Scaling Laws for Hyperparameter Optimization.
NeurIPS(2023)
摘要
Hyperparameter optimization is an important subfield of machine learning that focuses on tuning the hyperparameters of a chosen algorithm to achieve peak performance. Recently, there has been a stream of methods that tackle the issue of hyperparameter optimization, however, most of the methods do not exploit the dominant power law nature of learning curves for Bayesian optimization. In this work, we propose Deep Power Laws (DPL), an ensemble of neural network models conditioned to yield predictions that follow a power-law scaling pattern. Our method dynamically decides which configurations to pause and train incrementally by making use of gray-box evaluations. We compare our method against 7 state-of-the-art competitors on 3 benchmarks related to tabular, image, and NLP datasets covering 59 diverse tasks. Our method achieves the best results across all benchmarks by obtaining the best any-time results compared to all competitors.
更多查看译文
关键词
optimization,power
AI 理解论文
溯源树
样例
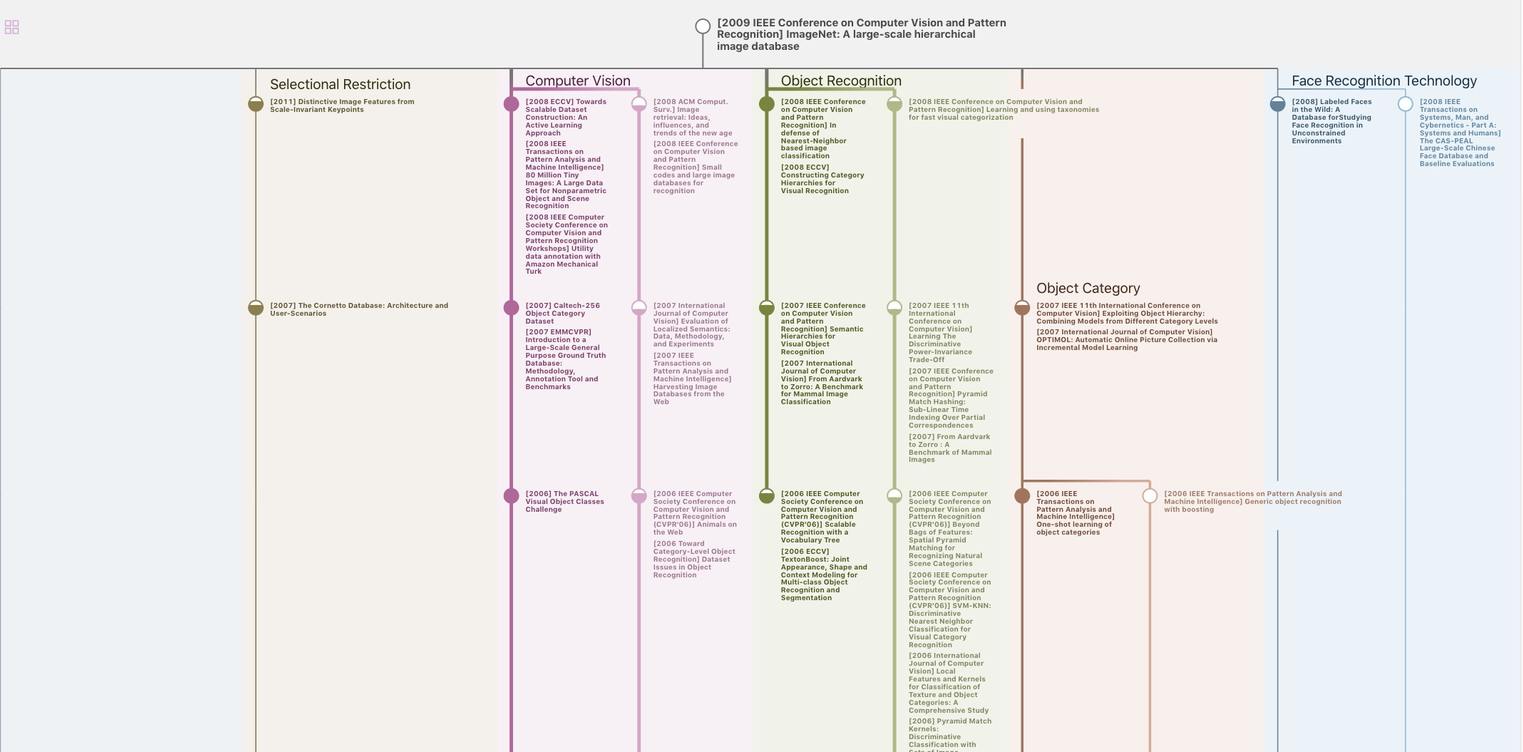
生成溯源树,研究论文发展脉络
Chat Paper
正在生成论文摘要