Comprehensive Review of Benefits from the Use of Neuron Connection Pruning Techniques During the Training Process of Artificial Neural Networks in Reinforcement Learning: Experimental Simulations in Atari Games
2023 7th International Symposium on Multidisciplinary Studies and Innovative Technologies (ISMSIT)(2023)
摘要
This paper presents a comprehensive examination of the advantages associated with the application of neuron connection pruning techniques in the training of Artificial Neural Networks (ANNs) within the context of Reinforcement Learning (RL). Our study involved rigorous experimentation through numerous Atari game simulations, with a focus on recording crucial metrics, including reward accumulation per episode, actions performed per episode, and computation time per action. The key highlight of our research is the compelling discovery that increasing the likelihood of disconnecting connections between neurons yields a significant enhancement in RL model performance. This remarkable performance boost can be attributed to the unique characteristics of RL and ANNs in RL tasks. In RL, each model processes input layers containing vast vectors of information encompassing all attributes of the current state. Our findings provide valuable insights into optimizing ANNs for RL tasks and demonstrate the practical benefits of connection pruning techniques in enhancing the performance of RL models. These results shows that when no pruning of connections is applied, and ANNs train as fully connected, the simulations are left unsolved. However, when ANNs with some pruning are employed, the tasks are successfully solved. Furthermore, our findings reveal a noteworthy side effect: increasing the dropout rate of train connections can significantly reduce processing time. This outcome holds significant implications for optimizing RL models, as it not only enhances performance but also streamlines computational efficiency.
更多查看译文
关键词
Reinforcement Learning,Artificial Neural Networks,Neuron Connection Pruning Techniques
AI 理解论文
溯源树
样例
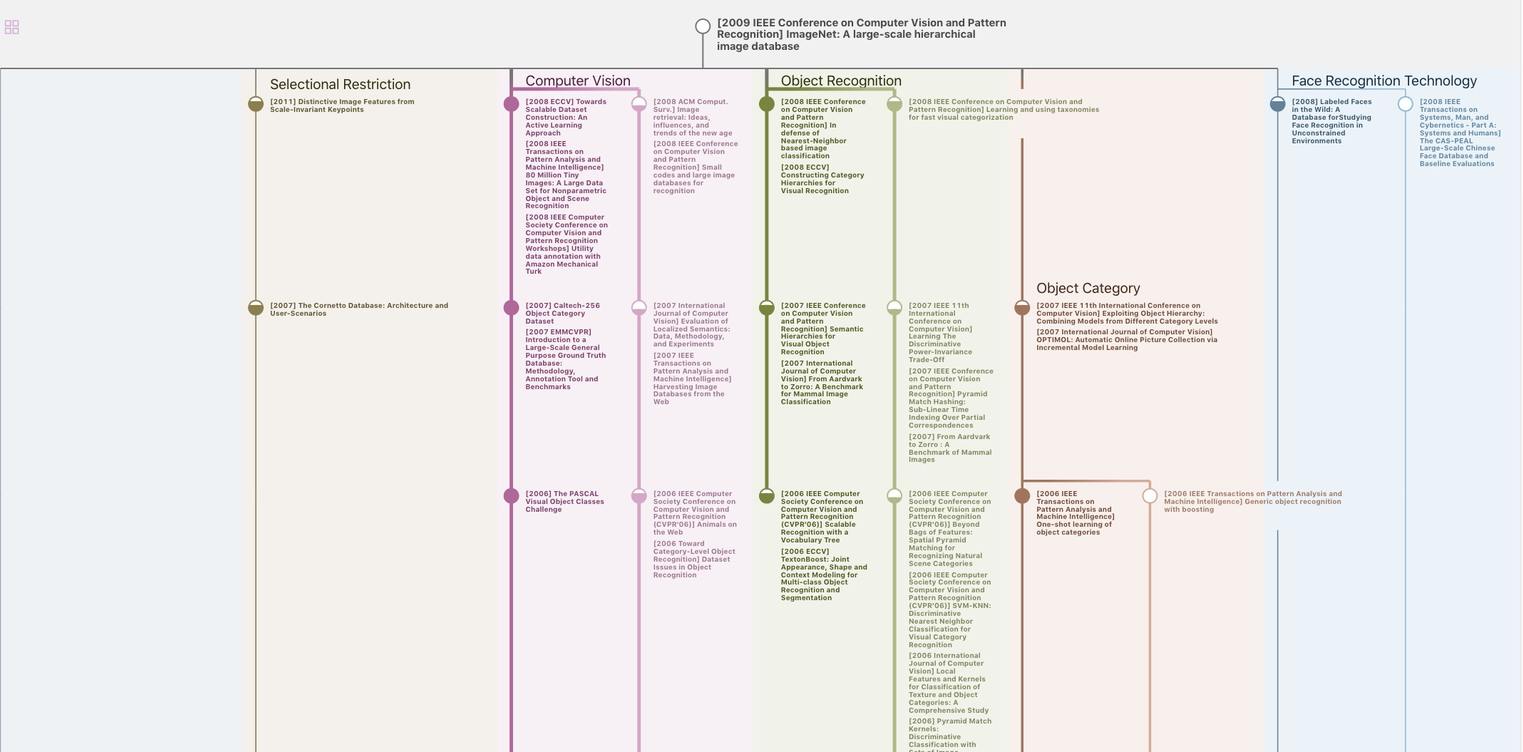
生成溯源树,研究论文发展脉络
Chat Paper
正在生成论文摘要