ConPep: Prediction of peptide contact maps with pre-trained biological language model and multi-view feature extracting strategy
COMPUTERS IN BIOLOGY AND MEDICINE(2023)
摘要
The accurate prediction of peptide contact maps remains a challenging task due to the difficulty in obtaining the interactive information between residues on short sequences. To address this challenge, we propose ConPep, a deep learning framework designed for predicting the contact map of peptides based on sequences only. To sufficiently incorporate the sequential semantic information between residues in peptide sequences, we use a pretrained biological language model and transfer prior knowledge from large scale databases. Additionally, to extract and integrate sequential local information and residue-based global correlations, our model incorporates Bidirectional Gated Recurrent Unit and attention mechanisms. They can obtain multi-view features and thus enhance the accuracy and robustness of our prediction. Comparative results on independent tests demonstrate that our proposed method significantly outperforms state-of-the-art methods even with short peptides. Notably, our method exhibits superior performance at the sequence level, suggesting the robust ability of our model compared with the multiple sequence alignment (MSA) analysis-based methods. We expect it can be meaningful research for facilitating the wide use of our method.
更多查看译文
关键词
Peptide contact map prediction,Pre-trained language model,Multi-view feature fusion
AI 理解论文
溯源树
样例
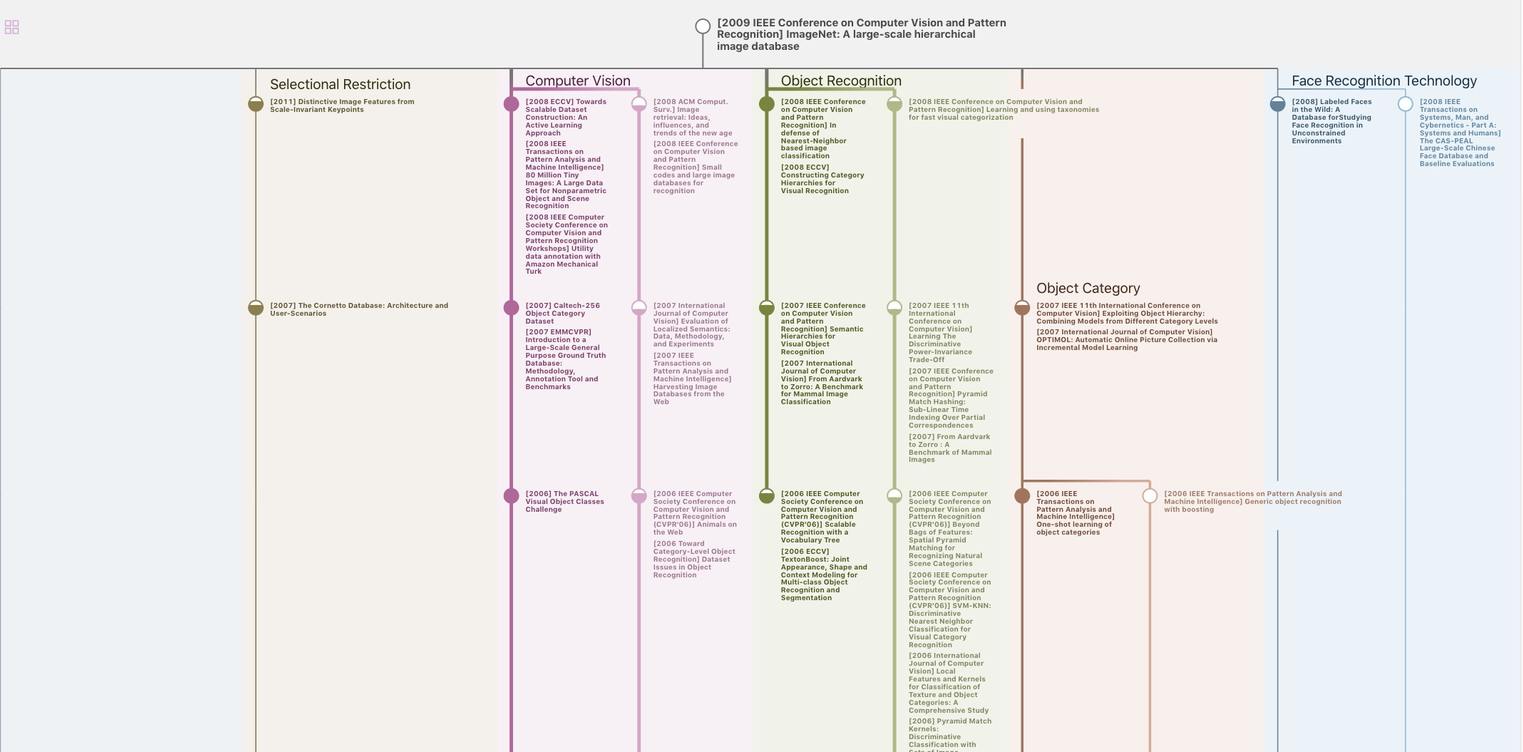
生成溯源树,研究论文发展脉络
Chat Paper
正在生成论文摘要