Molecular Graph-Based Deep Learning Algorithm Facilitates an Imaging-Based Strategy for Rapid Discovery of Small Molecules Modulating Biomolecular Condensates
Journal of medicinal chemistry(2023)
摘要
Biomolecular condensates are proposed to cause diseases, such as cancer and neurodegeneration, by concentrating proteins at abnormal subcellular loci. Imaging-based compound screens have been used to identify small molecules that reverse or promote biomolecular condensates. However, limitations of conventional imaging-based methods restrict the screening scale. Here, we used a graph convolutional network (GCN)-based computational approach and identified small molecule candidates that reduce the nuclear liquid-liquid phase separation of TAR DNA-binding protein 43 (TDP-43), an essential protein that undergoes phase transition in neurodegenerative diseases. We demonstrated that the GCN-based deep learning algorithm is suitable for spatial information extraction from the molecular graph. Thus, this is a promising method to identify small molecule candidates with novel scaffolds. Furthermore, we validated that these candidates do not affect the normal splicing function of TDP-43. Taken together, a combination of an imaging-based screen and a GCN-based deep learning method dramatically improves the speed and accuracy of the compound screen for biomolecular condensates.
更多查看译文
关键词
molecules,deep learning algorithm facilitates,molecular,deep learning algorithm,graph-based,imaging-based
AI 理解论文
溯源树
样例
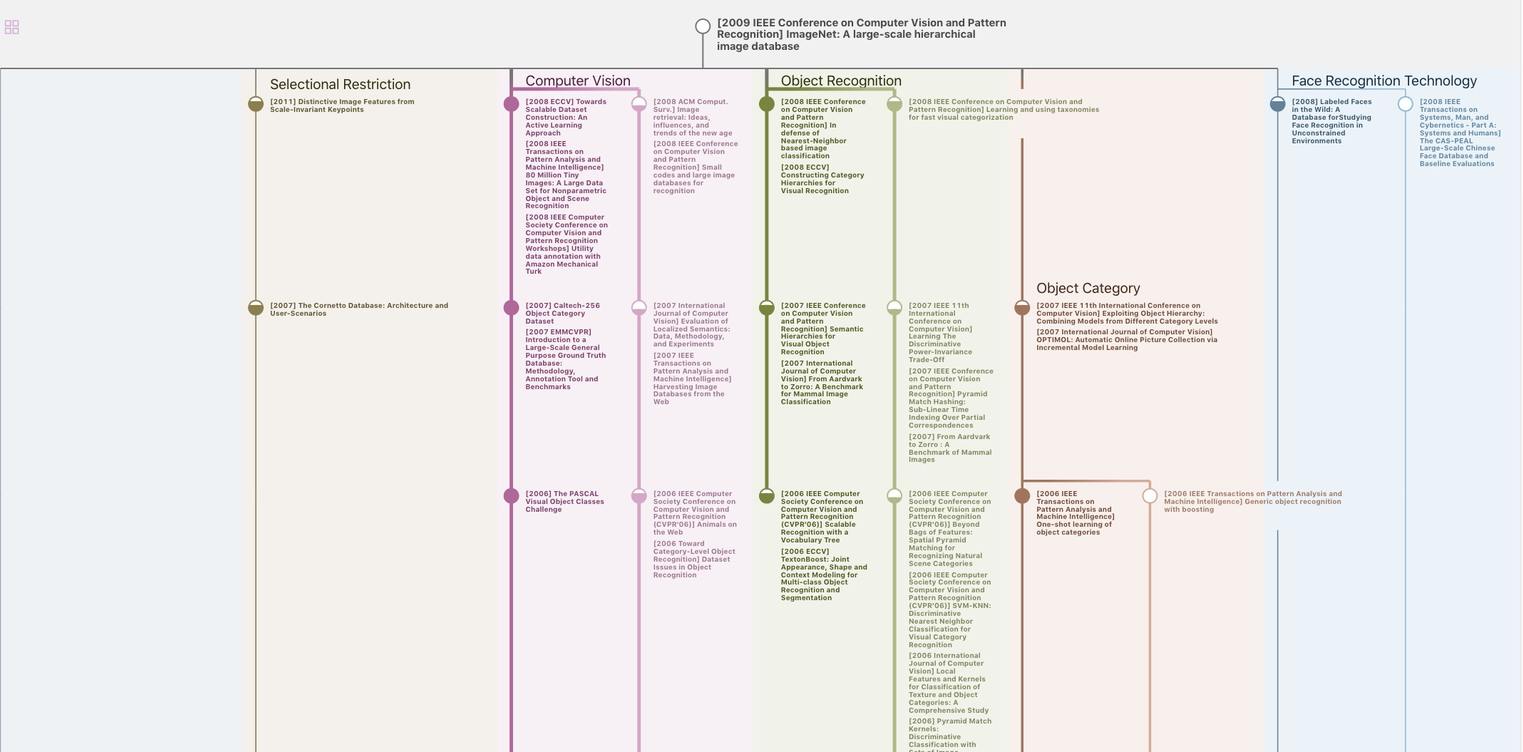
生成溯源树,研究论文发展脉络
Chat Paper
正在生成论文摘要