient approach that results in noticeably enhanced performance and robustness on language modeling and time series classification tasks. Our methods can be combined with existing transformers to augment their robust properties, thus promising to impact a wide variety of applications.
更多查看译文
关键词
robust kernel density estimation
AI 理解论文
溯源树
样例
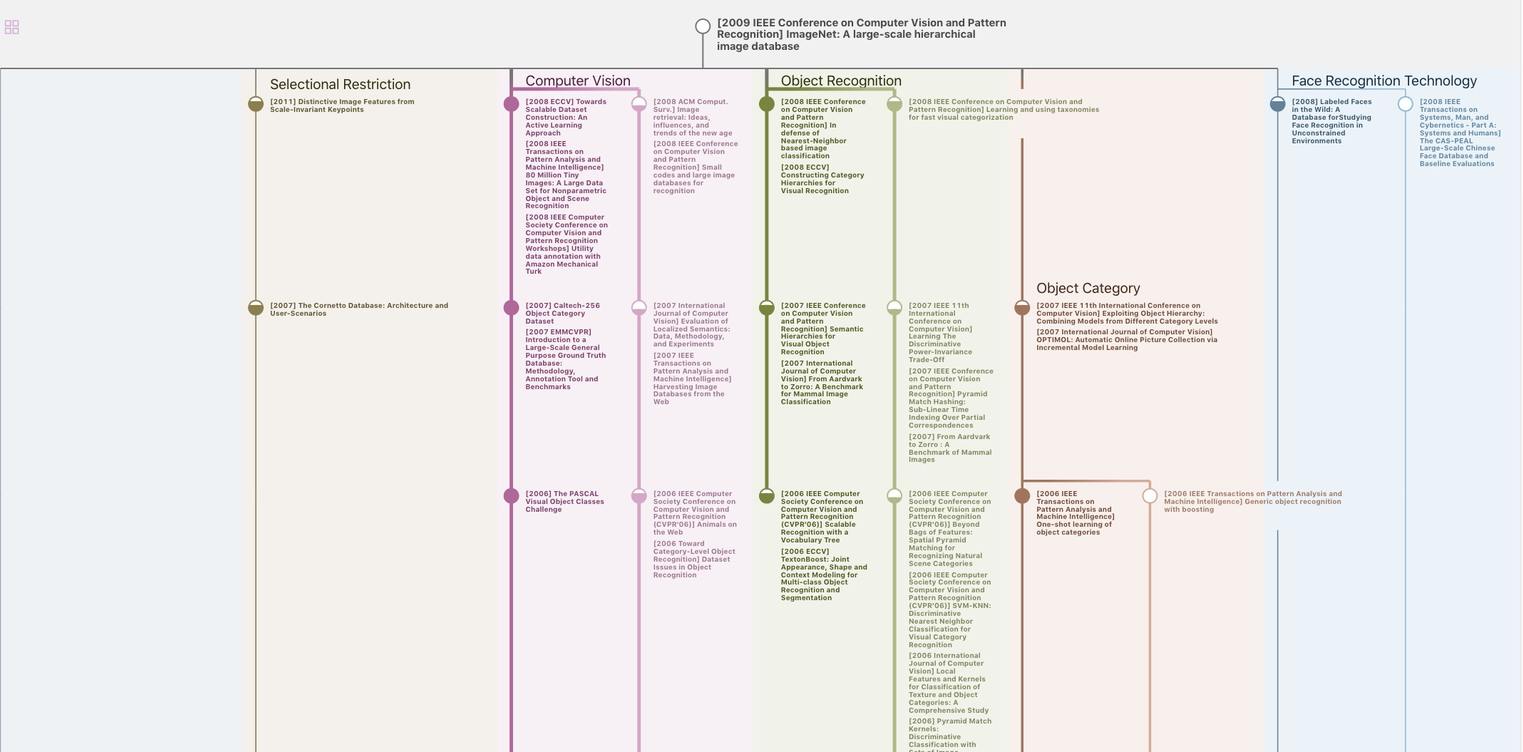
生成溯源树,研究论文发展脉络
Chat Paper
正在生成论文摘要