Knowledge distillation-driven semi-supervised multi-view classification
INFORMATION FUSION(2024)
摘要
Semi-supervised multi-view classification is a critical research topic that leverages the discrepancy between different views and limited annotated samples for pattern recognition in computer vision. However, it encounters a significant challenge: obtaining comprehensive discriminative representations with a scarcity of labeled samples. Although existing methods aim to learn discriminative features by fusing multi-view information, a significant challenge persists due to the difficulty of transferring complementary information and fusing multiple views with limited supervised information. In response to this challenge, this work introduces an innovative algorithm that integrates Self-Knowledge Distillation (Self-KD) to facilitate semi-supervised multi -view classification. Initially, we employ a view-specific feature extractor for each view to learn discriminative representations. Subsequently, we introduce a self-distillation module to drive information interaction across multiple views, enabling mutual learning and refinement of multi-view unified and specific representations. Moreover, we introduce a class-aware contrastive module to alleviate confirmation bias stemming from noise in the generated pseudo-labels during knowledge distillation. To the best of our knowledge, this is the first attempt to extend Self-KD to address semi-supervised multi-view classification problems. Extensive experimental results validate the efficiency of this approach in semi-supervised multi-view classification compared to existing state-of-the-art methods.
更多查看译文
关键词
Multi-view learning,Semi-supervised classification,Self-knowledge distillation,Contrastive learning
AI 理解论文
溯源树
样例
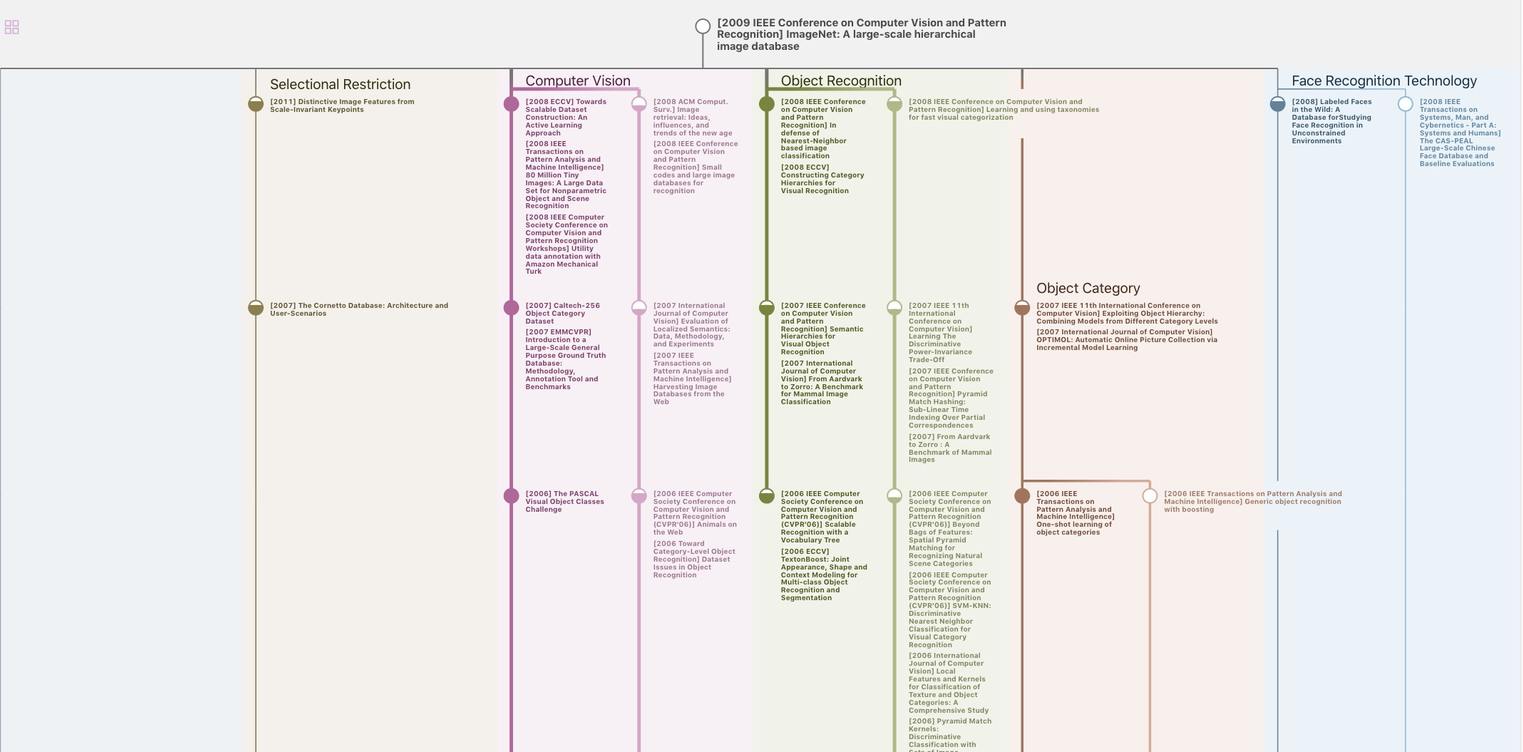
生成溯源树,研究论文发展脉络
Chat Paper
正在生成论文摘要