Parameter Tuning in the Radial Kernel-Based Partition of Unity Method by Bayesian Optimization.
CoRR(2023)
摘要
In this paper, we employ Bayesian optimization to concurrently explore the optimal values for both the shape parameter and the radius in the partition of unity interpolation using radial basis functions. Bayesian optimization is a probabilistic, iterative approach that models the error function through a progressively self-updated Gaussian process. Meanwhile, the partition of unity approach harnesses a meshfree method, allowing us to significantly reduce computational expenses, particularly when considering a substantial number of scattered data points. This reduction in computational cost is achieved by decomposing the entire domain into several smaller subdomains, each of them with a variable radius. We provide an estimation of the complexity of our algorithm and carry out numerical experiments to illustrate the effectiveness of our approach, dealing with test and real-world datasets.
更多查看译文
关键词
unity method,optimization,partition,kernel-based
AI 理解论文
溯源树
样例
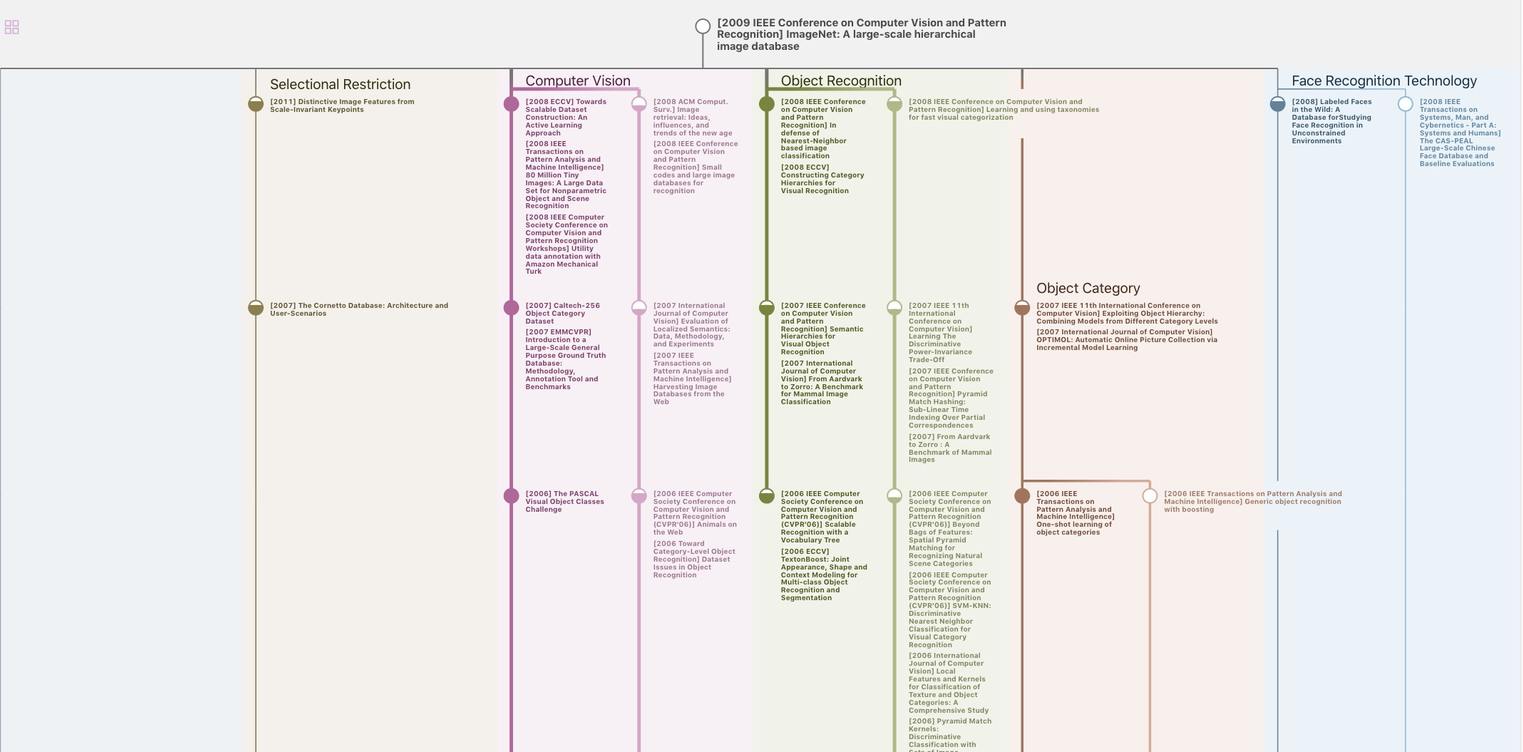
生成溯源树,研究论文发展脉络
Chat Paper
正在生成论文摘要