Improving transportability of randomized controlled trial inference using robust predictionmethods
Statistical methods in medical research(2023)
摘要
Randomized trials have been the gold standard for assessing causal effects since their introduction by Fisher in the 1920s, since they can eliminate both observed and unobserved confounding. Estimates of causal effects at the population level from randomized controlled trials can still be biased if there are both effect modification and systematic differences between the trial sample and the ultimate population of inference with respect to these modifiers. Recent advances in the survey statistics literature to improve inference in nonprobability samples by using information from probability samples can provide an avenue for improving population causal inference in randomized controlled trials when relevant probability samples of the patient population are available. We review some recent work in "transporting" causal effect estimates from trials to populations, focusing on the setting where there is a "benchmark" or population-representative sample along with the RCT sample. We then propose estimators using either inverse probability weighting (IPWT) or prediction that can accommodate unequal probability of selection in the "benchmark" or population, and use Bayesian additive regression trees for both inverse probability of treatment weighting and prediction estimation that do not require specification of functional form or interaction. We also consider how the assumption of ignorability may be assessed from observed data and propose a sensitivity analysis under the failure of this assumption. We compare our proposed approach with existing methods in simulation and apply these alternative approaches to a study of pulmonary artery catheterization in critically ill patients. We also suggest next steps for future work.
更多查看译文
关键词
robust predictionmethods,transportability,randomized controlled trial,trial inference
AI 理解论文
溯源树
样例
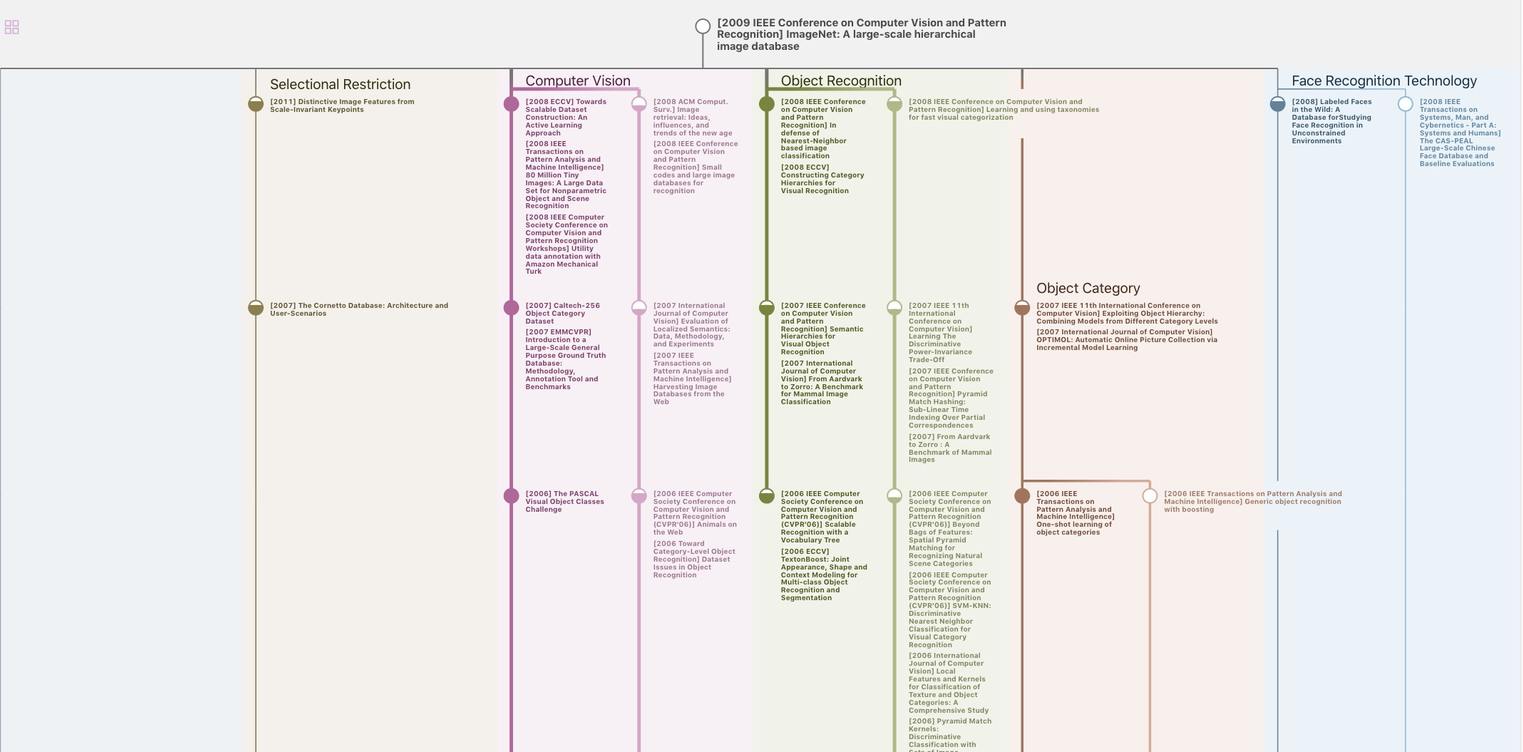
生成溯源树,研究论文发展脉络
Chat Paper
正在生成论文摘要