Learning to compensate for lack of information: Extracting latent knowledge for effective temporal knowledge graph completion
INFORMATION SCIENCES(2024)
摘要
The goal of temporal knowledge graph embedding (TKGE) is to represent the entities and relations in a given temporal knowledge graph (TKG) as low-dimensional vectors (i.e., embeddings), which preserve both semantic information and temporal dynamics of the factual information. In this paper, we posit that the intrinsic difficulty of existing TKGE methods lies in the lack information in KG snapshots with timestamps, each of which contains the facts that co-occur at specific timestamp. To address this challenge, we propose a novel self-supervised TKGE approach, THOR (Three-tower grapH cOnvolution netwoRks (GCNs)), which extracts latent knowledge from TKGs by jointly leveraging both temporal and atemporal dependencies between entities and the structural dependency between relations. THOR learns the embeddings of entities and relations, obtained from three-tower GCNs by (1) maximizing the likelihood of the facts in a TKG and (2) addressing the lack of information in a TKG based on the auxiliary supervision signals each entity. Our experiments on three real-world datasets demonstrate that THOR significantly outperforms 17 competitors in terms of TKG completion tasks. THOR yields up to 9.37% higher accuracy than the best competitor.
更多查看译文
关键词
Temporal knowledge graph,Graph embedding,Graph convolutional networks,Self-supervised learning
AI 理解论文
溯源树
样例
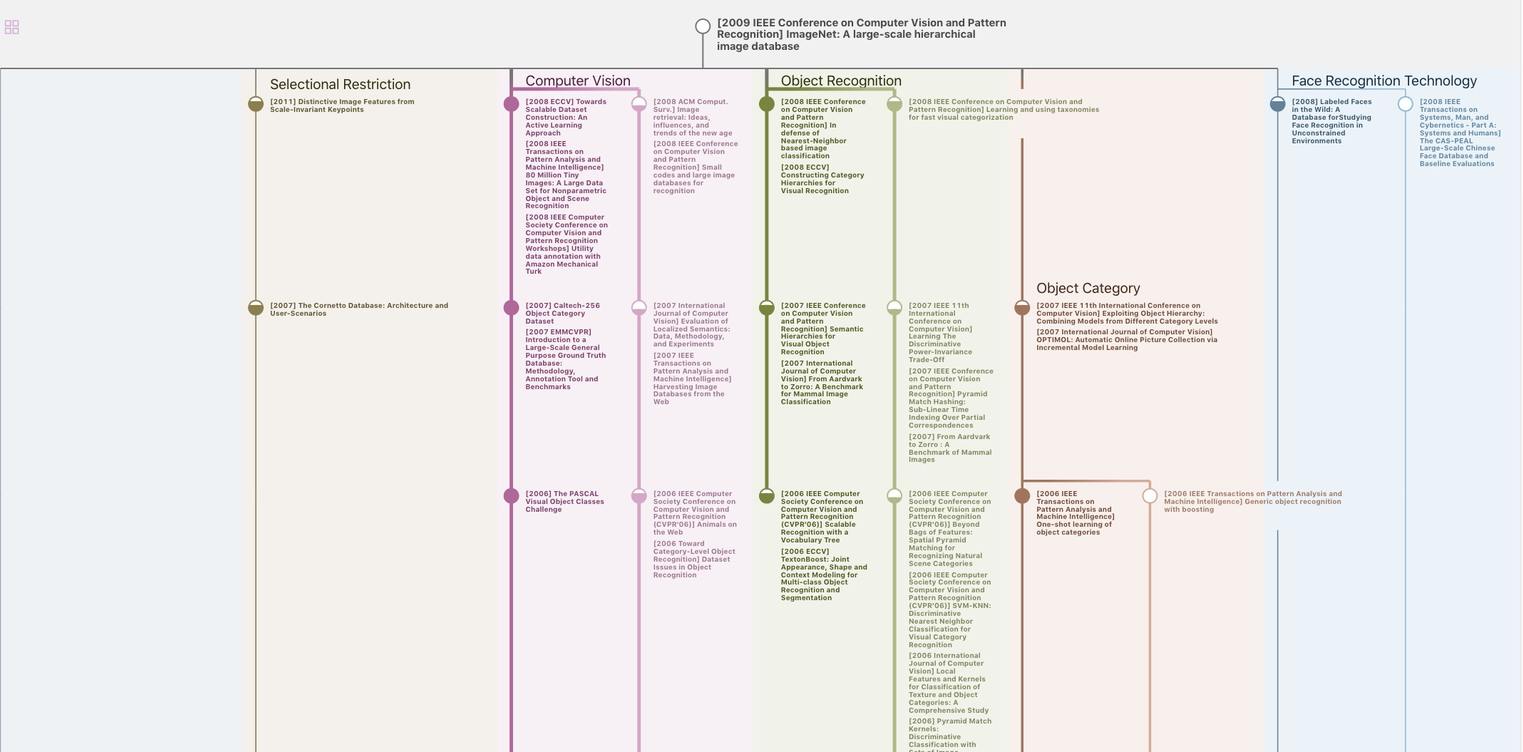
生成溯源树,研究论文发展脉络
Chat Paper
正在生成论文摘要