Long-term Time Series Forecasting based on Decomposition and Neural Ordinary Differential Equations.
CoRR(2023)
摘要
Long-term time series forecasting (LTSF) is a challenging task that has been
investigated in various domains such as finance investment, health care,
traffic, and weather forecasting. In recent years, Linear-based LTSF models
showed better performance, pointing out the problem of Transformer-based
approaches causing temporal information loss. However, Linear-based approach
has also limitations that the model is too simple to comprehensively exploit
the characteristics of the dataset. To solve these limitations, we propose
LTSF-DNODE, which applies a model based on linear ordinary differential
equations (ODEs) and a time series decomposition method according to data
statistical characteristics. We show that LTSF-DNODE outperforms the baselines
on various real-world datasets. In addition, for each dataset, we explore the
impacts of regularization in the neural ordinary differential equation (NODE)
framework.
更多查看译文
关键词
long-term time series forecasting,time series decomposition,instance normalization,neural ordinary differential equations
AI 理解论文
溯源树
样例
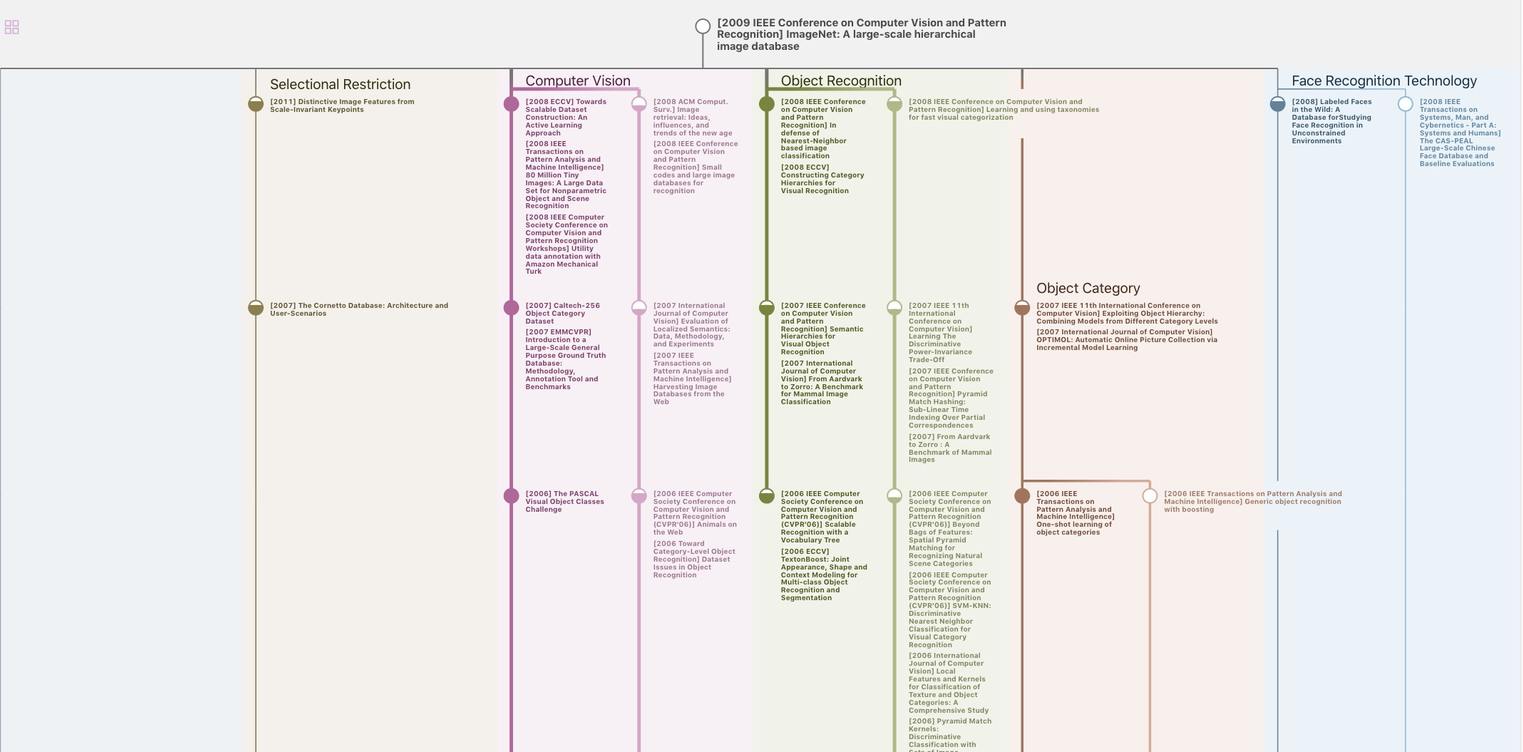
生成溯源树,研究论文发展脉络
Chat Paper
正在生成论文摘要