RegAgg: A Scalable Approach for Efficient Weight Aggregation in Federated Lesion Segmentation of Brain MRIs
2023 EIGHTH INTERNATIONAL CONFERENCE ON FOG AND MOBILE EDGE COMPUTING, FMEC(2023)
摘要
Federated learning (FL), a distributed machine learning paradigm, enables multiple clients to train models locally on their data while only sharing model updates with the central server. However, this process can be computationally intensive and resource-consuming, leading to communication overheads and scalability issues. In this study, we investigate the robustness and reliability of Regularized Weight Aggregation (RegAgg) and compare its performance with an additional regularization layer while aggregating model weights for the federated segmentation of cancer tumors in brain MRIs. Our method is evaluated using a large set of brain image data which consists of multi-institutional multi-parametric magnetic resonance imaging (mpMRI) scans. Through extensive experimentation, we demonstrate that the performance of federated lesion segmentation is significantly improved with RegAgg, surpassing the results obtained when an additional regularization process is introduced. The findings highlight the efficacy of RegAgg as a powerful strategy for advancing brain tumor segmentation in FL settings. Notably, RegAgg provides scalability, cost-effectiveness, and the ability to adapt to collaborators with heterogeneous non-IID characteristics. It enables efficient resource allocation and collaborator selection, contributing to enhanced performance in dynamic brain tumor segmentation. Overall, this research contributes to the development of efficient weight aggregation techniques for dynamic brain tumor segmentation in FL. The proposed approach demonstrates its potential to address the challenges associated with collaborative training on distributed brain MRI data while maintaining accuracy and privacy.
更多查看译文
关键词
Brain Tumors,Cancer,Collaborative Learning,Federated Learning,Federated Lesion Segmentation,Regularized Weight Aggregation
AI 理解论文
溯源树
样例
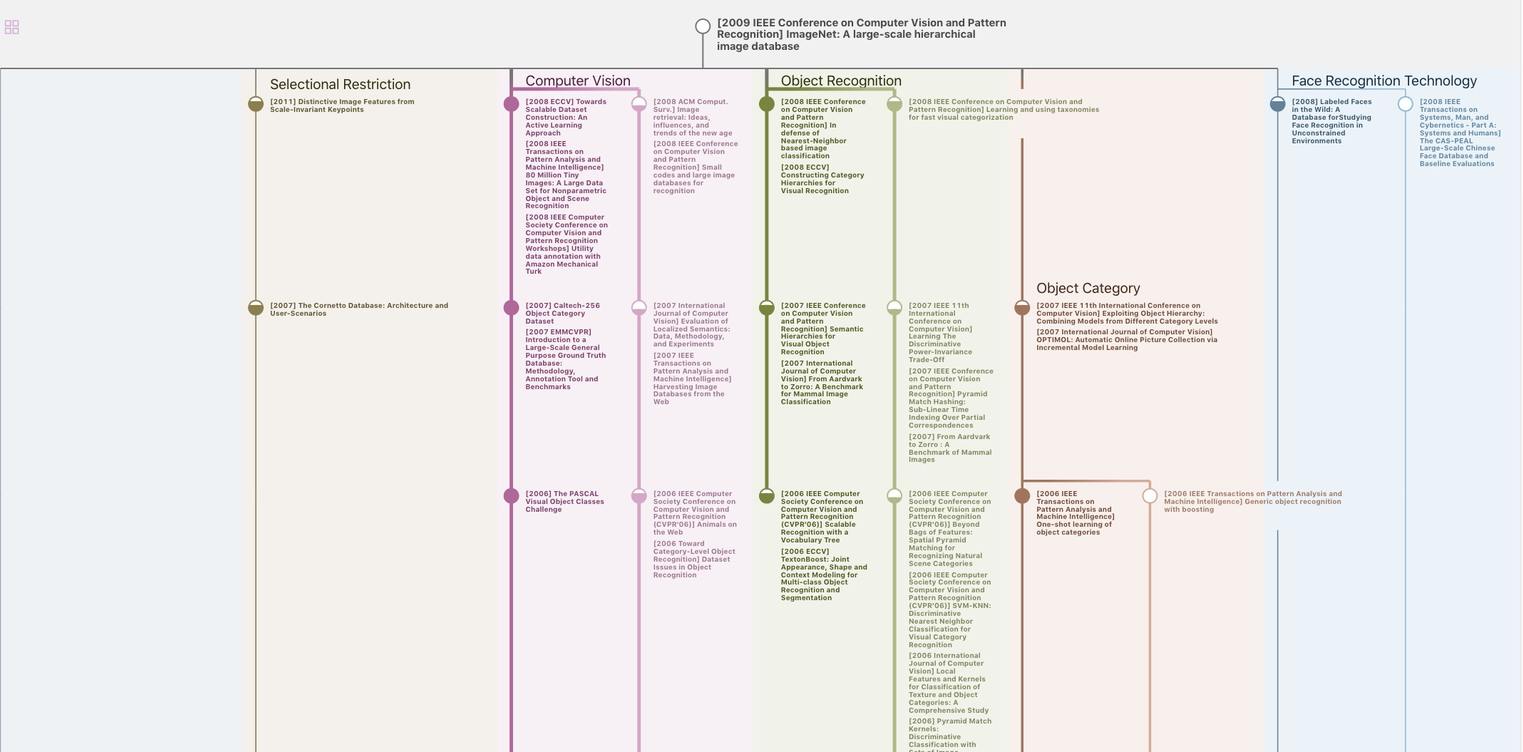
生成溯源树,研究论文发展脉络
Chat Paper
正在生成论文摘要